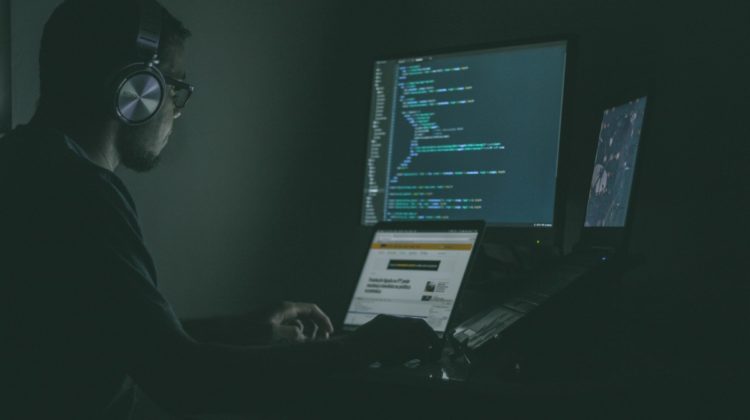
Accuracy and supremacy of AMTENnet
AMTENnet was tested against the following state-of-the-art baseline models:
In addition, other state-of-the-art modules were used to replace the AMTEN module, thus generating several hybrid models. These modules were SRM filter kernels by [Zhou et al., 2018], Constrained-Conv by [Bayar and Stamm, 2018] and hand-crafted feature extractor by [Mo et al., 2018].
Two datasets were used to train, validate and test all networks and can be found on GitHub here and here. The training, validation and testing were done for two cases; First, classification of face image manipulation techniques; Second, binary classification of face image manipulation (Is the image fake or not).
The results have shown that AMTENnet has a higher detection accuracy and generalization capability, and particularly for Multiple classification of facial image manipulation techniques, it has an increase of up to 7.61% in detection accuracy. For the binary classification dataset, AMTENnet had an increased around 1%, which although is low it is still better. To put numbers in perspective for the multiple classification problem, MISLnet achieved 74.03% of accuracy, whereas AMTENnet achieved 81.13% of accuracy. I encourage readers to check the full article here to examine all the results.