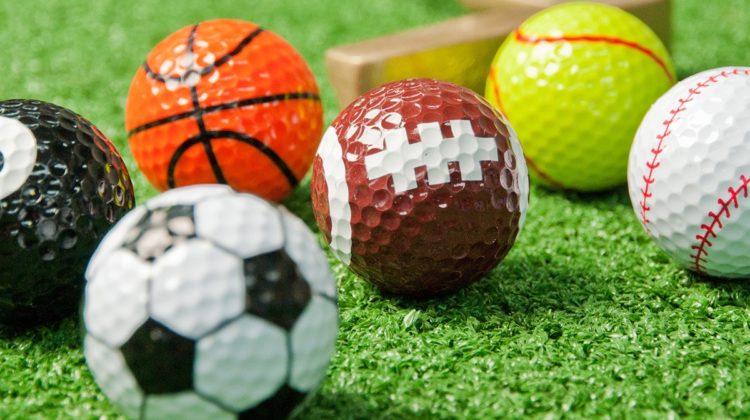
How i built a Sports Ball Classifier in Keras using CNN model…
- Overview
Created a tool that classify Sports Ball in three different category.
Scraped over 1000 Images for Sports ball from Google, Yahoo, Bing, Ecosia using two simple python scripts
Engineered Images to convert those images to data field to store in csv file
Approached for naive based Logistic Regression using LogisticRegression model (scikit-learn)
Approached for neural network based solution using CNN (Convolutional neural network) in keras
Build a client facing A
- Web Scraping
Tweaked and modified the web scraper github repo (“https://github.com/manijhariya/WebBot”) to scrape 1000 images from
Google, Yahoo, Bing, Ecosia search engines with each image we got.. 36 words (from dictionary to look in search engines) * 4 search engines = 1036 Images - Data Cleaning and EDA
After scraping the data, Scripted in python to clean the data so that it can be used by model. Made following changes to clean data.
Converted every image into 3 channels (RGB) form.
Resized every image into 100 * 100 (W * H)
Flatten every image data after taking it into array
Saved image array data in csv file for easy to share - Model Building
Data was ready to fit into the model. Before that i transformed the categorical variables into dummy variables AKA one hot encoded variables
* Using Linear Model
I tried easy Scikit-learn approach with LogisticRegression model with train and test split with 0.2 value
With LogisticRegression model i ended up with 85.2 % accuracy by scoring model *Using Neural Network
Implimented model in Tensorflow2 (keras) in Convolutional Neural Network approach with validation split 0.2 value
Compiled Model with Adam Optimizer with learning rate 0.001, EarlyStopping with CNN model i ended up with 88.4 % accuracy by metrics accuracy - Productionization
In this process, I built a flask API endpoint that was hosted on a local webserver by following along with flask documentation.
The API endpoint takes in a request in the form of an image of type jpeg, jpg, png and returns the predicted type of Sports ball
in the image.
You can find full repository here https://www.github.com/manijhariya/SportsBallClassifier