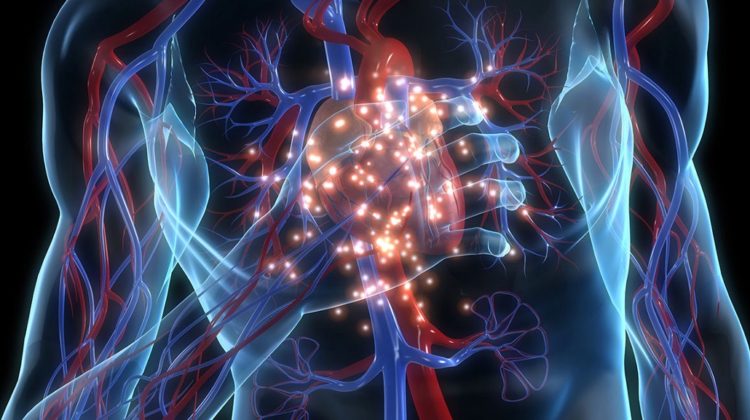

Artificial intelligence (AI) applications in cardiology have the potential to be groundbreaking, but which applications will actually help save lives and help people live longer? One major area that is being transformed is cardiac computed tomography (CT). Specifically, the use of AI to analyze coronary artery disease (CAD) and markers of CAD found on cardiac CT.
So far studies have been limited in quality and size, but there’s a growing body of data that suggests that cardiac CT machine learning (ML), including deep learning (DL), and neural network algorithms can improve the accuracy of predicting major cardiovascular events. This includes events such as major heart attacks and cardiac death. This improved accuracy can help save people’s lives long-term.
Being able to better risk stratify patients can help identify which patients need more aggressive treatment of risk factors and CAD versus which patients need the continued standard of care. In the future, it may be able to help tell if patients should be on medications and monitored for coronary inflammation and its reversal. This can help prevent the long-term progression of cardiovascular disease.
The other reason that this is important is because of the current and projected shortage of cardiologists in the United States. Primary care doctors can refer appropriate patients for cardiac CT and then based on the results recommendations are made regarding when and how frequently the patient needs to follow up with a cardiologist. The more accurate the evaluation of data from the cardiac CT is the more accurate the recommendations will be. Cardiac CT is already reimbursed by insurance companies, so cost is not relatively a major issue.
AI algorithms can be applied to the actual cardiac CT images and/or to the results from the cardiac CT images. [1] ML algorithms can be used to help predict the future risk of major cardiovascular events and mortality because they can take into account a greater number of factors than traditional methods. [2] One method is an ML algorithm created risk score that can improve the prognostic accuracy of existing cardiac CT angiography (CCTA) methods for risk scores. [3] AI can help cardiac CT be more useful for those classified to be at intermediate risk for cardiovascular events, to help reclassify them as high risk or low risk. [4] This can help better identify which patients may need more aggressive treatment and more frequent follow-up with a cardiologist.
Another method is using ML algorithms to analyze cardiac CT coronary artery calcium scores (CACS). [5, 6] Calcium scores, which reflect the amount of calcified cholesterol plaques in or on the cardiac arteries, are used for cardiac risk stratification. Cardiac CT scans that are done only for calcium scores don’t use as much radiation as calcium scores calculated from CCTA scans. ML algorithms used with CACS can be used to help better predict the likelihood of having blockages in the coronary arteries which would then be evaluated further by CCTA or cardiac catheterization. [7]
Alternatively, neural networks can be used to quantify a calcium score from a CCTA scan without first having to do a separate scan for the calcium score, this can help reduce radiation to the patient as well. [8] DL algorithms can also be used to calculate calcium scores from low dose CTs which can also help reduce radiation and save time, this method has been shown to have a good correlation with expert readers in some studies. [1]
Cardiac CT can also show if cholesterol plaques on the cardiac artery walls are calcified and stable or soft and unstable. Plaques that are soft are prone to rupture and are more likely to cause heart attacks. AI algorithms could help better visualize and quantify cholesterol plaques on the cardiac artery wall and they can help improve plaque characterization.
DL algorithms performing this process are helpful because classifying cholesterol plaques manually is time-consuming. There can also be expert reader variability. AI algorithms could also help better predict which lesions will be the culprit lesions, the ones likely to be causing a decrease in blood flow to the myocardium leading to myocardial ischemia. [9]
Fractional Flow Reserve-CT (FFR-CT) AI algorithms look at which coronary artery lesions are ischemic. [10, 11, 12, 13] This is a non-invasive technology that calculates the amount of blood flow through the coronary artery. It can more accurately predict the significance of cardiac artery cholesterol blockages than CCTA alone. [14, 15]
Another method to look at significant blockages includes using DL algorithms to segment the left ventricle myocardium for FFR-CT. [16] In addition, a newer use of AI for FFR-CT includes looking at the shear stress of blockages along the cardiac artery walls to predict future cardiovascular events. [17] This non-invasive test, FFR-CT, can help better determine which patients should undergo cardiac catheterization, an invasive procedure. [18]
Radiomics works to extract a large number of imaging features from the cardiac CT image using data characterization algorithms. Radiomics risk prediction models will likely play an important role in the future predictive value of cardiac CT. ML algorithms can help discover previously unknown patterns that can help predict cardiovascular events, a radiomic score could be an additional imaging biomarker. [19] This can add predictive value for cardiac risk to the calcium score. Alternatively, cardiac CT algorithms can also help identify people having heart attacks based on changes not visible to the human eye. [20] These are newer technologies and still need to be improved for consistent accuracy, improved spatial resolution will likely help with this issue.
Another area of interest in radiomics is the evaluation of epicardial fat and perivascular fat for the prediction of cardiovascular events. [21] A newer cholesterol plaque assessment technology, called the fat attenuation index (FAI) is an area of interest. AI algorithms can visualize and quantify coronary inflammation by evaluating the surrounding fat tissue. [22] Quantifying the amount of coronary inflammation can be predictive for future cardiovascular events and mortality. [23] Because AI algorithms can detect disease-related changes in the epicardial and perivascular fat tissue this could be another imaging biomarker for cardiovascular risk. [1, 24]
One of the major concerns with AI algorithms is bias. This is because many of the databases used in research studies are skewed for local populations. Hospitals make it difficult for research groups to share data. [5] This can make data biased for gender, and more commonly race and ethnicity because of regional differences. [25] This of course would make a difference in predictions as there are gender, race, and ethnicity differences in cardiovascular outcomes. Larger, multi-center merged cardiac imaging databases are needed to help with this issue. [1]
Another area of concern is that the prediction of risk for future cardiovascular events can also be seen negatively for the patient in regards to obtaining health insurance, disability insurance, and life insurance. Also, an area of concern is long-term risks from radiation and how to minimize radiation per patient. This is one of the reasons that patients don’t generally get yearly cardiac CTs right now to monitor the progression of cardiovascular disease.
Future opportunities include AI algorithms that can help further improve the existing accuracy of cardiac CT risk scores and calculations to predict future cardiovascular events. In addition, AI algorithms that can extract additional data or imaging biomarkers from the cardiac CT scan without additional radiation to the patient is a major area of interest. Given that cardiovascular disease is the number one cause of death in the world, there’s a wide range of opportunities for the future use of AI algorithms to improve the capabilities of cardiac CT and other cardiac imaging modalities as well.
- Artificial Intelligence in Cardiovascular Imaging: JACC State-of-the-Art Review
- Machine learning for prediction of all-cause mortality in patients with suspected coronary artery disease: a 5-year multicentre prospective registry analysis
- Maximization of the usage of coronary CTA derived plaque information using a machine learning based algorithm to improve risk stratification; insights from the CONFIRM registry
- Latest Advances in Cardiac CT
- Deep convolutional neural networks to predict cardiovascular risk from computed tomography
- Machine Learning in Predicting Coronary Heart Disease and Cardiovascular Disease Events: Results from the Multi-Ethnic Study of Atherosclerosis (MESA)
- Machine learning of clinical variables and coronary artery calcium scoring for the prediction of obstructive coronary artery disease on coronary computed tomography angiography: analysis from the CONFIRM registry
- Automatic coronary artery calcium scoring in cardiac CT angiography using paired convolutional neural networks
- A Boosted Ensemble Algorithm for Determination of Plaque Stability in High-Risk Patients on Coronary CTA
- Evaluation of Significant Coronary Artery Disease Based on CT Fractional Flow Reserve and Plaque Characteristics Using Random Forest Analysis in Machine Learning
- State-of-the-Art Deep Learning in Cardiovascular Image Analysis
- A machine-learning approach for computation of fractional flow reserve from coronary computed tomography
- Deep learning analysis of left ventricular myocardium in CT angiographic intermediate-degree coronary stenosis improves the diagnostic accuracy for identification of functionally significant stenosis
- Diagnostic Accuracy of a Machine-Learning Approach to Coronary Computed Tomographic Angiography–Based Fractional Flow Reserve
- Integrated prediction of lesion-specific ischaemia from quantitative coronary CT angiography using machine learning: a multicentre study
- Deep learning analysis of the myocardium in coronary CT angiography for identification of patients with functionally significant coronary artery stenosis
- Future Directions in Coronary CT Angiography: CT-Fractional Flow Reserve, Plaque Vulnerability, and Quantitative Plaque Assessment
- A Recurrent CNN for Automatic Detection and Classification of Coronary Artery Plaque and Stenosis in Coronary CT Angiography
- Radiomics of Coronary Artery Calcium in the Framingham Heart Study
- Texture Analysis and Machine Learning for Detecting Myocardial Infarction in Noncontrast Low-Dose Computed Tomography: Unveiling the Invisible
- Detecting human coronary inflammation by imaging perivascular fat
- A novel machine learning-derived radiotranscriptomic signature of perivascular fat improved cardiac risk prediction using coronary CT angiography
- Non-invasive detection of coronary inflammation using computed tomography and prediction of residual cardiovascular risk (the CRISP CT study): a post-hoc analysis of prospective outcome data
- Deep Learning for Quantification of Epicardial and Thoracic Adipose Tissue from Non-Contrast CT
- Artificial Intelligence, Machine Learning, and Cardiovascular Disease