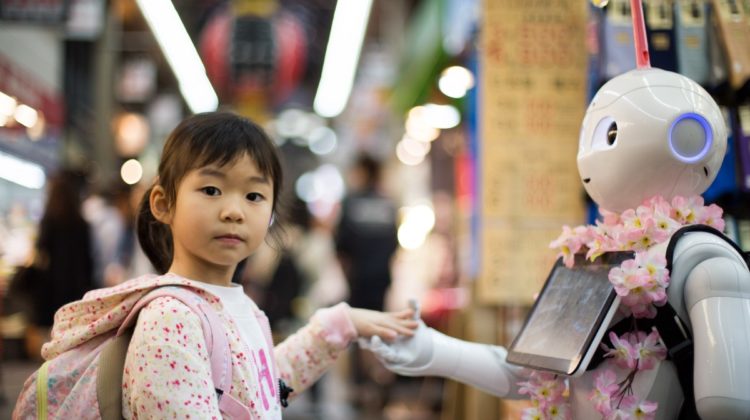
If there is something the graph shows you, it is the fact that there is a need for a large amount of data for a machine learning/deep learning project’s progress. For a good machine learning project, a company needs to have access to clean data. To do this, companies should make sure they have access to data, data that has relevance to their business and is free of inaccuracy, and available.
As part of the ultimate objective of an automated relationship, the data must be clean and organized, and easily accessible for both short tasks and large scale training. With this simple access, knowledge can be readily made available for both short tasks and large scale training.
Data scientists cause companies to add models that are not built as legacy-resilient structures. The choice on whether to substitute with current legacy systems is focused on the result of data science models designed to promote creativity without taking into consideration that these legacy systems are not modernized. While the solutions were designed to be run in the market, once they were integrated with the whole system, there are very few successes in terms of ease of adoption.
The only way to improve this technology is to bring both teams that will be working on the machine-learning project and the one that manages the legacy system together. After this two-step deployment plan, a milestone-wise deployment of the project is recommended to aid easy adoption with a friction-less migration.
Companies have a shortage of data scientists in the market who solve the data problem. Although there are many computer science engineers taking up ML courses and labeling themselves as ‘data scientists’, the ones with the skills that are truly skilled to see through a complex ML project are extremely limited in number. A report released by the State of Enterprise Machine Learning project states that the demand for Machine Learning experts is rising, but there is a severe scarcity of people delivering the skills and training to serve as these roles.
As the number of updates to the machine learning project grows, the machine learning project tends to become outdated and fail to comply with the original purpose. This could be caused because of:
- The business situation has changed.
- Current demand for these will shift.
- Over time, better models come into the market.
The reason it is difficult to modify and update an existing machine learning model is because it is like building a new project when it is declined to do so. It is easy to notice that organizations tend to continue to use the models that worked in the past when they no longer remain available or useful.
Sometimes a leader might lack the expertise and determination to accomplish a task in a machine learning project. The project will be beneficial to society because it backs the fame that it gets. However, it will lack the necessary support, personnel requirements, accuracy, and accessibility to many people.
For a machine learning project to be effective, it is very important to keep everyone on board particularly the board members — because even a hint of doubt in them may lead to a lot of insecurity among the teams, something that guarantees project failure even before it sets off the ground.
So here are some major considerations that still prevent machine learning from reaching levels that businesses and industries need to survive and stay competitive.
There are several solutions to these problems and one of them is by working with a smart solution company that can help you deploy a new-generation technology into your firm. Integration of machine learning allows professionals to select a decision process that achieves the goal of the client as well as the client’s advantages.
Reference
See my other stories !!