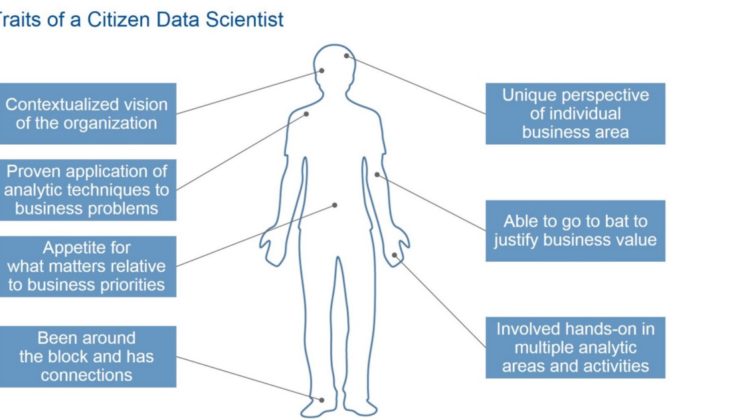
Not everybody wants to do courses, learn programming languages to become Data Scientist. So can you be a Citizen Data Scientist?

There is a growing consensus that organizations can leverage internal skills to bring the basic data science expertise in-house for advanced analytics while minimizing the burden on organizational resources. Anybody with an aptitude of problem-solving and some know-how of data, can be a citizen data scientist today.
Although the term has existed for a couple of years now, you won’t find job listings for “citizen data scientist” on Glassdoor.com. That’s because it’s not a role that an organization is going to hire for, but more like a requirement, they need to fill. People in the industry are using the term citizen, but those doing the hiring are focused on the tasks not currently getting done but require attention.
If it’s not a job title getting posted, what exactly is it? Gartner defines a citizen data scientist as “a person who creates or generates models that leverage predictive or prescriptive analytics, but whose primary job function is outside of the field of statistics and analytics.” They bridge the gap between those doing self-service analytics as business users and those doing advanced analytics as data scientists. InformationWeek states that the “defining trait is that statistics and analytics are secondary in the role.”
Analytics leaders like protonAutoML are working to build out tools to support the citizen data scientist. They are creating machine learning tools, data visualization tools, where data streams can be added, connected and analyzed via drop and drag methods. These tools are powered by an underlying analytics engine, so it is easier for the citizen data scientist to create more “ah ha” moments using data, algorithms and models.
Whether or not citizen data scientists are used, a company working to build advanced analytic capabilities also needs subject matter experts (SMEs) to provide industry/process-specific context for what the patterns identified by the algorithms and models actually mean. If these SME are given these tool, it can be a perfect combination.
It’s common knowledge that we have a shortage of data scientists, and businesses realize that not every job function needs to be done by someone with an advanced degree as they reckon with this shortage. Instead, a citizen data scientist can be trained to do work that is needed but not currently getting done.
For example, Big Data influencer Bernard Marr describes how Sears recognized a need for people who were more than average Excel users, but not as highly trained as data scientists to improve their customer segmentation. To meet this need, they reskilled existing staff into Big Data analysts to enable the retailer to make more informed decisions about products shown to website users. Doing so reduced data preparation costs by hundreds of thousands of dollars, as the so-called citizen data scientists handled exploratory analysis, visualization, and putting their insights into action.
By reskilling or upskilling employees to take on these enhanced data roles, organizations can make better use of data, which leads to both cost savings through greater efficiency and competitiveness through access and use of that data. Gartner predicts citizen data scientists will be doing more advanced data analysis than the better-educated data scientist as soon as 2020.
Data analytics professionals possess the deep domain expertise to recognize the core business challenges of their department and a thorough understanding of the available data but often lack the ability to perform some of the data science tasks that distinguish an analyst from a citizen data scientist. protonAutoML’s automated machine learning software replicates the tasks and processes that up to now have been manually performed by data science Ph.D.s, allowing users to implement machine learning solutions without writing code or preselecting algorithms.
Citizen data scientists can upload a dataset to protonAutoML and pick a target variable based on the practical business problem they wish to solve. The platform automatically applies best practices for data preparation and preprocessing feature engineering, and model training and validation. protonAutoML selects the most appropriate algorithms for the data and target variable and ranks trained models according to their accuracy so that citizen data scientists can easily interpret and select the most appropriate model for the business problem. protonAutoML automatically uncovers insights that users might not have noticed and keeps guardrails in place so that users can trust the output of their models.
About Author: Harsh Gupta has more than 7 years of experience building and directing AI initiatives across diverse industries, amounting to $10M + additional revenue during this period. He has served in technical roles such as Data Scientist at WWF and client-facing roles such as Consultant for Johns Hopkins, Grofers, OSUgiving. He is currently CEO of protonAutoML, a full-service data science consultancy and autoML software provider.
He can be reached out here for any advice or consultation.