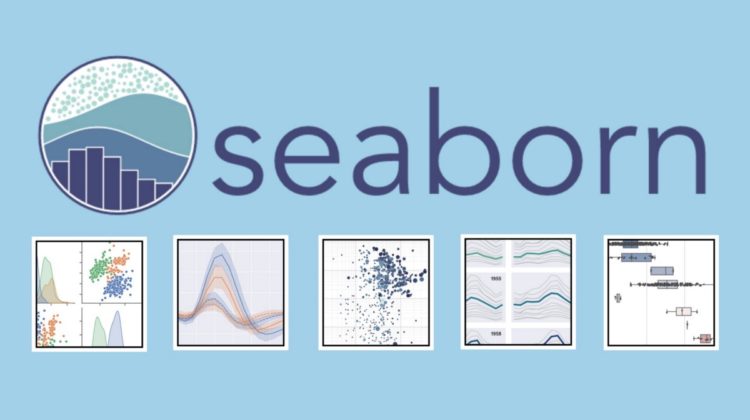
We now know the basics of seaborn
. Let’s put them into practice by building multiple charts over the same dataset. In our case, we will use the dataset “tips” that you can download directly using seaborn
.
First, load the dataset:
tips_df = sns.load_dataset('tips')
tips_df.head()
I like to print the first few rows of the data set to get a feeling for the columns and the data itself. Usually, I use some pandas
functions to fix some data issues like null
values and add information to the data set that may be helpful. You can read more about this on the guide to working with pandas.
tips_df["tip_percentage"] = tips_df["tip"] / tips_df["total_bill"]
tips_df.head()
Next, we can start plotting some charts.
Understanding tip percentages
Let’s first try to understand the tip percentage distribution. For that, we can use histplot
, which will generate a histogram chart:
sns.histplot(tips_df["tip_percentage"], binwidth=0.05)
That’s good. We had to customize the binwidth
property to make it more readable, but now we can quickly appreciate our understanding of the data. Most customers would tip between 15-20%, and we have some edge cases where the tip is over 70%. Those values are anomalies, and they are always worth exploring to determine if the values are errors or not.
It would also be interesting to know if the tip percentage changes depending on the time of day:
sns.histplot(data=tips_df, x="tip_percentage", binwidth=0.05, hue="time")
This time, we loaded the chart with the full data set instead of just one column. Then, we set the property hue
to the column time
. This will force the chart to use different colors for each value of time
and add a legend to it.
Total of tips per day of the week
Another interesting metric is to know how much money in tips the personnel can expect depending on the day of the week:
sns.barplot(data=tips_df, x="day", y="tip", estimator=np.sum)
It looks like Friday is a good day to stay home.
Impact of table size and day on the tip
Sometimes, we want to understand how variables play together to determine output. For example, how do the day of the week and the table size impact the tip percentage?
To draw the next chart, we will combine the pivot
function of pandas to pre-process the information and then draw a heatmap chart:
pivot = tips_df.pivot_table(
index=["day"],
columns=["size"],
values="tip_percentage",
aggfunc=np.average)
sns.heatmap(pivot)