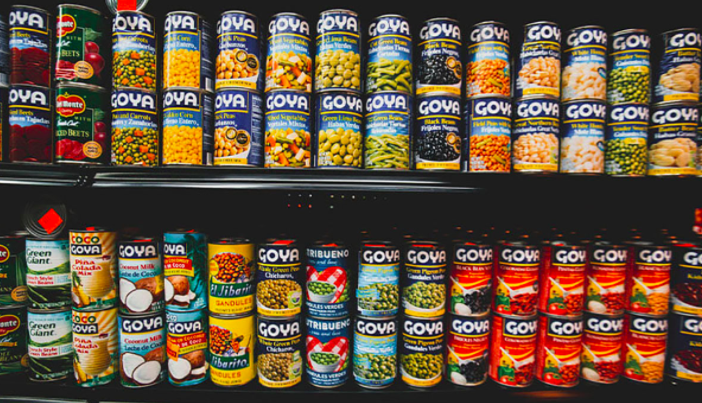

The retail industry is a labor-intensive industry, among all the sections, the cashier settlement cost occupies a considerable big proportion of the total cost.
With the development of deep learning, it is an inevitable trend to use image recognition technology to achieve cost reduction and efficiency increase in the retail industry.
At present, a popular intelligent container solution is “visual recognition solution”, which takes image recognition as the technical core, camera, and mainboard as the hardware core, conducts target detection and classification, realizes automatic identification and settlement, improves the shopping experience and saves labor cost.
Such solutions are already being commercialized in some regions. However, in the process of practical application, some problems are gradually exposed, and the focus is on the accuracy of item identification.
The most common items sold in smart containers are beverages and boxed snacks. In the real scene, the quality of the photos in the inspection can not be quarantined, and it is difficult to have an ideal front shooting environment in the real scene. Meanwhile, the outer packing sometimes doesn’t have many differences, which is confusing AI. All these problems bring great difficulties to the identification of the computer vision algorithm.
=
It is well known that the quality of the data set can greatly influence the effect of the final model. Therefore, improving the quality of data sets is the key to solve such problems.
At present, there are mainly the following types of data annotation commonly used in the new retail field:
Label out all the goods:
2. Polygon
Label out the goods that have been take-out
3. Segmentation
Segment different areas
At present, scenization and refinement are the major development trends of the data labeling industry. High-quality data sets will effectively improve the accuracy of image recognition and add new vitality to the commercialization of the new retail industry.