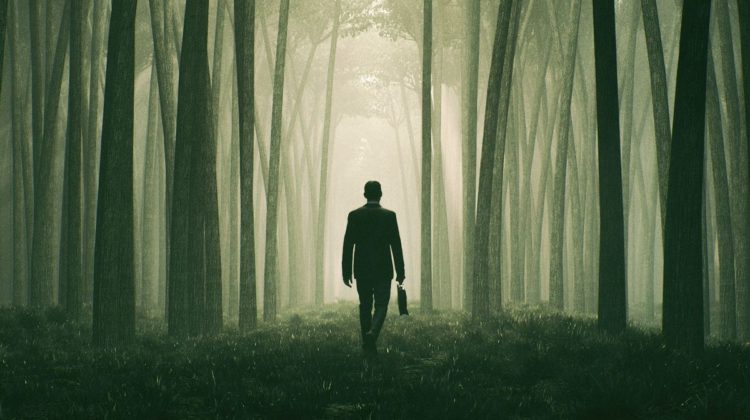
When building models with elements of randomness we should always validate our performance (really we should do this regardless, but hear me out). We want to know whether our model will work well with new/unseen data. Fortunately, the bootstrapping procedure will leave us with a subset of observations that weren’t used to train the model. This is typically around 25% of the data and mimics the train/test split commonly found in data science. The official term for this unused data is “out-of-boot” data and we can apply the above aggregate prediction technique to assess how well our Random forest performs. Bootstrapping to aggregate prediction modal estimates based on out-of-boot data is unfortunately termed “bagging”.
Well done. You now know the fundamental mechanics of a Random forest. There are optimisation parameters, such as the number of randomly selected features to assess at each node that we can adjust, but I think we’ve covered enough for this weeks article.
I want to leave you, as always, with some real life examples of methods described today.
The future of medicine is decision augmentation using novel machine learning algorithms in parallel with expert clinical experience.
Prostate cancer is one of the most common cancers on the planet (even though it only affects men). Despite, or indeed, because of its commonality most men die with prostate cancer, not from it. Those who die from prostate cancer often have very aggressive disease by definition. Presently, our best test for screening men for prostate cancer is measuring a blood marker called PSA. PSA is incredibly sensitive, but lacks in specificity. This means it can be raised for many reasons other than cancer and if used for screening it will lead to many men being referred for prostatic biopsy. The problem with biopsy is it’s a particularly invasive line of questioning and comes with a whole host of adverse complications. Further to this, the majority of men, who endure a prostatic biopsy, don’t go on to develop aggressive forms of prostate cancer. This paradox has plagued the urological public health departments of developed worlds since the advent of screening programs.
L. Xiao et al have used Random forests to combine clinico-demographic data with two less suitable screening investigations (serum PSA and transrectal US) to achieve a specificity of 93.8%. Incredible! This is another demonstration of the power of applying novel ML approaches to address real-world clinical problems. This model will of course need to be validated on many new test sets, but should it stay the course it will prove a useful tool for augmenting clinicians identifying high-risk individuals for prostatic biopsy.
Thank you for reading, I hope you found it useful and give it a clap if did!
Follow here for more like this and subscribe to my YouTube channel to learn more.