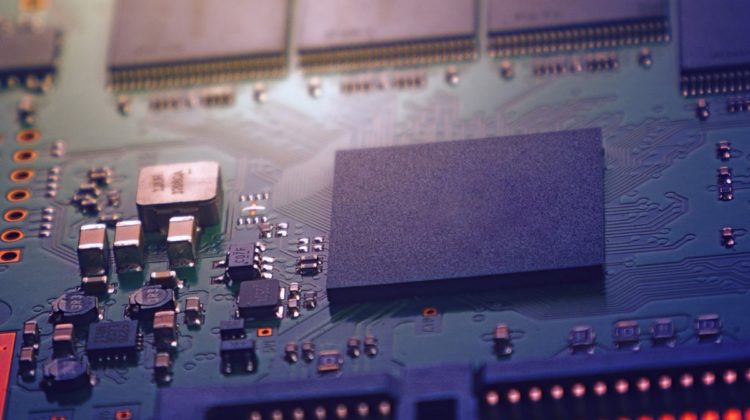

Written by Tony King-Smith, Product Manager of aiWare
Many automotive system designers, when considering suitable hardware platforms for executing high-performance NNs (Neural Networks) frequently determine the total compute power by simply adding up each NN’s requirements — the total defines the capabilities of the NN accelerator needed. Or does it?
The reality is almost all automotive NN applications comprise a series of smaller NN workloads. By considering the many forms of parallelism inherent in automotive NN inference, a far more flexible approach, using multiple NN acceleration engines, can deliver superior results with far greater
scalability, cost-effectiveness and power efficiency…