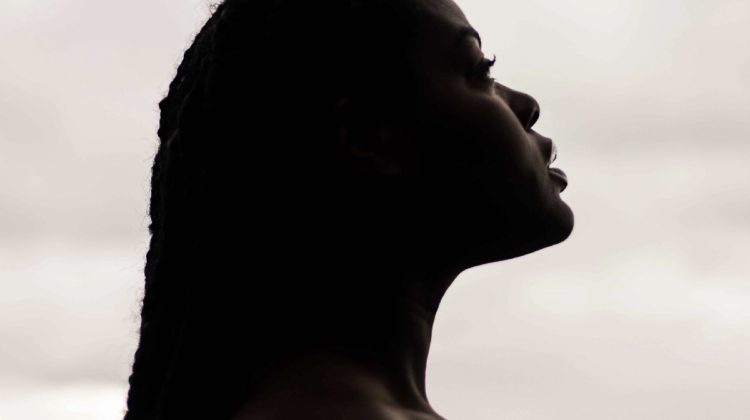
Understand how racial and gender biases in artificial intelligence systems occur and how to address them

In April 2019 New York University’s AI Now Institute released a report into the impact of bias in artificial intelligence systems. About 12 months later, you might remember the Twitter the storm caused when a pixelated image of Barack Obama was turned into a higher resolution image of the former president by an AI model. Except the model returned a high-resolution image of a white man when it was given a pixelated image of a black man. This is a classic example of the bias The AI Now Institute detailed in that NYU report. As we increasingly integrate artificial intelligence systems into our daily lives, I think it is paramount to describe these problems and open dialogue about how to address them. Before we take a look at how these biases come about and what action needs to be taken to address it, let’s first take a quick look at how supervised machine learning algorithms work.
How do supervised machine learning algorithms work?
The aim of a supervised machine learning algorithm is to learn a function that best approximates the relationship between input data and target data. Supervised learning algorithms learn from a given training dataset labelled with the desired output. A good algorithm must be generalizable, meaning it must be able to accurately predict the output of new data based on what was learned in the training data. If the incoming new data contains features not previously seen in the training dataset, the AI will have trouble classifying what this new data is. If this all sounds a bit abstract, here is a quick example. Imagine you want to train an ML model that classifies if a photograph has a horse or a dog in it. In the training dataset, you feed it hundreds of thousands of labelled images of chihuahuas, and stallions. If you show your model an image of a Great Dane, it will likely misclassify this as a horse. The model is not generalisable enough to all dog breeds because it has not been trained with images of other dog breeds.
How does bias occur in ML models
The simple answer is, biased ML output occurs because of biased training data. But of cause data itself isn’t inherently biased, the real reason bias occurs in AI is that the human beings in charge of supplying the training data have the biases or fail to notice biases in their data.
Failure to notice biased data is a subtle concept but results in just as bad outcomes as carelessly collecting biased data. Look at Amazon’s recruitment tool from 2015 as a classic example. The tool was supposed to better automate the selection of top candidates for recruitment to the company. The model was apparently trained on CVs from a decade prior. In theory, so far so good. There should be a good mix of people in that CV pool. Not so in reality, the applications for these positions were even more biased towards men in 2005 than they were in 2015. The end result being the tool was found to be biased against perfectly qualified women, and Amazon quickly abandoned the whole project when it became apparent what was going on. As far as the model was concerned, men are the best candidates for the job because all the training data seemed to suggest as much.
Another reason why biases occur in AI is the lack of representation in the companies that build these models. Here are some statistics from the AI Now Institute report; 80% of professors in artificial intelligence are white males; between Google, Facebook and Microsoft, none of them had a workforce with more than 4% black people. The numbers for women in the field are equally bleak, despite a push for more women in tech. Further, the people in the field tend to be wealthy which introduces potential for socioeconomic biases
How do we address this?
The first step in addressing this issue, I think, is acknowledging the problem. I see encouraging signs here because AI professionals and institutes appear to recognise these issues and are working towards addressing them. Another step that should be taken towards addressing bias in artificial intelligence systems is inclusion of people biased against at all levels of companies that develop and distribute the systems. Groups biased against need representation in leadership positions at these companies. The numbers for minority groups, women and disabled people for some tech companies seem to be moving in the right direction, but it’s not clear how many of these people are in positions to make decisions that affect the biases of their products.
Concluding remarks
Like in many areas of our society, we are seeing unacceptable biases in our artificial intelligence systems. This is a problem that requires addressing sooner rather than later as these systems integrate into more aspects of our lives, and companies use them to make decisions that affect entire communities. Encouragingly, artificial intelligence research institutes such as the AI Now Institute recognise the seriousness of the issue are dedicated to addressing it. It now falls on tech companies to do more in having representation of women, people of colour and those with disabilities in leadership positions and empower them to be part of the solution to biases in AI.
I will leave you with what I thought was a powerful quote from Professors Ayanna Howard and Charles Isbell discussing AI racial biases.
“Sometimes people of color are present, and we’re not seen. Other times we are missing, but our absence is not noticed. It is the latter that is the problem here.”