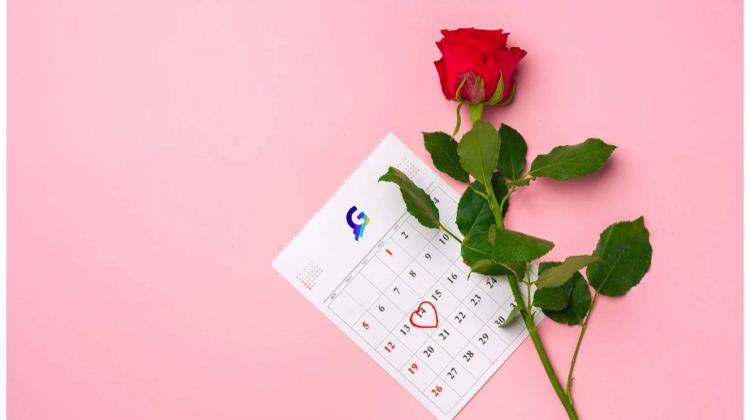

Creating and crafting data science projects that have staying power and impact business value is a central focus for our data science team at Gesture and we aim to refine our commitment to creating a data driven environment continuously.
With Valentines Day on the horizon, we thought we would share some love with our broader data community and share some of the best practices that have worked for us. This is a tricky road to navigate for a lot of data science and analytics teams: how do we create and use tools that work for us and are used by our business partners? Defining and framing the problem we’re trying to solve, creating a solution, approach or design and translating the insights from that design into actions that change the way the business works and improves is a challenge for companies of all sizes. There must be a clear connection from data to analysis to insight and ultimately: to action.
Here are the top data best practices that have worked for us that we hope you can take advantage of in your business as you grow out your data science and analytics teams!
Start with one key project to focus on so that your data science and analytics team can get some street cred.
All companies have some political component to them. Even if your company’s culture is low key and laid back, all your business teams will need to understand what the data and analytics team does and why they should care about them. Creating good PR around your data team is key to ensuring a data first culture at your company.
Focus on one really important, relevant project that will get eyes on your data team from all aspects of your organization from marketing to operations to sales to finance. Make sure you have executive sponsorship for your project, have clear metrics and KPIs to measure the impact of your results and make that data available so that everyone can see the fruits of your efforts! Maybe it’s dashboards, maybe it’s a customer acquisition tool, maybe it’s a recommendation system. Whatever it is, make sure it’s a good first win that everyone can use and appreciate.
Define your DS & A team structure so that everyone understands the function of the key roles in the team.
At Gesture we have a hub and spoke model. We have a key DS hub and we have analytics folks that sit with sales and marketing and effectively serve as our sales and marketing partners within the company. This allows us to have a core team that focuses on high level data initiatives and refining the strategy of the data science team while also creating stakeholders that sit in other teams at the company for more specific projects and tasks.
Data democratization is a big part of the success of a data science organization and having folks working on a core team accompanied by satellite teams in various other groups allows for the kind of synergy that the data stakeholders need within the company to make their data projects come to life continue to see the light of day in their relevance. Making sure that we have cross functional talent and sponsors is crucial to ensuring all our teams are practiced at being data driven and delivering impactful results.
Have recurring meetings with marketing, finance, product and sales. You’ll thank us later. Communicate with your business partners often and keep those lines of communication open. Don’t be an island. Your colleagues won’t bite and they’ll appreciate your leadership.
Define your tools & KPIs
What works for one data organization might not work for another. Are you a python house or an R house? Where are you warehousing? What are you using for analytics and dashboarding? Are you on the cloud or behind a firewall? Where are you deploying models? How are you measuring your performance? Choosing tools and environments is a story for another blog post but what’s really important here is you make a choice that’s relevant for your journey as a company.
Decide on infrastructure that fits your corporate identity as well as your data team assets strategy. Metrics are incredibly important to define on the onset so that your data stakeholders are speaking one language and they can leverage their tools to the best of their ability. ROI, cost or revenue metrics will likely get you pretty far when you’re trying to get the word out on the results of your data and analytics projects. Data folks will need to tie the impact of their data tools to leadership in words that make sense to non technical people so think in terms of cost, profit and customer journey/service metrics.
Proof of concept your data science projects with buy in
Creating an agile approach to how you ideate and execute on your data science projects will help you. Break up your project into 2–3 week springs and invite key stakeholders within your organization to your planning meetings. Have an easy to digest dashboard showcasing your proof-of-concepts so that non technical leaders can understand the scope of your project and what business problem it’s attempting to solve.
Coming up with data science projects that sound enticing and snazzy is fun, but if the political will for it isn’t there, if it isn’t easy to understand the relevancy of your project ideas and what they’re doing for the business and, ultimately, if there isn’t the infrastructure in place to deploy your project, they’re just castles in the sky.
Data science and analytics is fun and there are many creative detours you can take with it. Make sure you’re doing what you can to help your business leveraging your data science team to the max!
Good luck!
Irene Bratsis, Gesture Data and Analytics Team