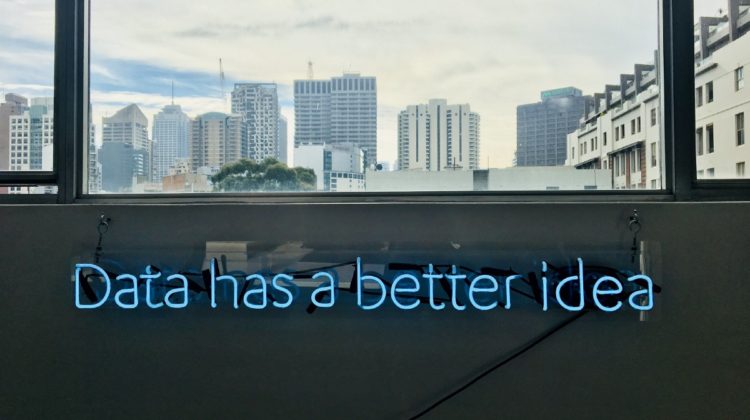
The total amount of stuff shuffled around the world is truly dizzying. And everything produced based on a wrong forecast can only become waste.
There are more than $2 trillion of inventory in the United States alone, $2.04 trillion last time I checked — $2,040 billions. And that is just the United States, a country that tracks figures closely. Keep in mind the US is a service-driven economy, and a fraction of global GDP anyways.
$1.43 of inventory for every $1 in yearly sales: which means 43% more inventory today, just right now, than all the product sold throughout this entire year.
Faced with the choice of producing more or risking to sell less, any manager would easily pick the first option. But what happens to our planet?
We waste huge resources every day
According to the United Nations, the waste created is huge. How big?
- The world produces as much as 50 million tonnes of electronic and electrical waste a year, weighing more than all of the commercial airliners ever made. Only 20% of this is formally recycled.
- The world wastes 1.3 billion tons of food each year. One-third of all food is wasted according to a different report of the United Nations
- Each European wastes 179kg of food per year according to the Swedish Institute for Food and Biotechnology.
While 45% of children under 5 years of age die because of undernutrition (source: World Health Organization), our supply chains waste one third of the food produced every single day.
What if we better matched supply and demand instead?
If somehow we could prevent the gaps opened up by forecasting errors, the benefits to the planet, the economy and ultimately everyone would be huge.
How can forecasting errors be avoided?
Solve for impact, not accuracy; let data and machines find their own answers.
Where prediction is impossible, focus on Rapid Response instead.
Remember the theory of Action/Reaction/Feedback? Years spent in school and university learning about cause-effect Action/Reaction — with maybe a footnote somewhere saying that Feedback is important. As far as I can remember, nowhere did theory help explain how to actually build systems around feedback. Since feedback can be unpredictable, classical theory tends to ignore or under-study it. Easier to make a career studying predictable cause-effect links.
What if, on the contrary, you built a Supply Chain system based on feedback instead? A prescriptive system is geared towards continuous learning & discovery: a feedback-first machine.
Now how to build a supply chain learning machine? Let us cook a succesful yummy recipe using 5 secret ingredients.
Why not target accuracy? This is a profound cultural shift for many people whose entire job description was to build accurate systems.
At least in the traditional definition of predictive systems, accuracy is measured as observed versus predicted demand, which is less relevant to prescriptive systems. A prescriptive system is literally a specific type of learning machine that efficiently targets optimal results for its objective. If you set an inaccurate objective by asking the wrong question, then you will get optimally wrong results.
Not just wrong, but very efficiently wrong, even!
Here are some examples of how traditional accuracy can be inefficient business-wise:
- Business outcomes are measured in $. Imagine to predict you will sell 10, and then sell 20 — which means you will need to replenish faster. This would be a 100% inaccurate prediction: error of 10 on a baseline of 10. But it is also a 100% business gain! Why a penalty to a 100% gain? In principle it’s understandable, but business-wise it is wrong, makes no sense really when you think about it.
- Cost of risk. Not all errors have the same cost. The previous example shows one case of understock (sell more than expected); what if instead we end up overstock? Imagine you have two products A and B, normally a fast-seller and a slow-seller, selling respectively 100 and 10 units per week. You run a promo and predict 300 sales of each, but they only sell 200 each. Both forecast errors are 50%, but the accuracy measure tells you nothing about the risk of having left 100 extra pieces of A (just 1 week of regular sales) versus B (10 weeks of sales!). Poor metric.
- Customers substitute across products. Measuring granular accuracy assumes that sales of different products are independent, which is incorrect. For example you have two products, A and B, and estimate to sell 10 of A and 10 of B. Then you have the same 50% average error (MAPE) for the scenarios where the actual is 5&15 (substitution) or 5&5 (same proportion but less overall). In the first case, the aggregate estimate of 20 was 100% right, but in the other case it’s 50% wrong. Accuracy tells you nothing valuable about substitution really!
- Probability versus reality. Expected sales are a probability distribution a priori, and there is no good way to assess the actual probability distributions from observed outcomes a posteriori, without adding noise and distortion. You start with a curve (expectation), end up with a number (sales), how to tally the two against each other?
- Demand versus sales. You can only direct observe sales, not the underlying demand. Therefore even at a conceptual level you cannot measure demand accuracy, which would be the correct metric.
All these points are examples suggesting much the same thing: accuracy is just not such a relevant business metric overall, and specifically it should never be used to build or measure a prescriptive system.
Rather than improving accuracy, change perspective entirely: overcome those structural limits by targeting profits directly.
The beauty of learning systems is that they can directly target any business outcome you want to set for them. Profit is much more relevant to any business than accuracy.
If you are like me: then at this point you should not be fully convinced yet! Where is the data? Where is the evidence?
Feeding forecasts into separate logistics systems destroys value. The magic, “secret” recipe is instead to: blend ops costs and future revenues together!
Unfortunately managers in companies today are suboptimally split into silos. One my larger clients explained just yesterday how their forecast feeds the order system that in turn feeds the shipment system. Three steps away removed from the customer and the market.
Each step breaks up the problem into manageable sub-chunks that may make sense from the management point of view, but dissipate information and therefore ultimately create waste. Destroying value from the shareholder’s point of view.
Here is why, in numbers. Using a simplified but relevant example.
Let us imagine you are the supply chain manager of a grocery retailer selling bananas. You need to decide how many bananas to ship today to your store. Similar logic would apply to any type of product or service and any time horizon, but let’s keep things simple for the argument’s sake.
Let us imagine that your true expected Demand function for bananas is a classical normal distribution, with mean/mode/average demand of 50 and standard deviation of 10. Any other probability distribution could be used, or even just implied, but let us keep things very simple for this example.
Now, if someone asked you what is the forecast demand of bananas, and assuming you want to keep you job, you would likely reply with the least wrong, or the most accurate, value of 50.
You would expect actual sales to have an error in the region of ±10 (assuming 1 standard deviation just for simplicity), which is a 20% error rate; but, hey, that is actually a pretty good estimate if you go by the opening benchmark above! So all is good. Or not?
Your error measure would compare sales ex-post with expected demand ex-ante, which is conceptually wrong as they are different. But, let us accept this fault: demand is not directly observable, only sales are, therefore no traditional way could be used to directly measure your estimate of demand. OK, so let’s move forward anyways.
In fact, coming from a corporate world based on forecasts, you are likely armed with a traditional system that thinks in terms of point estimates, so you may need to think a little bit to match your traditional logic with the probability distribution example. But it is doable.
However, there is a much bigger problem.
Forecast error is really not the problem: entrenched in the forecast/order/shipment process, a huge systematic usage error occurs every day, even with perfect forecasting.
If I were the logistics manager receiving your estimated forecast of 50 pieces sold, I would try to fulfill this at my best; due to operational constraints, I may only be able to fill 30 pieces today, but let us even assume I am so great at my job that you can get a 100% fulfillment rate from me, so I will just pass along your forecast pieces efficiently to the store. What happens then?
Every shipment effectively trades-off a guaranteed cost today for a likely profit tomorrow.
- Today, I spend money directly for guaranteed costs in inventory, logistics, finance, shrinkage (the product lost in transit) and overhead
- Tomorrow, I will generate potential profit from units sold out of those we had shipped, but also additional cost from waste and returns where product is left unsold.
Depending on the exact values of costs and profit, optimal shipment can be entirely different from forecast, even if zero-error.
For example assuming a total cost of 40 for every unit shipped, an expected gross profit of 60 (assuming list price of 100 and product cost of 40) for every unit sold, and standard deviation of 35, then the curve of expected profit based on the number of shipments N is as follows:
However, if instead assuming a total cost of 10 for every unit shipped, and the original standard deviation of 10, then the curve of expected profit based on the number of shipments N changes as follows:
NOTE: full workings in the APPENDIX at the bottom of the piece, also including the downloadable illustrative source file for detailed review of the example.
In this example, by shipping 50 units you are GUARANTEEING a systematic profit mistake, even with a correct forecast. Direct negative impact on profits. By cutting out all valuable demand information between forecasting and logistics, using a single point estimate as demand input for your separate logistics system, you will have systematically destroyed value.
The logistics system would not be able anymore to make an informed assessment of optimal shipment, let alone of more complex cross-product estimates like substitution. Just try to fill the point estimate of demand.
Traditional forecasting is not aligned with the perspective of customers, and therefore destroys business value by virtue of its own design.
Interestingly, the simplified but realistic expected profit curve above shows a strong skew. Since the expected profit is much higher on the right-hand than on the left-hand of the optimal solution, managers instinctively tend to over-ship, creating systematic waste (see the section on our planet above) rather than risking to miss out on valuable sales.
Ever heard of safety stocks systematically added on top of estimates, anyone?
You do not trust your own forecast, so you keep a margin of safety. In fact you really should not trust the process overall: even when the forecast is right, the system will still miss out on opportunity.
So you keep wasting your own stock and miss out on sales opportunity.
Depending on the standard deviation of demand i.e. how volatile and unpredictable the market is, as well as on logistic costs, the optimal shipment could take any value from 0 to 100+, even while the forecast is 50!
Let us deal specifically with risk, or to be more technical the demand variance. What happens when demand becomes more volatile and less predictable? Even with the same forecast of 50?
In this example, the max accuracy prediction is still 50, but instead of a standard deviation of 10, let us assume this is now 5x more volatile, therefore also standard deviation is now 50.
In the modern world, demand tends to become more and more unpredictable after all, making traditional forecasting even more obsolete. So this is a common scenario. But risk can also be upside opportunity!
In this case, the optimal shipment would not be 60 anymore, but 98. Broadly 2x the forecast of 50. By using traditional forecasting, you would introduce a huge systematic profit error.
And you would be left none the wiser, because you would keep shipping 50 and being close to 100% accurate by selling all 50 pieces.
On average sell 50. Which means that sometimes you would still not be out-of-stock, making the detection of missed opportunity quite tricky. And you would continue to repeat the same mistake over and over, until the demand function will change — for example because a competitor would get your missed sales of bananas and thank you for the new customers gifted to them.
Business opportunity gone forever, all while being nearly 100% accurate.
Better to be rich or right?
This simplified treatment assumes you are going to ship bananas today for delivery tomorrow to the store.
In other scenarios, frequency of shipment may be lower, lead time of shipment (the time it takes between order and receipt) may be longer. Technically, a predictive system would try to forecast sales forward, making the forecast error even larger.
A prescriptive system, on the other hand, can just take those parameters as input: the longer the lead time, and the slower the shipment frequency, the greater uncertainty in demand and the greater would be the systematic error of traditional predictive systems and processes.
What about constraints?
For example, if I only have 100 units of bananas in my warehouse and assuming I want to keep 1 to eat myself, depending on total uncertainty and cost per unit of stock, I may ship anything from 0 to 99 units. Even while ALL these scenarios have the same forecast demand of 50.
In this interesting table, showing optimal shipments anywhere from 0 to 99 even while forecast demand is always 50, the bottom left could show higher numbers than 99 had I not had the warehouse constraint (and the sneaky appetite to eat 1 banana myself!). But other than that, the whole discussion of constraints is really simple: limiting the freedom of my prescriptive system to operate.
Why, for example, imposing minimum shelf quantities? Or minimum shipment volumes, when shipment profit is already being maximized? No need, really.
Every constraint can only worsen the expected profit, but never improve it. So the fewer, the better.
More details about this part of the equation in my other Medium piece:
How to implement a rapid response system?
Prescriptive analytics are the new frontier of management effectiveness, yet they open up significant questions:
- How to determine the Demand function?
- How to make sense of system recommendations?
- How to validate results, if accuracy is not the right metric?
Every day I am more impressed as I see prescriptive systems routinely over-perform traditional predictive systems by 20–30% greater inventory efficiency: more sales with less inventory. Sometimes less can be more.
At the same time, I see the cognitive dissonance. In real quotes from managers I work with, some of whom may read themselves here:
- We have been working for years to improve accuracy by 1 or 2 points, how could a 20–30% improvement even be possible? [it’s actually very often a day 1 improvement]
- After A/B testing, how can long-term improvement be measured vis-a-vis other variable business factors? [by choosing the right target KPIs]
- Will we have the right data to estimate demand perfectly? [existing data is typically OK when enriched with external data sources; and the more the merrier, whereas perfect is not possible anyways]
Ultimately prescriptive systems can only be measured by testing them, which is a self-recursive loop: they can only work if one believes in them as the solution to otherwise unsolvable challenges.
Addressing all the complex points above extensively is beyond the purpose of this piece. So let us be inspired by analogy to other Rapid Response systems:
Analogy: Customer demand & the weather
It may be called weather forecasting, but it actually is a rapid observation — pattern recognition — update system. Since proper weather forecasting is notoriously hard, as hard as estimating customer demand really, scientists rely on advanced techniques to gather as much data as possible and adjust their estimates in real-time.
The improvement versus traditional approaches is very significant according to the NOAA: taking the pulse of the planet, processing enormous amounts of data, evolving services to the customer.
Exactly the same steps required towards a Rapid Response Supply Chain.
Analogy: Customer demand & the stock market
Unlike what some may think it to be, the stock market is not really a forecasting engine, as much as a Rapid Response system. In the long-term it tends to represent the estimated value of companies’ equity, but on a daily basis it is the most efficient engine to rapidly incorporate new information as as soon as it comes along.
Think about it: stock prices are determined in real-time by the balance of supply and demand. And this continuous discovery engine can rather efficiently allocate trillions of actual dollars every day. Or, at least, no one could come up with an even more efficient mechanism yet.
Why not adopting the same approach for the matching of actual supply and demand, for the allocation of trillions of inventory dollars in everyday supply chain decisions?
By starting with the business objective in mind, you can unlock tremendous business value every day, and in doing so, actually help our planet faster and better than many sustainability programmes ever could.
After all, Rapid Response guarantees that any error will self-correct, so what is the risk?
To build a Rapid Response system, you need two ingredients:
- Machine learning to estimate expected demand, built on as large a data set as possible
- Profit optimization to prescript decisions, using classical operations research techniques at large scale.
In a previous post, I had advocated for the salmon strategy: start from the impact you want to achieve, and working backwards then becomes relatively logical.
Like the Chinese proverb:
A Journey of a Thousand Miles Begins with a Single Step
The accuracy-driven traditional predictive process of forecast-order-shipment is broken. Embracing the Power of Prescriptive is the logical first step to finally match supply with demand in our world of ever-growing complexity.