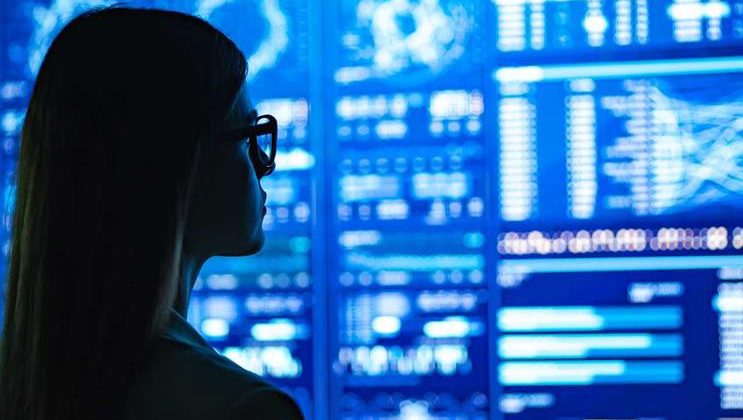

Machine Learning adoption is widespread across all industries. Its ability to perform specific tasks better than humans creates tremendous opportunity to improve economic productivity. The global machine learning market is projected to attain a Compounding Annual Growth Rate (CAGR) of 43% between 2020 and 2024, growing from $7.3b to $30.6b. As practitioners of ML applications, we are all incentivized to focus on the benefits of this technology in order to procure investment and resources.
However, I think we need to pause and introspect on it’s shortcomings just as much as we tout its benefits. Recognizing that Machine Learning can impact lives — on such a large scale and in such rapid speed — at the press of a button, we have a responsibility to make thoughtful decisions on its applications. After all, our children will live in this world we are creating.
As a student of Data Science, I am tremendously excited about its potential. However, the more I delve into the subject, the more I also recognize its deficiencies. I believe this is a good thing, and this is something all Data Scientists need to embrace more. We need to discuss the shortcomings of the models we build just as much as we espouse their benefits, to raise awareness and focus our resources towards building better, not necessarily more, artificial intelligence. In this blog, I’d like to broadly cover the use cases of Machine Learning, while also providing examples of where ML has gone wrong and the lessons we should learn from it.