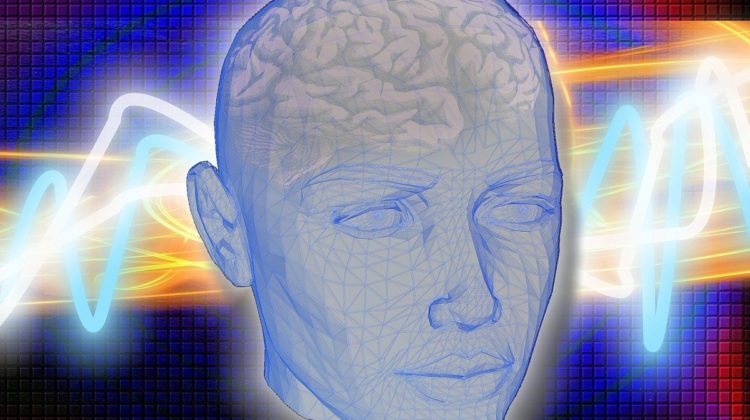

The familiar adage a picture is worth a thousand words especially resonates in the world of healthcare. In the realm of radiology, it could more appropriately be said that a picture is worth a life. Since the arrival of the X-ray in the 19th century, the use of imaging in medicine has been steadily growing and has become an indispensable part of patient care, from screening to post- procedure follow-ups. Imaging is not just an aid for diagnosis, but also helps in creating a roadmap for surgery — from giving coordinates for precisely placing stents in the brain and coronaries, to guiding the delivery of radiation seeds in the prostate.
The use of radiological images has flourished; however, the growth in the number of professional radiologists has stagnated. Automation and artificial intelligence (AI) have made their way into the field, addressing the challenges posed by worker shortages and the increase in use of imaging tools. Yet, challenges still remain in the field of radiology, namely, (i) a rapid increase in volume of data coupled with a continued shortage of radiologists and (ii) the need to quickly deliver precise diagnoses to an array of specialists, often including the patients.
In a recent publication by Smith-Bindman et al.1, it was reported that at seven healthcare systems in the United States and Ontario, Canada, over 135 million imaging studies were performed from 2000 to 2016, with the rate of imaging growing in double-digits, though at a slower pace. In addition, the latest National Health Service (NHS) report2 highlighted that between June 2019 and May 2020, there were 40.4 million imaging tests performed in the United Kingdom. Globally, it is estimated that about 3.6 billion diagnostic X-ray examinations3 and 40.4 million MRI studies4 are performed every year.
However, such volumes are straining radiologists. For example, radiologists often need to review a whopping 4000 images in a CT scan of multiple body parts for patients with multiple trauma5. Unfortunately, the number of professional radiologists is not scaling with the amount of imaging studies. It is estimated that there are about 100 radiologists per million people in the United States, while in the United Kingdom, there are merely 7 radiologists per million people. Furthermore, the WHO estimates that about two-thirds of the global population lack access to proper diagnostic imaging procedures, and with the fast growth in radiological exams this situation could be exacerbated. Additionally, radiologists prefer to work at urban centers, resulting in a shortage of imaging services for rural communities.
Digitization has the power to solve the two challenges discussed above, as well as the problem of delivering fast and precise diagnoses. A considerable amount of time can be saved by applying digital tools to routine, non-challenging procedures such as detecting lung nodules, pneumothorax, hemorrhagic stroke, and more. Moreover, the same digital tools can augment radiologists with expedited and precise diagnoses of complex procedures. Below I will discuss some examples of frontiers in digitization.
Hyper-automation in Teleradiology
Teleradiology has been in use for quite some time but is currently on overdrive because of the radiologist shortage and the ongoing COVID-19 pandemic. In fact, one can expect a further increase in the use of teleradiology for stroke care due to recent recommendations by the American Heart Association (AHA) and the American Stroke Association (ASA), and due to updated reimbursement codes for telemedicine.
Digital tools used in teleradiology towards virtual consultation, workflow automation, and process automation are efficient and deliver timely results to patients and other specialists, thus relieving stress to the strained radiology community. Digitization also, to an extent, solves the aforementioned problem of the rural-urban divide by enabling radiologists to focus on coordination of care in rural areas. Since teleradiology handles images and patient data from disparate sources, artificial intelligence can support standardization to make radiological workflow hassle free. Moreover, the use of augmented reality can make teleradiological consultation more impactful, especially for communities that lack radiological resources.
Augmented Intelligence
AI based algorithms enable the delegation of some tasks to nurses, radiographers, and clinicians who aren’t specialized in imaging. Based on the probability score from the AI algorithm the allied staff can alert the specialists for further review to make appropriate clinical decisions. For example, DL algorithms can detect missed fractures6 and can identify and characterize microcalcifications in screening mammograms. Incorporating these algorithms, for instance, in a screening procedure or in emergency departments, could result in efficient patient care pathways and also lessen the burden on radiologists.
Furthermore, through the use of neural network based algorithms (NN), digitization can map AO-Müller/Orthopedic Trauma Association (AO/OTA) classifiers of X-ray and CT images of orthopedic trauma patients. The algorithm’s classification will augment clinical workflow and enable radiologists to be involved in making image-guided road maps for surgical procedures, thus aiding trauma surgeons. Application of NN algorithms in image guidance, specifically three dimensional imaging, has been proven to reduce revision surgeries, which not only saves costs but also has a valuable impact on the patient’s quality of life.
AI can also be beneficial in detecting secondary and incidental findings, the latter being greatly important. When I was developing bilateral breast MRIs protocols7, the radiologist working alongside me located a nodule in the patient’s chest cavity in a few images. With further investigation, it was found that this had no clinical significance, but this did add additional overhead as the radiologist had to scour through multiple images and the patient’s clinical history. A well-trained algorithm can help avoid situations like this by detecting incidentalomas from the images that are outside of the referral for primary indication.
Relating to secondary findings in non-contrast computed tomography (NCCT) images, AI has the potential to pick distal lesions triggered by large vessel occlusions. In patients suspected to have had a stroke, NCCT is the first imaging procedure performed to rule out hemorrhage from an ischemic event. Tolhuisen et al.8 have shown that there are hyperdense signals in NCCT images that correlate with an ischemic event, which cannot at times be picked up by experienced radiologists as the signals are buried in background noise. Convolutional neural network (CNN) algorithms can be used to isolate these distal lesions early in the journey of stroke patients during the golden hour. This is of tremendous value in stroke care coordination, as it minimizes the use of contrast agents and radiation, therefore having a positive impact on patient outcomes.
ML algorithms are also currently being used in stroke care to extract important imaging biomarkers from CT and MR images such as tissue perfusion, oxygenation, and time to peak. Digitization in radiology delivers additional quantitative imaging biomarkers across the stroke care pathway from initial screening NCCT to post intervention MRI. These biomarkers, in combination with other data, can be used as prognostic indicators, benefitting care providers and the patients alike.
Radiology and radiologists have embraced digitization with the advancement in the field of computer vision and artificial intelligence, combined with cloud technologies. Digitization will not completely replace radiologists, but rather augments their clinical services, ensuring fast and precise diagnoses. In addition to the examples of digitization discussed above, more recently, augmented reality (AR) and virtual reality (VR) are some of the latest digital technologies radiology. Specifically, AR & VR are being used in the pre-planning of surgical procedures such as pedicle screw placement in spinal fusion and overlaying pre-op brain images for neurosurgical simulation. The use of these new technologies enables seamless interaction with images and the integration of patient clinical information, supporting radiological training and education as well. Through this ready embrace of technology, whether its teleradiology or virtual reality, radiologists, empowered with digitization, are truly able to make an image worth a life.
- Smith-Bindman, Rebecca, Marilyn L. Kwan, Emily C. Marlow, Mary K. Theis, Wesley Bolch, Stephanie Y. Cheng, Erin J. Bowles, et al. 2019. “Trends in Use of Medical Imaging in US Health Care Systems and in Ontario, Canada, 2000–2016.” Journal of American Medical Association 322 (9): 843–856.
- National Health Service. 2020. “Diagnostic Imaging Dataset Statistical Release.” Statistics. https://www.england.nhs.uk/statistics/wp-content/uploads/sites/2/2020/09/Provisional-Monthly-Diagnostic-Imaging-Dataset-Statistics-2020-09-24.pdf.
- Pan American Health Organization. 2012. “World Radiography Day: Two-Thirds of the World’s Population has no Access to Diagnostic Imaging.” PAHO. https://www.paho.org/hq/index.php?option=com_content&view=article&id=7410:2012-dia-radiografia-dos-tercios-poblacion-mundial-no-tiene-acceso-diagnostico-imagen&Itemid=1926&lang=en.
- Statista. 2020. “Number of magnetic resonance imaging (MRI) units in selected countries as of 2019.” Statista. https://www.statista.com/statistics/282401/density-of-magnetic-resonance-imaging-units-by-country/.
- Jha, Saurabh, and Eric J. Topol. n.d. Journal of American Medical Association 316, (22): 2353–2354.
- Jones, Rebecca M., Anuj, Sharma, Robert Hotchkiss, John W. Sperling, Jackson Hamburger, Christian Ledig, Robert O’Toole, et al. 2020. “Assessment of a deep-learning system for fracture detection in musculoskeletal radiographs.” npj Digital Medicine 3, no. 144 (October): 1–6.
- Friedman, Paul D., Srirama V. Swaminathan, and Robert Smith. 2005. “SENSE Imaging of the Breast.” AJR Am J Roentgenol . 184, no. 2 (February): 448–51.
- Tolhuisen, Manon L., Elena Ponomareva, Miou S. Koopman, Ivo G. Jansen, Anna M. Boers, Charles B. Majoie, and Henk A. Marquering. 2019. “Artificial Intelligence Based Detection of Large Vessel Occlusion on Non-Contrast Computed Tomography in Stroke.” Stroke 50, no. Suppl 1 (January): 1.