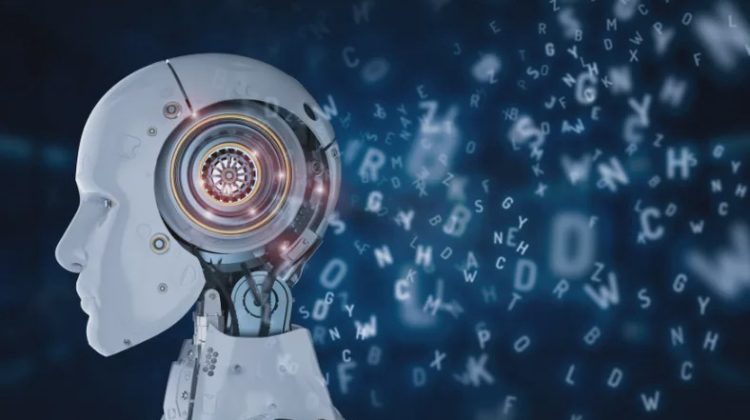

AI-DRIVEN ROBOTIC INTELLIGENCE
Historically, mobile manipulators were teleoperated, controlled by an operator from a standoff distance. Today, thanks to enhancements in artificial intelligence (AI) and machine learning, mobile manipulators are on the path to becoming fully autonomous and enhanced with the ability to not only see, but to think and react like humans.
When combined with AI, today’s mobile manipulators have the capacity to revolutionize business operations. AI-infused manipulators have the ability to “course correct,” allowing them to learn, over time, which methods and processes are the most effective. This capability can therefore lead to better efficiency and a higher degree of reliability than what their human counterparts can typically offer.
Using data collected from RE2 Detect, RE2’s autonomy algorithms, known as RE2 Intellect, fuse geometric computer vision with traditional machine learning and deep learning techniques to provide human-like decision processing. Unlike traditional autonomy algorithms, which are based on a single method that can only work in structured environments with controlled lighting, RE2 Intellect can handle anomalies typically experienced in unstructured, outdoor environments with variable lighting — similar to the way human beings perceive and process information. In other words, our algorithms can think in any environment.
In addition to thinking, systems must then be able to act. The complete process is often described as the OODA Loop: observe, orient, decide, act. RE2 Detect is responsible for observing and orienting, while RE2 Intellect decides and acts. In humans, the brain is what closes this loop and determines the action based on perception. RE2 Intellect is the brain of RE2’s intelligent mobile manipulators.
How Do Robots Learn?
Teaching a machine to learn involves data, features and algorithms working together to build a robot’s “intelligence.” The more diverse the data, the better the results will be. Likewise, features, which are considered to be the building blocks of data, provide the algorithms with the characteristics or properties needed to perform a given calculation. Finally, choosing the correct algorithm for your data and features is critical. Algorithms can include supervised methods like classification and regression, or unsupervised methods like clustering or patterns search. The selected method directly affects the precision, performance and size of the final model.