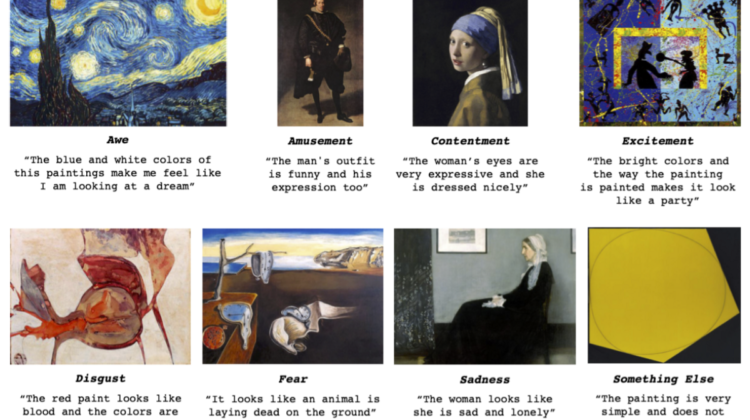
The researchers built the ArtEmis dataset on top of the visual art encyclopedia WikiArt. ArtEmis contains 81,446 curated artworks from 1,119 artists, covering 27 art styles and 45 genres from the 15th century to the present. It also includes 439,121 explanatory utterances and emotional responses related to the artworks.
The researchers trained a series of “neural speaker” models on ArtEmis. While these are not exactly art critics, they can produce plausible grounded emotion explanations after viewing artworks. The team says they speak in language that is “significantly more affective, abstract, and rich with metaphors and similes” to express “moods, feelings, personal attitudes, but also abstract concepts like freedom or love.” (Maybe they are a lot like art critics.)
The neural speakers perform emotion explanation evoked by artworks with the help of two popular language model backbone architectures: Show-Atten-Tell (SAT), an image encoder with a word/image attentive LSTM combined; and M² — a meshed Transformer model with memory for image captioning. The set of proposed neural speakers also includes a fine-tuned ImageNet-based pretrained ResNet32 encoder, in which researchers minimized the KL-divergence between its output and the empirical user distributions of ArtEmis.
The researchers say the task of predicting fine-grained emotions explained in ArtEmis data remains challenging due to intrinsic difficulties involved in producing emotion explanations in language. Nonetheless, the team observed in experiments that the best of their neural speaker models was able to produce “well-grounded affective explanations, respond to abstract visual stimuli, and fare reasonably well in emotional Turing tests, even when competing with humans.”
The paper ArtEmis: Affective Language for Visual Art is available on arXiv, and the ArtEmis dataset and newly proposed neural speakers can be found at artemisdataset.org.