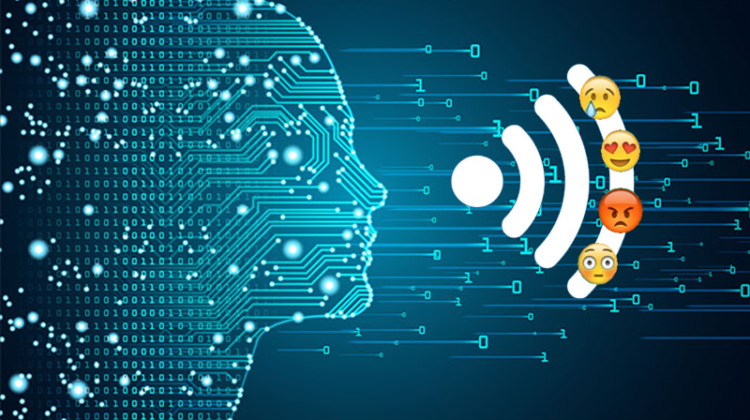
DEEP LEARNING
Scientists have proposed a novel & non-invasive AI approach to detect emotions using wireless signals

If there is any doubt in your mind that are not headed to a future where mind-machine meld is going to be the new norm, just look at Elon Musk’s Neuralink’s BCI. The animal trials are already underway, as claimed by Musk, a monkey with a wireless implant in his skull with tiny wires can play video games with his mind. Although designed to cure a wide variety of diseases, the experiment aligns with Musk’s long-term vision of coming up with a brain-computer interface that is able to compete with increasingly powerful AIs.
However, Neuralink’s proposed device is an invasive one that requires fine threads that need to be implanted in the brain. And as if these invasive devices were not scary enough for a person like me, new breakthroughs in neuroscience and artificial intelligence might infiltrate our emotions — the last bastion of personal privacy. Don’t get me wrong, I am all for using the novel tech for healthcare purposes, but who is to say that this can’t be used by nefarious players for mind control or “thought policing” by the State.
Scientists at the Queen Mary University of London have explored a novel Deep Learning technique to measure heart rate & breathing signals by using radio waves to predict how someone is feeling — in the absence of any other visual expressions. The process involved the application of a deep neural network that can determine a person’s emotional state by analyzing the above-mentioned wireless signals.
In the human trials, the participants were asked to watch a video that is supposed to induce one of the four basic emotion types — anger, sadness, joy and pleasure. While watching the video, scientists sent harmless radio signals towards the participants and let the algorithm study them when they bounce back off them to determine what kind of emotion they were exhibiting. The wireless signals used are similar to the ones used by radar or WiFi.
“Being able to detect emotions using wireless systems is a topic of increasing interest for researchers as it offers an alternative to bulky sensors and could be directly applicable in future ‘smart’ home and building environments.”
~ Ahsan Noor Khan, First Author of the Study
Changes in the bounced back signals enabled the team to determine an individual’s heart and breathing rates. Although researchers have previously used AI techniques previously to carry out such an experiment, data analysis has depended on the use of classical machine learning approaches — whereby the algorithm only classifies emotional states within the data.
In a departure from that technique, scientists used deep learning techniques, where the AI model learns from the raw data it collects—thus showing that this approach could detect emotions more accurately than traditional machine learning methods. For the purpose of this study, the deep learning model accurately measured emotions in a subject-independent way.
This is also a huge improvement from traditional emotion detection, which relies heavily on the assessment of visual indicators — such as facial expressions, speech, body gestures, or eye movements. This, however, only provides an external assessment of physical movements and not necessarily reflect the internal human emotions.
Researchers have also been looking at other ‘invisible’ signals, such as ECG to understand emotions. ECG basically measures the electrical activity in the heart. But once again, these are also measured using invasive sensors that are placed on the body. This study is a significant step towards the non-invasive approach that scientists have been looking for.
The scientists believe this research could help with the wider management of health and wellbeing apart from detecting psychological conditions like depressive states. Although the research has shown promise for commercial-scale deployment, scientists believe they would need to ponder over social and ethical concerns, including data protection.
Complete Research was published in the Journal PLOS ONE.