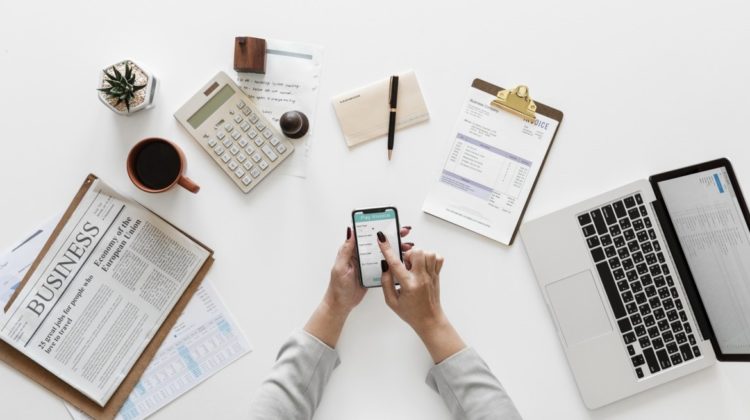

It is widely expected that A.I. adoption will accelerate in firms post covid[1, 2, 3, 4]. Similarly, a report from McKinsey states that “companies plan to invest even more in AI in response to the COVID-19 pandemic”[5]. In this article, I would highlight three reasons for this growth, and importantly what we can do to prepare for this growth.
The way we work has changed over the past year. At our workplaces, physical face to face meetings and physical events have been replaced by virtual meetings and webinars. E-documents and e-signatures have replaced physical documents and signatures. These point to the apparent that the pandemic has forced the digitization of processes in workplaces wherever possible.
Hence today, instead of just meeting minutes that are digitized, entire meetings consisting of audio discussions, typed conversations and video are now digitized. Every document that requires the collaboration of two or more executives is now shared either over email or the cloud. In a bid to reach customers virtually, firms are placing product information online and customers are making these buying decisions on digital platforms.
The net effect will be an increase in digital data for businesses. Data, which has been touted as the new oil[6,7,8] is one of the key foundations A.I. technologies require to work. Reason being one of the key techniques of A.I. today is one known as machine learning. It is so because machine learning techniques relies heavily on data.
Machine learning techniques typically has a phase known as a “training phase”. During the training phase, A.I. engineers would use (1) the data and (2) the expected predictions from this data to generate a set of rules (also commonly referred to as a Machine Learning “Model”). The assumption is once you have generated this model, you are able to feed this model new data and to generate future predictions. Hence it is common to have the belief that the more data you provide to these machine learning techniques in the training phase, the better your model will be. However, the performance of these classical machine learning algorithms (such as decision trees, logistic regression) typically plateaus at a certain point, with additional data having little effect.
Since the early 2010s, A.I. researchers found one particular machine learning technique (deep learning) that seems to defy this plateau. Renowned researcher Andrew Ng has claimed that for deep learning techniques, you would be able to obtain better performance when the amount of data increases[9]. The improvement in performance is so apparent that just in the span of a few years, deep learning techniques have been adopted in many verticals.
As we are seeing the convergence of both data growth and deep learning, we start to create a “Virtuous circle of AI” positive-feedback loop where data created improves the deep learning models, which attracts more users and thereby creating more data[9]. This feedback loop creates defensible business models and builds resilience in our firms.
In the face of such upending changes, studies have shown that business leaders believe that only a quarter of their workforces are prepared for A.I. adoption[10]. Such changes are often met with confusion, skepticism and even mistrust. On the other hand, there is also the real risk of having such A.I. models misperform[11].
Certainly, a skilled workforce with a sufficient knowledge of A.I. will be able to alleviate some of these risks. Employers would do well to focus part of their efforts on employee re-training. However we recognize that knowledge only brings us quite so far. Ultimately all organizational changes are the result of staff performing the required changes themselves.
As employers we must make the best efforts to see that such changes not only provide value to the firm, but also alleviates the pains of employees. This will help obtain buy-in from staff and their commitment to see these changes through. As employees as we are carrying out the changes, we have to keep an open mind and must be ready to feel uncomfortable, challenged but indispensable as both the employer and employee work hand in hand to confront the bumpy recovery road ahead of us.
Note: this article inspired from a masterclass I gave in December 2020. You can find the presentation here: https://masterclass.sg/2-dec-introduction-to-deep-learning/
Dr Donny Soh is an Assistant Professor from the Infocomm Technology Cluster (ICT) at Singapore Institute of Technology (SIT). He holds a PhD from Imperial College, London.
[2] https://www.bcg.com/publications/2020/business-applications-artificial-intelligence-post-covid
[3] https://www.defenseone.com/ideas/2020/10/post-covid-world-we-need-ai-more-ever/169531/
[4] https://www.xero.com/sg/the-next-chapter/?escape=true
[7] https://www.wired.com/insights/2014/07/data-new-oil-digital-economy/
[8] https://www.kenwayconsulting.com/blog/data-is-the-new-oil/
[9] Andrew Ng. (2018). Machine Learning Yearning. Draft https://www.deeplearning.ai/content/uploads/2018/09/Ng-MLY01-12.pdf
[10] https://sloanreview.mit.edu/article/getting-your-employees-ready-for-work-in-the-age-of-ai/