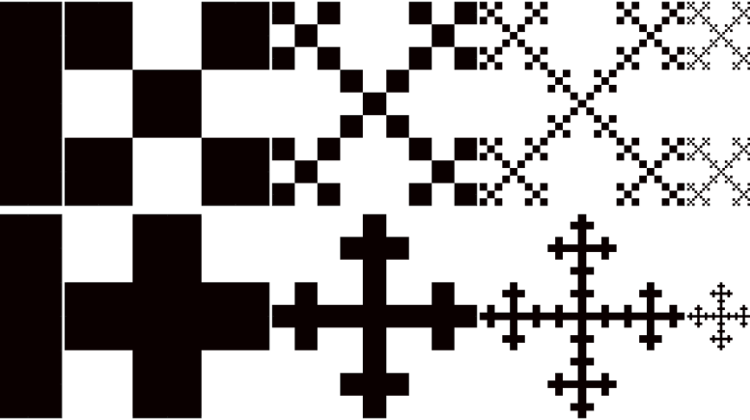

2020 was a mixed year for Artificial Intelligence: GPT-3 wowed many with its generative capabilities and Timnit Gebru lost her job for pointing at the risks of the kind of models it represents. AlphaFold 2 predicted the structure of proteins with unprecedented accuracy towards the end of a year when AI failed to make a significant contribution in the fight against Covid-19.
These disparate yet connected events point at AI’s expanding influence and impact but also at its limitations, and at the complexity of its development and deployment.
They also suggest three directions for AI ethics researchers looking to steer AI’s trajectory towards the common good and respect for human values.
AI ethics tends to focus on the mitigation of ethical risks created by existing AI systems, in particular around fairness and privacy. More recently, AI ethics researchers have started to pay more attention to questions of power and inclusion — for example under the rubric of participatory ML — and developed methods to involve relevant stakeholders and communities in the deployment of AI systems to make it more beneficial and empowering. But even this progressive work takes AI systems and technologies as exogenous.
“On the Dangers of Stochastic Parrots: Can Language Models Be Too Big?”, the paper that ostensibly led to Timnit Gebru’s dismissal from Google Brain, breaks away from this approach and questions the ethical desirability of AI’s dominant technological trajectory along several fronts: today’s state-of-the-art AI systems have to be trained on massive and hard to curate datasets that include racist, sexist and inflammatory content. They rely on big computational infrastructures that create environmental costs that are primarily imposed on vulnerable groups and communities. They provide an illusion of progress in AI capabilities (“to understand language”) while diverting resources and talent away from alternative techniques. The issue at stake is not how to mitigate the risks that these AI systems create, but whether we should develop them at all (or at least to the extent we are), given those risks. Perhaps unsurprisingly, this is a controversial thesis for Google, a company that has invested billions of dollars in the development of large language models and embedded them deeply in many of its products and services.
If some technologies are intrinsically more likely to create ethical risks, this also means that ethical considerations should be taken into account when AI technologies are starting to be developed as well as further downstream when their risks are identified but harder to mitigate. As Gebru pointed out in an interview soon after being ousted from her position at Google Brain: “You can have foresight about the future, and you can get in early while products are being thought about and developed, and not just do things after the fact”.
This upstreaming of AI ethics would make it easier to raise a central ethical question about new technologies: when not to create them. Developing frameworks and processes to inform this decision in the context of pervasive uncertainty that characterises the early stages of an innovation, and given the intrinsic dual nature of many AI technologies should be a driving concern for AI ethicists in future work.
This brings me to a second potential direction for AI ethics research, concerning the type of organisations that undertake it.
The Gebru case has cast serious doubts on the idea that private companies can be trusted to be impartial when they assess the ethical risks of the AI systems that they develop. AI ethics researchers who have called for increasing diversity in the AI workforce with the goal of mitigating biases and blindspots that may lead to the development of discriminatory AI systems should reflect on how the organisational environments where they operate may, in a similar way, skew their research agendas implicitly and explicitly. This could create AI ethics meta-risks — the risk that AI ethics neglects some ethical risks. Perhaps the lack of attention to AI directionality which I pointed to above is an illustration of this.
One implication is that the AI ethics community needs to create an effective division of labour between researchers in industry, academia, government and the third-sector. This requires determining what AI ethics questions can be better explored outside of industry because they require a degree of impartiality that is unfeasible or unsustainable in the private sector and which ones are more suitable for an industry context, for example because they require deep knowledge of specific technologies or development processes.
It will also be important to put in place institutional mechanisms and incentives to create and sustain a healthy flow of knowledge and ideas between public and private research domains while preserving a degree of independence and contestation. These efforts should be underpinned by a stronger understanding of the way in which different organisational and social contexts shape the development and deployment of AI ethics. This is a fertile ground for new AI ethics research in collaboration with other disciplines such as sociology, management science and economics.
I conclude with a third direction for AI ethics that will also require researchers to pay more attention to the organisational and social context where AI systems are deployed — the ethical implications of the adoption of AI in scientific R&D. We can also think of this as a potential research and application site for the two other directions I set out above and will draw their connections throughout.
AlphaFold 2’s success has demonstrated the potential of AI as a driver of scientific productivity and discovery. The ethical risks that it creates have received much less — if any — attention. This is probably explained by the fact that AlphaFold 2 makes predictions about the structure of proteins using open biological datasets so there are at this point less reasons to worry about its implications for fairness or privacy, two focus areas for AI ethics researchers. This is also consistent with the idea that ethical considerations around new AI systems tend to receive attention downstream, reactively, instead of upstream, strategically.
I believe that this is misguided. the indirect impacts of powerful AI systems such as AlphaFold 2 on the scientific enterprise raise important questions that AI ethicists should study in tandem with philosophers of science, epistemologists and science and technology studies and “meta-science” scholars: how will AI transform scientific norms around reproducibility, explainability and publicity of research findings? Will it increase the concentration of research on a small number of institutions — many of which are in the private sector — that have access to data and computation, perhaps neglecting the interests and needs of developing countries and vulnerable groups? Will it devalue scientific problems less amenable to prediction and forms of knowledge such as theoretical and subject-specific knowledge and displace scientific labour in processes of automation that economists like Anton Korinek claim have an important ethical dimension? This assessment of the implications of AlphaFold 2 for structural biology and protein prediction by Mohammed AlQuraishi highlights many of the issues at play.
My own research about the deployment of AI methods in the fight against Covid-19 suggests that AI researchers have focused on problems such as analysing medical scans that are amenable to existing deep learning techniques but of limited relevance to medical practitioners. This brings us back to the idea that the current direction of AI research may be privileging some use cases for AI and downplaying others, and highlights the need to ensure that public interests play a central role shaping agendas of AI in science that are starting to be dominated by the private sector.
Science deeply influences the pace and direction of human progress and our understanding of nature, so it is vital to carefully assess how it stands to be transformed by powerful AI systems developed by private sector companies. As I pointed out earlier in this essay, ethics has a vital role to play informing this process from the very beginning, which is now.
A slightly modified version of this essay appeared originally in the Montreal AI Ethics Institute State of AI Ethics report — you can read it here.