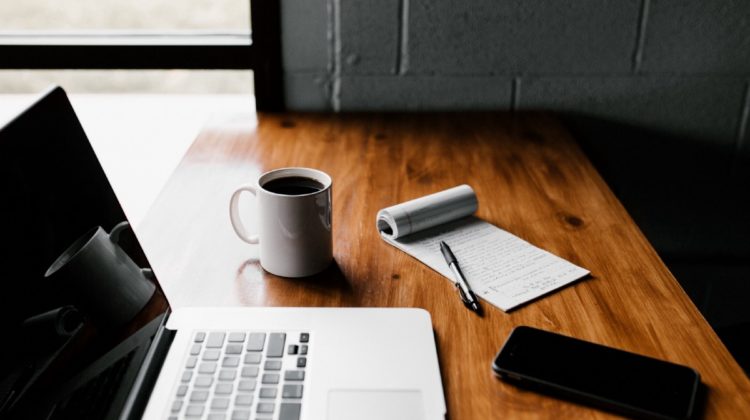

During this marvelous time with the pandemic, many are finding their careers affected. This includes some of the most talented data scientists with which I have ever worked. Having shared my personal experience with some close friends to help them find a new job after being laid off, I thought it worth sharing publicly. After all, this affects more than my friends and me. Any data scientist who was laid off due to the pandemic. Who is actively looking for a data science position can find something to relate to, which I hope will finally offer hope in your job search.
To be forced to start looking for a new job was daunting, to say the least. After browsing the data science job openings on the market, I soon realized my knowledge gap. What I was doing at the B2B startup (a mix of entry-level data engineering and machine learning) was irrelevant to many of the job requirements out there, such as product sense, SQL, stats, and more. I knew the basics but was unsure how to fill the gap towards more advanced skills. However, even that issue seemed secondary to more pressing questions, such as how do I even get an interview? I had a mere 1.5 years of work experience with a startup, and I lacked any statistics or computer science-related degree. More questions soon followed. What if I cannot find a job before I lose my visa status? What if the economy takes a downturn before I can find a new job? Despite my fears, there was little choice. I had to find a new job.
In the face of what felt like an overwhelming task, I needed some information to decide my next steps. After researching, I realized that more than half of the data science positions on the market were product-driven positions (‘product analytics’). The rests were either modeling or data engineering-oriented positions. I also noted that works other than product analytics tended to have higher requirements. For example, most modeling positions required a Ph.D. degree, and engineering positions required a computer science background. Clearly, the conditions for different tracks varied widely, so it followed that preparation for each would differ as well.
With this knowledge in hand, I made an important decision: preparing for all tracks would be overwhelming and most likely less effective. I would need to focus on one. I choose product analytics because, based on my background and experience, there was a higher chance that I could get interviews on this track. Of course, not everyone in data science has my exact knowledge and experience. Below, I have summarized the general requirements for three categories of data science positions at big companies. Understanding this basic breakdown saved me a lot of time, and I trust it will prove beneficial for others looking for a job in data science. However, I will add that for small startups. It’s possible that the interview will be less structured and require more of a mixture of all three.
Product Analytics (~70% on the market)
- Requirements: practical experience launching products; strong business acumen; advanced SQL skills
- Examples: Data Scientist, Analytics at Airbnb; Data Scientist at Lyft; Data Scientist at Facebook; Product Analyst at Google
Modeling (~20% on the market)
- Requirements: knowledge of machine learning (not only how to use it but also the underlying math and theory); strong coding ability
- Examples: Data Scientist, Algorithms at Lyft; Data Scientist, Algorithms at Airbnb; Applied Scientist at Amazon; Research Scientist at Facebook
Data Engineering (~10% on the market)
- Requirements: an end to end data scientists with data engineering skills; knowledge of distributed systems; MapReduce and Spark; practical experience working with Spark; strong coding ability
- Examples: Data Scientist, Foundation at Airbnb; Data Scientist at some startups
In light of my own experience, the rest of this post is strongly tailored towards those preparing for product analytics positions. Come back later to check out my post on preparation for a data engineering position.
The first thing I did once I knew I would be laid off was to apply widely and aggressively to other jobs. I used all the job boards I knew, including Glassdoor, Indeed, and LinkedIn. I also asked everyone I knew for referrals. However, since it was almost the end of the year, I did not receive any responses until January 2019.
Asking for referrals proved to be much more effective than applying by me. Out of about 50 raw applications, I only got 3 interviews, but out of 18 referrals, I got 7 interviews. Overall, it was becoming obvious that I was not considered a strong candidate in this market.
While the structure of interviews was different for each company, there was a general outline that most companies followed:
- A recruiter initial phone call
- 1 or 2 rounds of technical phone screen (TPS) or a take-home assignment
- A 4 ~ 5-hour onsite interview typically includes 3 ~ 4 rounds of technical talks and a behavioral interview with hiring managers
Around half of the companies (4/10) that I’ve interviewed with had a take-home assignment before or instead of a TPS. Take-home assignments consumed a lot of energy. Typically, an 8-hour take-home assignment caused me to need at least half a day to rest after submission. Because of this, I did my best to schedule the interview accordingly. There were no interviews the morning after my take-home assignment. Simply being aware of the basic structure can go a long way in making you feel more at ease and able to cope with the process of finding a new job.
Going into my interviews, every opportunity was critical to me. Although I was aware that some people learn by interviewing, becoming better after many interviews, and typically obtaining offers for the last few companies they interview, I did not feel I could take this approach. When I graduated in 2017, I only received 4 interviews out of 500 raw applications. I was not expecting to get many more in 2019. Thus, my plan was to be fully prepared for each interview I got. I would let no opportunity go to waste.
One benefit of being laid off was that I could study full time for the interview. Each day I structured what I looked, focusing on two or three things per day. No more. From previous interviews, I had learned that a deep understanding allows you to give more thorough answers during interviews. It especially helps to have a depth of knowledge in an interview situation when you tend to be more nervous and anxious than usual. That is not the time when you want to try faking things.
As I describe my own experience, I can’t help thinking of a common misconception I often hear: it’s not possible to gain knowledge on product/experimentation without experience. I can’t entirely agree. I did not have any prior experience in product or A/B testing, but I believed that those skills could be gained by reading, listening, thinking, and summarizing. After all, this is the same way we were taught things in school. Actually, as I get to know more senior data scientists, I continue to learn that this method is standard, even for people with years of experience. What you will be interviewed on may not be related to what you were doing at all, but you can gain the knowledge you need in ways other than job experience.
Here are the basics of what you can expect. Typically, product and SQL questions were asked during a TPS. Onsite interviews included a few rounds of problems, including product sense, SQL, stats, modeling, behavior, and maybe a presentation. The next few subsections summarize the most valuable resources (all freely available) I used when preparing for interviews. In general, GlassDoor was a good source to get a sense of company-specific problems. Once I saw those problems, I understood both what the company needed and where my gaps were in fulfilling those needs. I was then able to develop a plan to fill those gaps.
Photo by Andrew Neel on Unsplash
The following six subsections are how I prepared for the specific content in interviews for the product analytics tracking. In explaining my own preparation, I hope to make the path smoother for those who come after me.
Working as a data scientist at a startup, I was mainly responsible for developing and deploying machine learning models and writing spark jobs. Thus, I barely gained any product knowledge. When I saw some real interview questions on GlassDoor, such as “how to measure success?” or “how to validate the new feature by current users’ behaviors?”, I had utterly no idea how to approach such questions. At the time, they seemed far too abstract and open-ended.
I resorted to the essential read and summarized strategy to learn product sense, using the resources listed below. All this reading helped me build up my product knowledge. As a result, I came up with a structured way (my own ‘framework’) to answer any product questions. I then put my knowledge and framework to the test with that all essential to learning any skill: practice. I wrote out answers to questions involving product sense. I said my answers out loud (even recording myself with my phone) and used the recordings to finetune my solutions. Soon I could not only fake it for an interview. I actually knew my stuff.
Resources:
The first time I took a SQL TPS, I failed, and it was with a company in which I was very interested. Clearly, something needed to change. I needed to, once again, practice, and so I spent time grinding SQL questions. Eventually, I completed in a day questions that had previously taken me an entire week. Practice makes perfect!
Resources:
To prepare for these kinds of questions, I brushed up on elementary statistics and probability and did some coding exercises. While this may seem overwhelming (there is a lot of content for both topics), the interview questions for a product data scientist were never hard. The resources below are a great way to review.
Resources:
Without a CS degree, I went into the job search with limited machine knowledge. I took some courses during my previous job, and I reviewed my notes to prep for interviews. However, even though modeling questions are getting more and more frequent nowadays, the interview questions for a product data scientist mainly geared toward how to apply those models rather than the underlying math and theories. Still, here are some helpful resources to bump up your machine learning skills before the interview time.
Resources:
Some companies required candidates to either present the take-home assignment or the proudest project. Still, other companies asked about the most impactful project during behavioral interviews. However, no matter what the form, the key is to make your presentation interesting and challenging.
That sounds great, but how do you do that? My main recommendation is to think through all the details, such as high-level goals and success metrics to ETL, modeling implementation details, deployment, monitoring, and improvement. The little things add up to make an excellent presentation rather than one big idea. Here are a few questions worth rethinking to help reach your ideal presentation:
- What were the goal and the success metric of the project?
- How do you decide to launch the project?
- How do you know whether customers are benefiting from this project? By how much?
- How do you test it out? How to design your A/B test?
- What was the biggest challenge?
When presenting a project, you want to engage the audience. To make my presentations interesting, I often share interesting findings and the biggest challenges of the project. But the best way to make sure you are engaging in the practice. Practice and practice out loud. I practiced presenting to my family to ensure my grasp of the material and ease of communication. If you can engage the people you know, an interviewer, who is required to listen, doesn’t stand a chance.
While it is easy to get caught up preparing for the technical interview questions, don’t forget that the behavioral problems are equally important. All companies I’ve interviewed with had at least 1 round of behavior interviews during the onsite portions. These questions typically fall into these three categories:
- Why us? / what do you value most in a job?
- Introduce yourself / Why are you leaving your current job?
- The biggest success/failure/challenge in your career. Other versions: Tell me about a time you resolved a conflict, or you’ve had to convince your manager or a PM on something.
Behavioral questions are essential for data scientists. So be prepared! Understanding a company’s mission and core values helps answer questions in the first group. Questions like 2 and 3 can be answered by telling a story — 3 stories were enough to answer all behavioral problems. Make sure you’ve got a few good reports on hand when you walk in for an interview. Like product questions, I practiced a lot by saying it aloud, recording, and listening to fine-tune my answers. Hearing a story is the best way to make sure it works.
Photo by Campaign Creators on Unsplash
The night before an onsite interview was typically a stressful, hectic night. I always tried to cram in more technical knowledge while simultaneously reviewing my statistics notes and thinking of my framework to answer a product question. Of course, as we all learned in school, none of that was beneficial. The results were largely determined due to the amount of preparation before not a single night of cramming. So practice is important, but there are some rules you can follow the day of to make sure your interview is a success.
- Always clarify questions before answering. Ensure that you understand what you are being asked by repeating back the problem in your own words. It’s a red flag if you answer the questions without clarifying them.
- Organize the answer to all questions. Write down your thought process with bullet points. This shows the interviewers that you have a systematic way to approach a problem and help interviewers write a later review.
- Don’t panic when you don’t know the answer. It’s okay if you are not familiar with the domain. In such cases, you could start by making a few assumptions, but make sure to communicate that you are making assumptions and ask whether they are reasonable. Sometimes it’s wonderful to ask for more time. What if you cannot think of any answer and your brain went blank? Talk about an experience you have that is related to the question.
- Attitude matters. Companies are looking for someone who is willing to listen and who can embrace different opinions. You want to show that you are someone with whom it is easy to work. Be humble and respectful. Listen and clarify. Bring your positive energy to the room, and do your best to have a good conversation.
- Research, the company. Could you get familiar with its products? Ask yourself how to improve the products and what metrics can be used to measure those products’ success. It’s also helpful to understand what data scientists do at each company by reading their blogs. Doing this kind of research leads to more profound and ultimately better conversations in interviews.
Using these rules, this was the feedback I got from onsite interviews:
- A very structured way to answer product questions
- The presentation is very organized, well thought out.
- Showed deep interest in our products and offered valuable ideas on improvements
After receiving verbal offers, the next step was to work with recruiters to finalize the numbers. There’s only one rule here that I stick with — ALWAYS negotiate. But how?
Haseeb Qureshi has a beneficial guide on negotiating a job offer (with scripts!), which I followed religiously during my offer negotiation phase. Every single rule was so true. I dealt with all companies that gave me an offer. The average increase for offers was 15%, and the highest bid was, in total value, increased by 25%. Negotiating works, so don’t be afraid to try it!
- LOTS of practice is the key.
- Failure is part of life and a part of job searching. Don’t take it too seriously.
- Find a way to destress that works for you.
After losing 11 pounds and lots of cries and screaming (job hunting is stressful, and it is okay to admit that), I finally got 4 offers within 2 months of being laid off. 3 of those offers were from companies that I have never dreamed of joining: Twitter, Lyft, and Airbnb (where I ultimately joined) and another offer from a healthcare startup. By the end of two frenzied months, I had received a total of 10 interviews, 4 onsite interviews, and 4 job offers, giving me a 40% TPS-to-onsite rate and 100% onsite-to-offer rate.
Image by Emma Ding | Timeline from being laid off to joining my dream company
I was so lucky that I got lots of support and help from family and friends after being laid off, which was critical to landing a job at my dream company. It wasn’t easy. Ironically looking for a job is also a lot of work, but everything was worth it.
There is so much to prepare for interviews. I hope this post has made things clearer for other data specialists out there in need of work, and if you want more advice feel free to contact me here. I am grateful to be working in a great job, and I would be happy to help you get there too!
So I decided to make a series of videos to help you land your dream data science job. Check my YouTube channel if you are interested!
Data Interview Pro
WELCOME! I’m EMMA DING. I’m a Data Scientist/Data Engineer at Airbnb, and when I’m not busy… I make videos and write…
Bio: Emma Ding is a Data Scientist & Software Engineer at Airbnb.
Thanks for reading…