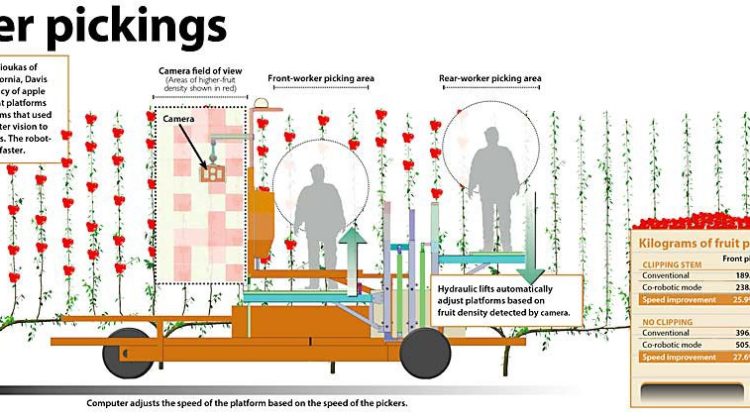
Zumo Labs presents The Juice, a weekly newsletter focused on computer vision problems (and sometimes just regular problems). Get it while it’s fresh.

____
What is a sandwich? Maybe you’ve heard this one before, but try to define a sandwich. Odds are good that one of your more annoying friends would be able to suggest something that, yes, upon closer examination, is technically a sandwich. (“What about open-faced sandwiches? Hoagies? Hot dogs? A folded slice of pizza?”) The point of the prompt is to show that, as humans, we have trouble with this sort of classification exercise.
But it’s not so much that we’re bad at classification — we seem to demonstrate a good intuitive sense of what’s what — as it is the sloppy mess of labels for the things in our everyday life. And if messy labels can trip us up, you can imagine what they do to a computer vision model. We advocate for synthetic data a lot and, while it’s not a silver bullet, it does resolve a lot of the issues of the industry standard that it’s intended to supplement (or in some cases, replace): sourced labeled data. It’s a topic we covered in our most recent blog post, Five Big Problems With Labeled Data.
Gosh, how would you even begin to train a computer vision model to detect a sandwich? No idea, but if you’ll excuse us, we made ourselves hungry.
____
Researchers from Northeastern University have published a study on the flattening effect of labels within datasets where race is concerned. “In a paper, they argue that labels are unreliable as indicators of identity because some labels are more consistently defined than others and because datasets appear to ‘systematically’ encode stereotypes of racial categories.”
Researchers find that labels in computer vision datasets poorly capture racial diversity, via VentureBeat.
As a nice coda to last week’s newsletter on Flickr and MegaFace, researchers Inioluwa Deborah Raji and Genevieve Fried have published a historical survey of over 100 face datasets constructed between 1976 and 2019. They found that as the demand for labeled facial data increased, researchers gradually abandoned asking for people’s consent. From their paper’s conclusion: “Dataset evaluation is a critical juncture at which we can provide transparency and even accountability over facial recognition systems, and interrogate the ethics of a given dataset towards producing more responsible machine learning development.”
This is how we lost control of our faces, via MIT Technology Review.
Gesture Technology Partners, a company founded by Timothy Pryor, sued Apple last week, claiming that its Face ID and other camera features (including facial recognition and smart HDR) infringe on its patents. Apple had previously secured several patents from Pryor, including one related to their multitouch technology.
Lawsuit alleges Apple biometrics infringe patents, via Biometric Update.
The danger of deepfakes is widely acknowledged (even if they now seem like an over engineered way to convince people to believe crazy things). That’s why many tech giants have endeavored to create deepfake detectors in the first place. But a team out of UC San Diego has recently shown that, by inserting adversarial examples of the face and inserting them into the frame, even the most advanced deepfake detectors in the wild can be fooled.
Scientists prove that deepfake detectors can be duped, via Engadget.
We’re super bullish on the potential that computer vision holds for agtech, so we’re not surprised to see several news items in the space this week. First, the USDA’s Natural Resources Conservation Service awarded a $1.5 million grant to NC State for an imaging system they’re developing that will predict herbicide-resistant weeds in cover crops. Meanwhile, researchers at UC Davis share results from a 2019 trial of a cobot — a collaborative robot — that uses computer vision to automatically raise and lower a worker-assist platform based on where the most apples hang.
Alternative Weed Control — We’ll Have An App For That, via NC State University.
Collaborative robotics tested in tree fruit, via Good Fruit Grower.
“I’m not a cat.”
Kitten Zoom Filter Mishap, via YouTube.
____
Synthetic Data and Hierarchical Object Detection in Overhead Imagery
Using a GAN to style transfer the appearance of real images onto synthetic images is a key component of the synthetic data creation toolkit. This paper proposes a variation they call the “GAN-Reskinner”, where a GAN generates the texture that is applied to a 3D model (rather than being applied to the final image itself). They demonstrate the effectiveness of their new approach on a satellite image detection task.
____
Think The Juice was worth the squeeze? Sign up here to receive The Juice weekly.