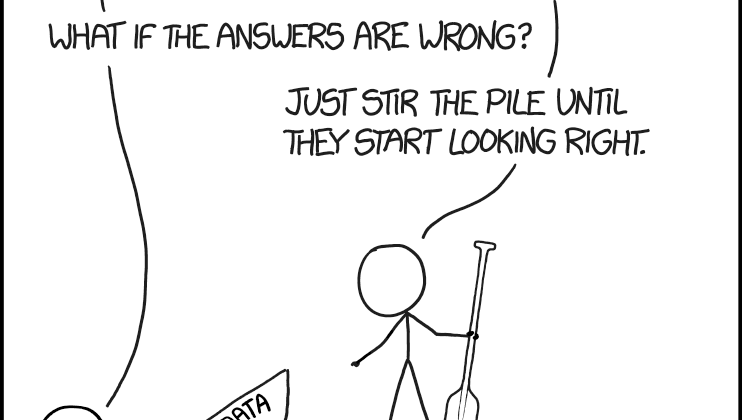
5 minutes for 5 hours’ worth of reading

I saw the image above in a blog post by Sandeep Uttamchandani about examples of what can go wrong in a machine learning project. People often read these articles as funny anecdotes that can never happen to them. Machine learning projects usually require significant investment (talent, time, and technology). Accordingly, it would be a mistake to think that the probability of making a mistake is more important than what would be its consequences.
Also, I subscribed to yet another newsletter — Machine Learning Ops Roundup. Check it out if MLOps is of interest to you.
But now, let’s get into the reading list!
- The Unicorn in the Attic: I’m a strong believer of value of data and analytics for business. The article, written be the director of data and analytics of JLR, is reasoning that even traditional businesses — rooted in physical production — are existentially dependent on considering information and analytics their core product. This is a strong message by itself. The article certainly whetted my appetite, and I can’t wait for practical examples of how JLR’s data and analytics department made around £100 million in incremental profit. Hats off to Harry for sharing his and his team’s story. Now we can either nit-pick the numbers or get inspired by a data story written in automotive. (Harry Powell)
- Looking ahead to the future of computing and data infrastructure: Another outlook for the future trends in data infrastructure. But this time from Kleiner Perkins, which gives it a bit more weight. Many trends are elaborated, three resonated with me the most: (1) building applications directly on top of the data warehouses; (2) business processes written as code; and (3) consolidation of the ML infrastructure space. The second one, in particular, will be interesting to see as it will require a massive culture change in many organisations. (Kleiner Perkins)
- Real-time Machine Learning For Recommendations: Many millions of companies use e-commerce; the most prominent ones are known for intelligent recommendation systems. I like reading Eugene’s articles because they combine high-level ideas, with low-level technical details. This one quickly describes a modern architecture of recommendation systems, when it is beneficial to recommend in real-time, what are the consequences of doing so and also reviews real-life examples — from MVP to industry examples. (Eugene Yan)
- Breakthroughs in Time Series Forecasting at Neurips 2020: Flow forecast repository is an open-source deep learning for time series library. Time series forecasting is an old and important task. Because of the importance there is a lot of research in the field. The work the community around the library is doing is imho amazing for two reasons: (1) they are constantly monitoring the latest advancement in the field; and (2) they are implementing it so that it’s easy to use for the rest of us. For (1) read the blog post. For (2) check out the library. (Isaac Godfried @ Towards Data Science)
It’s been three months since I started these weekly reading blog posts. Not a big anniversary yet but it’s definitely becoming a habit.