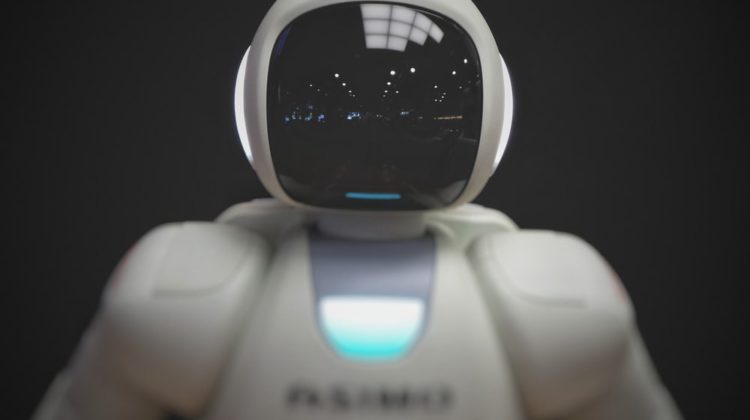

Posted on February 24th, 2021 by Emilio Miles
Cathy O’Neil, author of the book Weapons of Math Destruction, describes an AI model as nothing more than an abstract representation of a process. A model takes what we know and uses that information to predict responses in various situations. In her book she uses various different scenarios to describe how models work.
For example, in baseball she uses the idea of statistics to show how coaches make decisions. If a player tends to hit a ball to the right side of the field, the coach might have more players cover that area in order to get more outs. A player’s stats, such as home run percentage, might influence a coach’s decision to sign him. She claims that this type of model, one which is transparent, factual, and constantly evolving, is a trustworthy model.
Another example she uses is more informal. She talks about how her cooking is influenced by the ingredients she has available, her time, her energy, and the information she has about her family. The model would be evaluated and tweaked depending on how well her food was received. This is a model that is tailored to her family and would only work with them. This model is based more on opinion and values. She values health, happiness of her family, and convenience, so her model reflects that.
The last example she mentions is that of LSI-R, a test used to determine a criminal’s risk of recidivism. The test is meant is to eliminate discrimination or prejudice from judges. She points out that this is a model affected by bias. It contains several highly relevant questions to assess risk of repeated offense, but as the questions continue, the test delves deeper into a person’s life, asking questions that would normally be dismissed in court. Some of these questions are biased in that they are more likely to be answered in a way that a person raised in a middle-class neighborhood is more likely to be seen as lower risk as opposed to a person raised in a low-income community. Things such as a person’s upbringing, friends, and family are included in these questions. For example, a person raised in a wealthy community would know less people/have fewer friends that have been in trouble with the law as opposed to someone in a low-income community.
Convicting a less fortunate person, having them be around other criminals, and then throwing them back into their community would then not only make it harder to get a job, but it would make it more likely for them to commit another crime. Then, this model can claim to be “successful” by correctly predicting recidivism. However, it is the model that contributes to this cycle and thus helps to sustain itself.
In order to identify a bad model, and perhaps a biased one, one must ask the questions:
- Does the model work against the subject’s interest?
- Is it unfair?
- Does it cause harm?
It can be very dangerous if mathematical models simply appear to work. Even if originally created to fix a problem, when they cause more harm than good, these models must be re-evaluated. There has to be a point in which we ask ourselves, is it ethical?