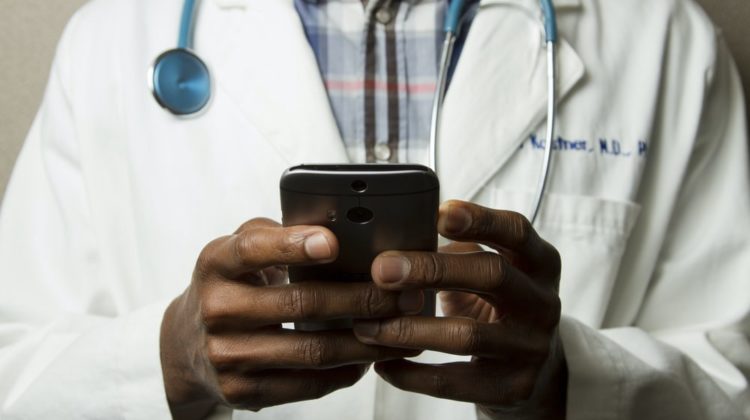
Key governance structures that countries should implement to facilitate and regulate AI adoption

AI development has raised concerns about the amplification of bias, loss of privacy, social harms, disinformation, and harmful changes to the quality and availability of care and employment. We need mechanisms for ensuring responsible development that are more robust than high-level principles.
Governance is the process of interaction and decision-making among those involved in a collective problem that leads to the creation, reinforcement, or reproduction of social norms and institutions. It sets the framework within which organizations conduct their business to manages risk and ensure an ethical approach.
Members of the public are concerned about the risks of AI development and do not trust organizations to govern themselves effectively.
A good example of effective governance is the airline industry:
People who get on airplanes don’t trust an airline manufacturer because of its PR campaigns about the importance of safety — they trust it because of the accompanying infrastructure of technologies, norms, laws, and institutions for ensuring airline safety.
— Brundage, et al. http://arxiv.org/abs/2004.07213
Aircraft manufacturers around the world follow a common, agreed-upon set of guidelines and regulations to make sure that all planes meet a set of safety standards.
Defining standards is key to effective governance. Current AI development guidelines tend to agree on some generic principles, but disagree over the details of what should be done in practice. For example, transparency is important, but what does it look like, and how is it achieved? Is it through open data, open code, explainable predictions…?
To lay out effective governance mechanisms, you first need to define what is important when developing and evaluating a system. The Centre for the Fourth Industrial Revolution at the World Economic Forum put together a set of principles for developing chatbots for health, which provides an excellent example of a well-defined and comprehensive set of principles (summarized below):
- Safety: The device should not cause harm to patients
- Efficacy: The device should be tailored to users and provide a proven benefit
- Data protection: Data should be collected with consent, safeguarded, and disposed of properly
- Human agency: The device allows for oversight, and freedom of choice by patient and practitioner
- Accountability: Device behavior should be auditable and an entity will be responsible for the algorithm’s behaviour
- Transparency: Humans must always be aware if they are interacting with an AI, and its limitations should be made clear
- Fairness: Training data should be representative of the population, and device behavior should not be prejudiced against any group
- Explainability: Decisions must be explained in an understandable way to intended users
- Integrity: Decisions should be limited to those based on reliable, high-quality evidence/data, ethically sourced data, and data collected for a clearly defined purpose
- Inclusiveness: The device should be accessible to all intended users, with particular consideration of excluded/vulnerable groups
Principles require actions in order to be implemented. Going beyond the set of principles laid out by RESET, the guidelines then outline a set of actions around each principle.
The actions are broken down by:
- Which principle is being considered
- Who is responsible (developers, providers, and regulators)
- What phase they should be done in (development, deployment, and scaling)
- What type of device is being developed (four types, stratified by the risks associated)
- Whether they are optional, suggested, or required
Different tools can be used to ensure AI is developed responsibly, each targeting different stakeholders and carrying a different level of power, from norms to laws. The approaches below are an adaptation and expansion of the mechanisms described in this paper:
Institutional mechanisms
Values, incentives, and accountability
- Guides to good practice: produced by developers, providers, or regulators to establish actions developers can take to build trustworthy AI
- Algorithmic risk/impact assessments: assessing possible societal impacts of an algorithmic system before or after the system is in use
- Third-party auditing: a structured process by which an organization’s present or past behavior is assessed for consistency with relevant principles, regulations, or norms
- Red-teaming: a structured effort to find flaws and vulnerabilities in a plan, organization, or technical system, often performed by dedicated “red teams” that seek to adopt an attacker’s mindset and methods
- Bias and safety bounties: give outside individuals a method and incentives for raising concerns about specific AI systems in a formalized way
- Sharing of AI incidents: improve societal understanding of how AI can behave in unexpected or undesired ways
Software mechanisms
Specific AI systems and their properties
- Audit trails: a traceable log of steps in system operation, and potentially also in design and testing
- Interpretability: an explanation of the AI’s decision-making process which fosters understanding and scrutiny of AI systems’ characteristics
- Privacy-preserving ML: software practices to protect the security of input data, model output, and the model itself
Hardware mechanisms
Physical computational resources and their properties
- Secure hardware: to increase verifiability of security and privacy claims
- High-precision compute measurement: to improve the value and comparability of claims about power usage
- Compute support for academia: to improve the ability of those outside of industry to evaluate claims about large-scale AI systems
- Do we need to be more deliberately pursuing AI literacy? With whom? (regulators, decision-makers, the public)
- What legal powers may be missing to enable regulatory inspection of algorithmic systems?
- How do we include marginalised and minority groups who may be most impacted by negative effects in the conversation?
As part of the Chatham House AI for Health Event Series, supported and organized by the Centre for Universal Health in conjunction with Foundation Botnar Youth Council, there will be a Youth Experts Roundtable on the 25th of January. I will be facilitating a discussion of these issues in a breakout session on governance. As a group, we will define three key governance structures for implementing AI in health. Chatham House will be integrating this youth perspective in the Governance Roundtable Event and Conference on Artificial Intelligence for Health: Opportunities, Priorities and Challenges that will follow.