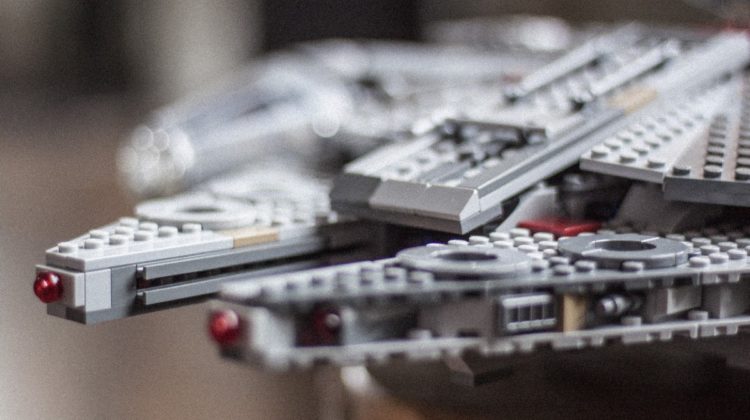
If you don’t know what Universal Approximation Theorem is, just look at the above figure. It pretty much explains itself. No, I’m just kidding. We’ll not go the heavy math route. Instead, I’ll try to explain it as simply as possible so even if you don’t know much about math or function approximation, you can still understand. Put on layman’s terms, UAT just means that giving a one hidden layer neural network with enough neurons. It can approximate(or simulate closely) any continuous function within the given input range. I’ll give you two figures to quickly explain what this means.
The above figures how neural networks with different sizes (number of neurons) approximate the function shown in the red curve. Instead of trying to be clever and find some mysterious curves that can add up to this red function curve above, what neural network does is split the curve into many many small bins and approximate each bin using one neuron (not exactly, but the main idea is this). If there are a lot of neurons, the bins will be quite small, and the target function curve with each bin becomes very ‘linear’, and the error will be sufficiently small. Given different inputs, it will fall into one bin and thus ‘activate’ one neuron. This neuron can get trained to give a value closes to the training data. That’s it. The above figure only shows a 2-dimensional function. Let’s look at a 3-dimensional function:
A 3-dimensional function is not that different, the bins now become cubes, and the UAT still stands. With neurons as the base granularity, neural networks are infinitely flexible and can ‘fit’ into any functions you throw at them. This is also why we usually call training a model ‘fit the model.’