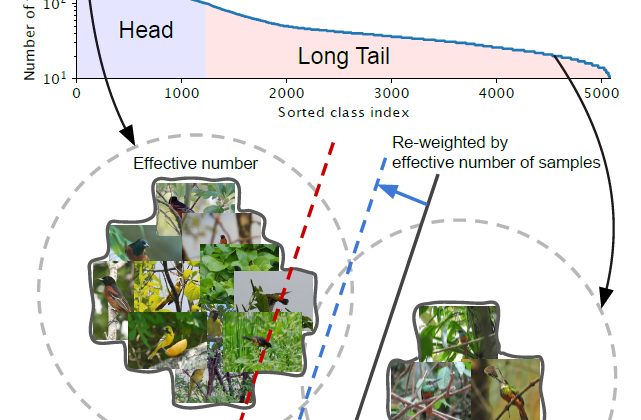
Using the Effective Number of Samples for Each Class to Re-Balance the Loss, Outperforms Focal Loss in RetinaNet

In this paper, Class-Balanced Loss Based on Effective Number of Samples, (CB Loss), by Cornell University, Cornell Tech, Google Brain, and Alphabet Inc., is reviewed. In this paper:
- A re-weighting scheme is designed that uses the effective number of samples for each class to re-balance the loss, called class-balanced loss.
This is a paper in 2019 CVPR over 200 citations. (Sik-Ho Tsang @ Medium)