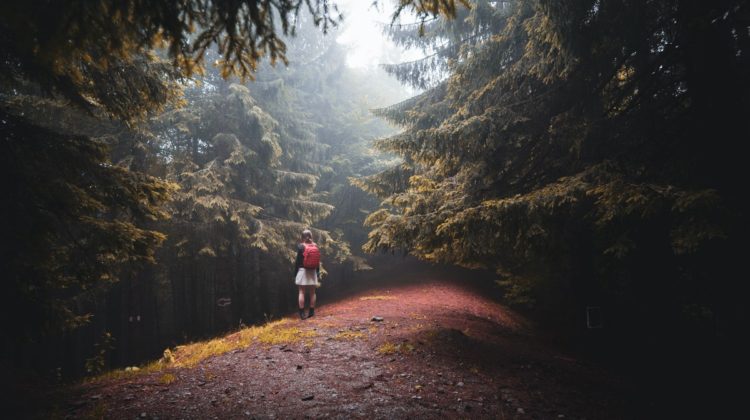
- Introduction
- Traditional Graduate Master’s Degree
- Certifications
- Bootcamps
- YouTube
- Online Tutorials
- Summary
- References
With the emergence of new technologies, competition, and world events, the path to become a Data Scientist has varied greatly. While there are the tried-and-true traditional ways to become a Data Scientist — that might not be your preferred way. In addition to shorter educational experiences, there are even more expedited processes you can take to start and keep up with your Data Science path. Lastly, there are nearly free resources out there for many to access. I will be discussing the top five paths to become a Data Science along with a discussion on how, and why it might be the best or worse option for you. Perhaps, you can combine several or all paths. Ultimately, it will be up to you to decide on how your Data Science learning journey plays out. That being said, it is critical to be aware of all of your options — and that is what I will be discussing below.
I am not writing about undergraduate degrees in Data Science specifically as I have not actually pursued one, however, I have completed a graduate degree in Data Science. I do think that some of the points I will discuss for graduate school can also be applied to undergraduate schooling. I attended Southern Methodist University (SMU), and I am glad I did. While expensive, almost all the material I learned is applicable to real-world Data Science use cases. There were times, however, where I wished that I had studied Software Engineering or Statistics in undergrad in order to be more well-equipped, but as we will see below, there are other ways to learn additional, yet specific facets of Data Science.
Here is a pretty comprehensive list [3] of the top Data Science graduate programs, this list actually helped me to decide which programs to apply to — Best 23 Schools with Data Science Master’s Programs:
Benefits of completing a Data Science Gradaute Degree:
- specialized to Data Science — not like undergrad that might include extra classes that are not useful
- easier to start a graduate problem when you are older
- collaborated with students who were already Data Scientists or aspiring ones that had established careers in a similar field
- which helped to build career connections, and to learn from them
- I personally chose a school with my state so that companies would be familiar with that school
- a lot of the times, GRE’s are not required
- since these are specific programs, you can receive financial aid
- graduate schools can be in-person or remote
- graduate schools often add once in a lifetime experiences like:
- conferences, workshops, and retreat-like experiences that allow you to become immersed in Data Science — learning from leaders in the industry
- some schools can be more reputable, which sometimes can lead to more job prospects
- some programs can be completed in a year
- some companies prefer grad degrees (this requirement is changing)
- having a sense of school pride and building long-lasting connections
Overall, Data Science learned from a more traditional graduate program can ensure landing a respectable career in Data Science. Of course, there are cons, like the price and lack of convenience. However, if that is the case in your situation, then you might want to start to consider other programs.
Here are some of the cons you can expect in a Data Science Graduate Degree program:
- price, some range $60,000+
- can take a long time to complete
- can be inconvenient — in-person, for example
As you can see, there are several more pros that I can point out compared to cons. This is my personal experience, so this experience may differ from yours. That being said, take my pros and cons with a grain of salt, and consider other options, like the ones below.
If you want a more tailored program covering specific courses and topics, then certifications are a great way to learn and become a successful Data Scientist. Similarly, if you are unsure of which type of Data Scientist you want to be, then this path is also a better alternative. For example, you may want to be a Computer Vision Engineer, Natural Language Processing Engineer (NLP), Machine Learning Engineer, Data Engineer, or Research Scientist. It can be difficult to know what type of Data Scientist you want to be when you are enrolled in a longer program, like a Master’s degree, where you only have one course in a specific topic — like NLP. Imagine paying thousands for a degree where you only learned about 6 hours of a specific topic on Data Science — this situation is where certifications shine; you can take countless certifications in the same topic, but cover different material within that topic or have different examples to learn from.
Benefits of completing Certifications in Data Science:
- convenient
- cheaper alternative
- many can be completed in your own time
- some certifications are from bigger, fortune 500 companies that, if you complete, it will, of course, help you when applying to that specific company
- you can supplement certifications with whatever current job you have
- so you do not have to sacrifice your normal salary
- sometimes companies that you work for will pay for these certifications as they are not as expensive as a graduate degree and can be tailored for the specific project you are working on at work
- along those lines, certifications can help you at your current job
- you can show your certificates online and highlight them on your resume
- counting as almost real-world experience, since these certifications are hyperfocused on specific problems and use cases (usually)
- certifications are often ranked and reviewed, more so than a university is, especially since perhaps only 100 people would be enrolled in a degree program, while there can be hundreds of thousands of students taking a Data Science certificate program, which can help to inform you on making the best decision
There are several benefits of completing certifications, whether that be the convenience, the cheaper price, or the ability to complete them in your own time. It could almost be argued that certifications, in general, are not as reputable, however, I believe there is a shift in education — people do not want large student loans, they do not want to spend years in a program that is not tailored, and they want a program that is flexible. Therefore, more and more people are enrolling in certifications and showing those off, creating a more reputable view and perspective on people solely applying to positions without traditional, formal education.
Cons of completing Certifications in Data Science:
- sometimes will not be seen by employers as enough experience
- might be created by non-dependable sources
Above, you can see that I have struggled to list some cons for certifications as a learning path for Data Science as well. It is also important to note that there is more variance in certification quality, which usually, most graduate degrees tend to be more stable and distinguished.
At first, it may seem that there is no difference in a bootcamp or group of certifications; however, there are some important ones to note still. One of the main differences of a bootcamp is having a scheduled or ordered learning path. Additionally, you can expect to, sometimes in person, work, study, and collaborate with classmates within that same bootcamp program. I find this point to be beneficial, as you are all working to achieve the same goal, where certifications can sometimes tend to be more individually focused.
Benefits of completing Bootcamps in Data Science:
- cheaper
- faster
- more convenient
- tend to be ordered or in a specific learning schedule
- some offer tuition reimbursement, or if you do not land a Data Science job within 6 months, you do not have to pay tuition
- in-person and remote
- sense of comradery, learning, and collaboration between others
- resembling a degree program but shorter and expedited
- can also complete these bootcamps after work — your current job
- include capstone programs
- reputable instructors and future companies to work with
One bootcamp, in particular, has always stood out to me, while I have not enrolled it personally, I have learned about their program process and have had several friends and a coworker who has completed bootcamps with them — they have gone on to have incredibly successful Data Science careers as well. This bootcamp is named General Assembly [6]:
Cons of completing Bootcamps in Data Science:
- now that bootcamps have become more popular, I have started to see some become more expensive as well
- some are not as well known sometimes
So, out of the first three paths to becoming a Data Scientist, which one will you pursue? So far, graduate degrees can be summarized as more reputable and informative, certifications have the most flexibility, and the cheapest thus far, while bootcamps have a similar structure to a graduate degrees but much faster and usually cheaper.
Now, we enter the space of more unique, and considerably less traditional paths. This fourth path of becoming a Data Scientist is free and has so many hours of Data Science content, perhaps millions of hours. The big con is that it is up to you to decide which videos to trust. A good way of doing that is by looking at the like to disklike ratio of the video, as well as general sentiment in comments. Sometimes, it can be messy, and less structured than the paths mentioned and discussed above, which, depending on the person, might not be worth the trouble. If you are completely new to Data Science, you would be trusting yourself completely to pick videos that have quality — which is another thing, sometimes, quality and correctness can be lacking. So here we have already introduced a lot of cons, but what are the pros, why would we want to learn Data Science this way?
Benefits of learning Data Science from YouTube:
- free
- convenient
- variety of content
- lots of straightforward examples of use cases, sometimes when we learn theory and are not shown an example, it can be stressful and confusing
- visual learning might be more beneficial, depending on the person
- videos can be more entertaining, which can sometimes lead you to learn more — if you enjoy what you are doing
- videos can have the most up-to-date information in Data Science —
for example, a graduate degree might be using a syllabus from a few years ago and not have any information on XGBoost, even though you spend thousands to learn on the older algorithms
- tailored to you by simply searching a very specific example
See, there are pros, and as the years pass, online videos like these are becoming more and more popular, more reputable, and full of even more content.
Cons of learning Data Science from YouTube:
- you might be spending hours learning something that is incorrect — how would you know something is correct or not if you are trying to learn it and don’t currently know it?
- disorganized videos
- wasting time
- don’t know where to start oftentimes
While there are more cons in this path to Data Science, it may be the future, and it is beneficial to start learning from this source because you have the free freedom to choose your Data Science topics, all the while being entertained.
What is different from online tutorials and YouTube you may ask? Well, when I refer to online tutorials, I mean the countless examples of GitHub, Python code, and Jupyter Notebooks, as well as articles like these posted online every second. So many of these have end-to-end code as well where you can simply plug in your data and see baseline results quickly. Sometimes, this amount of information can be overwhelming, and incorrect, so this is a reason to take this last path with a grain of salt. It is important to practice real-world use cases, and a lot of online sites have tutorials that cover anything from data ingestion, exploratory data analysis, business metric isolation, feature engineering, model building — Machine Learning algorithm comparisons, results, and discussions along with their respective visualizations.
Benefits of learning Data Science from Online Tutorials:
- free
- easy-to-use
- can do it on your own time
- can find specific examples that pertain to your questions in Data Science
- from people who are also learning, and not from seasoned teachers who might forget to include the basics of Machine Learning fundamentals
- GitHub has countless examples
- there are easy-to-understand Jupyter Notebooks out there with examples and explanations
- competitions out there also include common business problems and those might relate to yours
There are several benefits when choosing the online tutorial path, and I personally, use this path the most in my day-to-day work. There are places like Stack Overflow, GitHub, and Kaggle that house different examples and explanations, which sometimes can be more straightforward and considerably more beneficial than a degree — and free.
Cons of learning Data Science from Online Tutorials:
- similar to the above path, you may be learning something incorrect, which can be frustrating
- there are so many examples to choose from, so which do you pick?
- sometimes people can confuse examples and Data Science topics
Overall, if you are searching for some help, whether that be learning a whole topic of Data Science from start to finish, or from a quick Google search, online tutorials can prove to be the most versatile and sometimes, the most beneficial way to learn Data Science quickly.
We have discussed quite a few different paths to become a Data Scientist. A lot of them share some of the same qualities, while they can also have some differences. So, which path or paths to becoming a Data Scientist will you choose? There are essential factors to consider when answering this question.
Common factors include the following:* age* current job * price* convenience* specialization* flexibility* reputability
You can also choose more than one path, as I am sure many already do. So, to summarize, here are all of the top or most common paths you can pursue to become a Data Scientist:
- Traditional Graduate Master’s Degree
- Certifications
- Bootcamps
- YouTube
- Online Tutorials
I hope you found my article both interesting and useful. Please feel free to comment down below if you agree or disagree with the Data Science paths that I have discussed. Which path or paths have you taken? Will you take just one, or all five, and why or why not?
Here, you can find my previous, most recent article discussing the top five Data Science qualifications [9]:
These are my opinions, and I am not affiliated with any of these companies. Thank you for reading, I really appreciate it!
Please feel free to check out my profile and other articles, as well as reach out to me on LinkedIn.
[1] Photo by Bostan Florin Catalin on Unsplash, (2020)
[2] Photo by Vasily Koloda on Unsplash, (2018)
[3 ] MastersInDataScience.org is owned and operated by 2U, Inc.
© 2U, Inc., Best 23 Schools with Data Science Master’s Programs, (2021)
[4] Photo by Lewis Keegan on Unsplash, (2020)
[5] Photo by heylagostechie on Unsplash, (2018)
[6] General Assembly, General Assembly Data Science, (2021)
[7] Photo by Christian Wiediger on Unsplash, (2018)
[8] Photo by Luke Chesser on Unsplash, (2019)
[9] M.Przybyla, The Top 5 Data Science Qualifications, (2021)