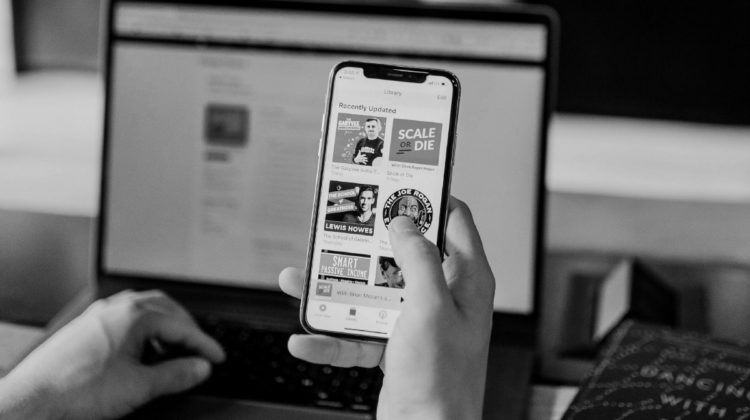
Another interesting case was investigated by Woerndl & Groh in 2007, where the team looked at the first real implications of context awareness within recommendation systems [4]. This paper focuses on the belief that one size does not fit all for these recommendation systems. As such, different contexts do not play the same role across the board in the success or failure of systems in different domains, like music and news. The paper showed the efficacy and implications of physical context on mobile app recommendations, showing that location can be very important when addressed by a system showing specific bits of content for the user to consume. Another approach the team took was to take social context into account when looking at collaborative filtering. Collaborative filtering is implemented by many recommender systems today, and you can thank it for recommending you items your friends and family enjoy and talk about.
The social and locational contexts were the beginning of an understanding that different contexts influence how a user perceives recommended content, and had far-reaching implications on recommender system development in the years that followed. It can be extrapolated then, that high cognitive loading as a result of physical location and social interaction placed upon a user would result in a more successful system, following these guidelines.
While the studies seem to point towards the importance of cognitive loading, it is important to consider the counter-argument, that it is in fact less significant or not significant at all to a user’s choice to conform to a recommendation or not.
In their study from 2012, Odić et al. presented a framework for determining which contexts are relevant and irrelevant to a recommender system’s success. They found that day type, season, weather, and health of the user were irrelevant in the recommendation system’s success. As such, it can be said that given a day is a weekend day, when users would most likely be under a lower cognitive load throughout the day than a weekday, this context actually plays a lesser role in the success of that recommendation system. The same could be said for a stormy winter day, or perhaps when the user is deeply ill with the flu.
On the whole, the contexts that this team found to be relevant support the argument around higher cognitive loading leading to higher success rates for a system. For example, time, location, mood, and the number of interactions the user has had with the system up until this recommendation are all relevant to its success or failure. Considering these contexts, a user who is accessing the recommendation late at night might be heavily influenced by their thoughts on other subjects, and as such lead to a successful recommendation. The same holds true for someone in a strange location or in a foul mood due to some other circumstances.
It can be argued that the general concept of cognitive loading is far too broad to make any specific claims about when it comes to the success or failure of recommendation systems. While specific contacts are perceived by users and generally the same way, the actual impact of these contacts on those users can be different from person to person, based on priming as well as past contextual interactions those same users of had throughout their history with a product. It was Smart of these researchers to focus in on specific areas and contexts, lest they become lost in a sea of broad terminologies and little to no specific findings about them.
Throughout my research, I have seen a multitude of evidence in support of the claims at a higher cognitive loading has a larger implication for someone when they are faced with a recommendation. We have seen the systems that are more highly aware of specific contacts that a user might be interacting with them and are more successful in recommending content to that user. We’ve also seen that while some of these specific contacts are relevant to the success of that system, others that might be classified as a high cognitive load situation have been deemed irrelevant.
I have discovered that throughout my search, perhaps I am asking the wrong question of the users of these recommender systems. The concept of cognitive loading could come from any number of different contests and situations, and while the end result might be the same, in this case a more successful recommendation, it is important to consider these different contacts, as they might be confounding. As the research has shown, focusing in on specific contacts like mood and Time might be much more relevant to a successful recommendation, rather than just whether a users mind is on something else or not. If the system can focus in on these contacts and become aware of them through interactions with the user, the more successful the system will become.
Citations
- Pinata Winoto, Tiffany Y. Tang. The role of user mood in movie recommendations, Expert Systems with Applications, Volume 37, Issue 8,
2010, Pages 6086–6092, ISSN 0957–4174, https://doi.org/10.1016/j.eswa.2010.02.117. - Zheng Y., Mobasher B., Burke R. (2016) Emotions in Context-Aware Recommender Systems. In: Tkalčič M., De Carolis B., de Gemmis M., Odić A., Košir A. (eds) Emotions and Personality in Personalized Services. Human–Computer Interaction Series. Springer, Cham. https://doi.org/10.1007/978-3-319-31413-6_15
- Jin, Y, et al. “How Do Different Levels of User Control Affect Cognitive Load and Acceptance of Recommendations?” Jin, Y., Cardoso, B. and Verbert, K., 2017, August. How Do Different Levels of User Control Affect Cognitive Load and Acceptance of Recommendations?. In Proceedings of the 4th Joint Workshop on Interfaces and Human Decision Making for Recommender Systems Co-Located with ACM Conference on Recommender Systems (RecSys 2017), vol. 1884, 2017, pp. 35–42.
- W. Woerndl, G. Groh, Utilizing physical and social context to improve recommender systems, Proceedings of the 2007 IEEE/WIC/ACM International Conferences onWeb Intelligence and Intelligent Agent Technology-Workshops, IEEE Computer ociety 2007, pp. 123–128.
- Kosir, Andrej & Tasic, Jurij & Odic, Ante & Tkalcic, Marko. (2012). Relevant context in a movie recommender system: Users’ opinion vs. statistical detection. CEUR Workshop Proceedings. 889.