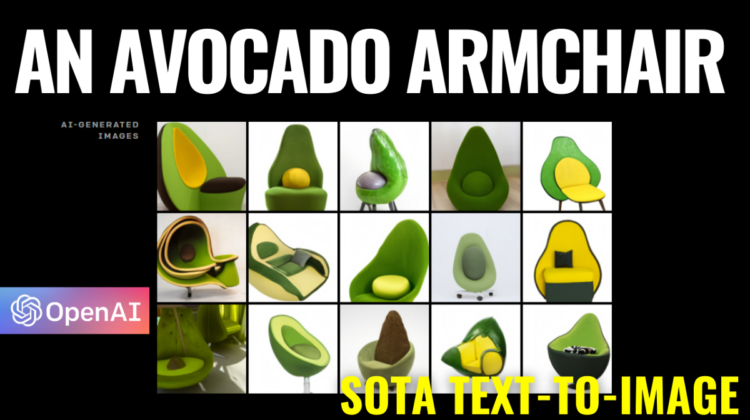
OpenAI just released the paper explaining how DALL-E works! It is called “Zero-Shot Text-to-Image Generation”.
It uses a transformer architecture to generate images from a text and base image sent as input to the network. But it doesn’t simply take the image, the text, and sends it to the network. First, in order to be “understood” by the transformer architecture, the information needs to be modeled into a single stream of data. This is because using the pixels of the images directly would require way too much memory for high-resolution images.
Instead, they use a discrete variational autoencoder called dVAE that takes the input image and transforms it into a 32 x 32 grid, giving as a result 1024 image tokens rather than millions of tokens for a high-resolution image. Indeed, the only task of this dVAE network is to reduce the memory footprint of the transformer by generating a new version of the image. Of course, this has some drawbacks, while it saves the most important features, it also sometimes loses fine-grain details, making it impossible to use for fine-grain applications that are based on very precise characteristics of the images. You can see it as a kind of image compressing step. The encoder and decoder in the dVAE are composed of classic convolutions and ResNet architectures with skip connections.
If you never heard of variational autoencoders before, I strongly recommend you to watch the video I made explaining them.
And this dVAE network was also shared in OpenAI’s GitHub, with a notebook to try it yourself, and implementation details in the paper, the links are in the references below!
These image tokens produced by the discrete VAE model are then sent with the text as inputs to the transformer model. Again, as I described in my previous video about Dall-E, this transformer is a 12-billion parameter sparse transformer model.
Without diving too much into the transformer’s architecture, as I already covered in previous videos, they are a sequence-to-sequence model that often uses encoders and decoders.
In this case, it only uses a decoder since it takes the generated image by the dVAE and the text as inputs. Each of the 1024 image tokens that were generated by the discrete VAE has access to all text tokens and using self-attention, it can predict an optimal image-text pairing.