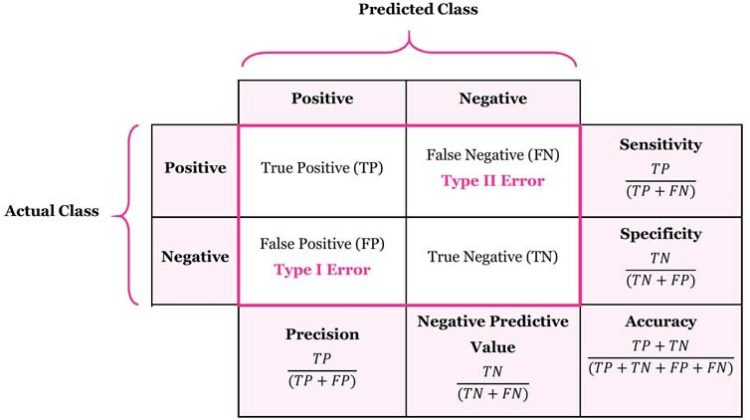

The confusion matrix is a matrix used to determine the performance of the classification models for a given set of test data. It can only be determined if the true values for test data are known.
Some features of Confusion Matrix:
1) For the 2 prediction classes of classifiers, the matrix is of 2*2 table, for 3 classes, it is 3*3 table, and so on.
2)The matrix is divided into two dimensions, that are predicted values and actual values along with the total number of predictions.
3)Predicted values are those values, which are predicted by the model, and actual values are the true values for the given observations
Meaning of the terms used in picture below:
1) True Negative: Model has given prediction No, and the real or actual value was also No.
2) True Positive: The model has predicted yes, and the actual value was also true.
3) False Negative: The model has predicted no, but the actual value was Yes, it is also called as Type-II error.
4) False Positive: The model has predicted Yes, but the actual value was No. It is also called a Type-I error.