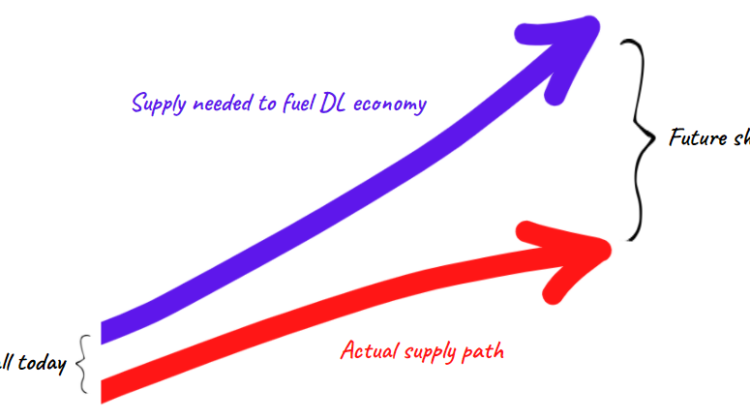

The promise of business transformation from Deep Learning is at risk due to widespread inefficiency in GPU utilization.
Artificial Intelligence, Machine Learning … anyone reading this has probably heard these terms. At minimum, you get the basic concept — smarter machines.
Machine Intelligence will change everything
What you might not realize is the degree to which machine intelligence is transforming the business landscape across every industry to the point where it could be barely recognizable in 15 years. Collisions between AI-driven and traditional firms are already happening across industries — if you’re not at least making a game plan, you could soon find yourself on the sidelines.
But the positive side of this massive disruption is that it carries the promise of creating unprecedented value, “enlarging the pie” in ways we’ve never seen. Leaving aside, for now, how critical it will be to elegantly manage the socioeconomic implications of this new world — it is crystal clear that it is coming, it is The Next Big Thing, and it Will Change Everything.
ARK Invest, an investment management firm focused on innovation, predicts that Deep Learning — the “smartest” cutting edge of machine learning — will create $30 trillion in value by 2037. It’s worth taking a moment to let this number sink in — DL will create more value than the Internet and all other Information Technologies have ever created.
Processing capacity is critical to DL
To fuel this vision, ARK says the average cost of training a DL model will grow from $1 million to $100 million per project, and a majority of that expense will be for the processing hardware to do the heavy lifting — expanding spending more than 4x during the next five years, from $5 billion a year today to $22 billion in 2025.
The processing hardware driving DL today is a specific chip design called a GPU.
A quick overview of GPUs
While the CPU (Central Processing Unit) in a computer is like a Swiss Army knife, happily switching between different kinds of tasks, a GPU is an altogether different chip design that’s more like a vastly parallel array of machetes — it’s only good for certain kinds of tasks, but it handles them very fast and very well.
GPUs (Graphics Processing Units) emerged because the sheer volume of parallel calculations necessary to process and display increasingly complex computer graphics (e.g. smoother and more lifelike video games) was bogging CPUs down — it’s hard to pull out the corkscrew to open that bottle of wine when you have to keep cutting open coconuts with that tiny blade.
Beyond “Graphics”
The “G” in GPU has become a bit of a misnomer. It turns out that GPUs are also great at handling all sorts of High Performance Computing (HPC) behind emerging tech like Virtual/Augmented Reality, the Edge Acceleration behind the Internet of Things, and most significantly, Deep Learning — driving both the rise of NVIDIA (top maker of GPUs and already the 18th largest company in the world) and 34% annual growth prediction in the overall GPU market over at least the next five years.
Adding lofty expectations of a new DL-driven economy to an already hot market, it’s clear that demand for GPUs will continue to increase steeply — but let’s take a look at where all this awesome hardware is supposed to come from.
Supply growth of GPU capacity is far from assured
Moore’s Law is officially dead — each advance in chip design is taking more time and exponentially more capital, and the cost per performance gain of each new generation is not heading in the right direction. Brutal economies of scale have led to a staggering narrowing of the field of cutting-edge chip foundries (the actual factories where the chips are physically produced) from over 25 manufacturers in 2000 to only two — TSMC (the 10th largest company in the world) and Samsung (the 12th largest).
As I write this, a chip shortage is shuttering auto factories — that extreme supplier consolidation, the pandemic, and extreme weather events all playing a role in supply chain disruption. Competition is already fierce for chips, and one need only pay attention to the news to imagine that such disruptions will become increasingly common.
The impending shortfall
So on one hand we have predictions of steep increases in demand for GPU. On the other hand we have asymptotic laws-of-physics limitations and the economic (not to mention geopolitical) realities of a very small number of suppliers running a handful of factories. GPU is already a premium resource that can bottleneck companies’ efforts to build business value, and our expectations of it are rising quickly — seemingly faster than we can build it.
It looks like the next big step for the global economy is at genuine risk of failing to launch, or at least of being significantly dragged down by lack of this precious fundamental resource. What is to be done? We might look to history for some guidance — namely the oil crises of the 1970s, where a shortfall of another precious fundamental resource forced us to focus on improvements in efficiency.
GPU utilization is very inefficient
Hard numbers are difficult to come by — Googling things like “estimated percentage of worldwide GPU utilization idle” is surprisingly disappointing — but one look at GPU utilization for DL suggests it’s well below 50% during DL training runs, not to mention the fact that GPUs are doing nothing when no one is using them.
Qualitatively, it makes logical sense that today’s usage of GPU is broadly inefficient. The main problem is that CPU-based DL applications are tightly coupled with the GPUs that power them. The GPU in any given machine has to be able to handle peak workloads from its CPU-application space in order to keep things running smoothly. But peak load is, by definition, higher than normal — so when operated below peak, the GPU is underutilized. Workloads are usually very spiky, with intermittent high utilization for any given job punctuating longer periods of much lower utilization.
An ideal “perfect utilization” would keep every GPU on the planet constantly pinned at 100%. My wild (only in the sense that the error bar is high — the number is probably conservative) guess is that average actual utilization is below 40%.
We can do way more with the GPU capacity we already have
GPU is a precious resource that’s being wasted — markets hate that — and its processing power will only come into higher demand as the new world of Deep Learning becomes a reality. Wasteful inefficiency welcomes a disruptive step-change, and (like for so many things) software can provide it.
In my next post, I’ll explore some of the reasons why GPU utilization is so inefficient, and a model for improvement through innovative software.
Steve Golik is co-founder of Juice Labs, a startup with a vision to make computing power flow as easily as electricity.