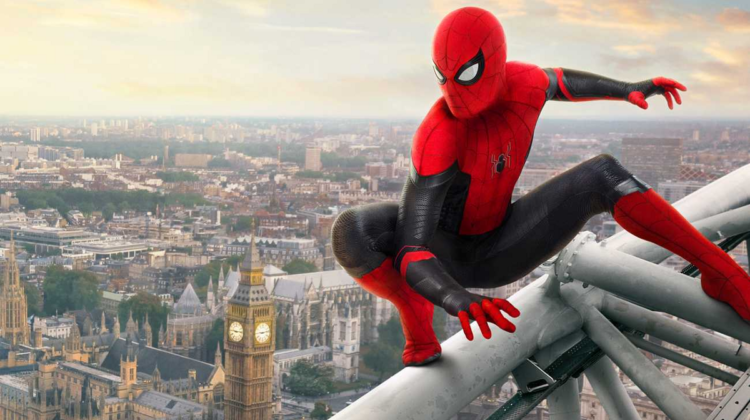

With great power comes great responsibility
Earth formed approximately 4.5 billion years ago. Life began around 4200 million years back. Primitive primates evolved around 55 million years back and humans with developed brain capability emerged around 600 thousand years ago with civilizations forming around 5000 years back. Biological evolution seems extremely slow however its still fraction of universal timelines.
First industrial and manufacturing revolution started around 320 years back developing economies and raising living standards. Then, information revolution begin with advent of microprocessors and internet within this century. Now the third revolution is brought through this decade via Artificial Intelligence (AI). AI is and will be augmenting human brain capabilities with unprecedented rates, enhancing our problem-solving capacities. Those who armed with AI, potentially can be transformed into Super Intelligent beings.
AI amplifies human intelligence and relationships between humans and AI should to be maintained synergistic and empowering rather than competitive. Yet, there are many fears around AI’s influence over human society. Unaddressed challenges in AI have to be looked at much broader sense rather than purely technological. As AI continues to undergo rapid evolution, what influences will be required so that AI enhances our life’s yet remains human?
We live in exponential progression. When progress line will became vertical, we will reach Singularity. When or if there will be singularity or humans are destined to develop non-biological evolvable Intelligence that industrialize Galaxies are still far fetched possibilities. However similar to basic literacy for every human, AI literacy can be right off everyone who would like to stay relevant.
Following list summaries current open challenges in AI:
- Ethical: Ethical behavior respects the dignity, diversity and rights of individuals and groups of people. As AI algorithm reliance increases, then honesty, fairness and equity in interpersonal, professional activities needs to be tracked and managed. Montréal Declaration for Responsible Development of Artificial Intelligence (2018) addresses the aspect of democratic control, governance and political deliberation of AI systems may need to be ehnanced to International treaty [1].
- Bias: Human biases that changes outcomes can make their way into AI systems can drive undesirable results. AI systems learn to make decisions based on training data, which can include biased human decisions or reflect historical or social inequities, even sensitive variables such as gender, race, or sexual orientation are removed. For example, facial analysis technologies had higher error rates for minorities and particularly minority women, potentially due to unrepresentative training data [2]. Measure of subjectivity and bias are yet unsolved parameters of AI.
- Inclusion: Recently AI have become increasingly common in human resources (HR) applications, such as resume parsing and employee attrition and turnover prediction. AI is used to make these tasks more efficient, it heavily relies on data created by humans. This has spurred active research on the topic of fairness in AI over inclusions of minority and women without discrimination [3].
- Security: Techniques and best practices to manage security are critically important as AI systems can be manipulated, evaded, and misled resulting in profound security implications for applications such as network monitoring tools, financial systems and autonomous vehicles. There is a pressing need for formal methods to verify AI and ML components, both independently and in concert as it relates to logical correctness, decision theory and risk analysis. New techniques are needed that specify what a system is expected to do and how it should respond to attack [4].
- Trust: To drive high-stake decisions in the contexts of legal, healthcare or military, AI must be calibrated for trustworthiness. Parameters to gauge trustworthiness can be Fairness, Explainability, Auditability and Safety (FEAS). Also, trust can be impacted throughout the life cycle of AI-based systems [5, 6] and need to be managed throughout.
- Transparency: Transparency in AI is particularly addresses issues of accountability. At the same time ‘transparency’ has multiple dimensions from system’s perspective. Rather than focusing on the individual algorithms or components, it is therefore broader term than algorithmic transparency. In order to understand transparency in AI as an applied concept, it has to be understood in context mitigated by literacies, information asymmetries, model definition as well as a set of competing interests. Transparency in AI, consequently can best be seen as a balancing of interests and a governance challenge demanding multidisciplinary development that needs to be adequately addressed [7].
- Democratization: AI democratization can ensure that the benefits of this technology are not limited to a small group of people. Democratization of AI offers three main benefits, first it reduces entry barriers, second it reduces the overall cost and thirdly it increases the speed of adoption. As current democratization efforts continue to focused towards access to data, algorithms, storage, compute, model development and marketplace, we need to move beyond just democratizing access for AI to a number of value drivers of democratization that can ensure AI is not mis-used [8].
- Generalization: Despite of existential progress in AI, broadening its applications from the lab environment to real-world applications continue to be challenging as algorithms often learn to use only a specific objective in a specific environment. AI is approaching near human Intelligence in many tasks, generalization becomes a critical three-way intersection of compute, neuroscience and psychology. We need to model how human intelligence really works by avoiding teaching machines concepts rather directly but through multiple-choice datasets [9].
- Regularization: As the word regularize implies, it make data regular or acceptable. Regularizations techniques are used to reduce error by fitting a function to the given training data set to avoid overfitting. While there is significant research in this area file systems to store AI specific data, parameters and transformations can be improved especially for those who would like to duplicate results. These file systems can make AI more practical rather than academic.
- Privacy: Many free apps from number of leading organizations sell your usage data and preferences to advertisement agencies. AI applications can go much beyond and inclusive of your thoughts and feelings, images, biological data, personal communication, location and space, association or grouping, identifiers and passwords, behavioral and actions, health records so on. Anonymize data, compliant privacy policy, right to be forgotten, legally adequate security practices needs to be considered for a comprehensive compliance program has to be essential part of AI development [10].
- Maturity: From research publications that demonstrate some advancement in AI and utilization of that advancement for common good or within some product are two completely different regimes. AI management framework with five maturity levels of the autonomous driving framework are being proposed as Level 0: characterized by an Initial Intent, Level 1: typically evolves towards an Independent Initiative, Level 2: advanced management leads to Interactive Implementation, Level 3: Interdependent Innovation, Level 4: A close combination of AI and human knowledge enables a sustainable competitive advantage with Integrated Intelligence, Level 5: draws on the intelligence-based approach to company performance, and it provides the basis for an AI maturity assessment in organizations. This framework need be extended beyond autonomous driving [11] to other AI applications.
- Virtualization: Existing virtualized cloud applications are assigned with dedicated CPUs that supports features like guest OS management. As AI as a Services (AI-aaS) on Software-Defined Infrastructure becomes ubiquitous and runs on HW accelerators such as GPUs or TPUs, applications will need to be virtualized [12].
- Culture: Problems can arise even when culture is used with good intentions. Trait list can be used track culture as difference or other important considerations. It encompasses diversity of subcultures, social groups, and individual differences. Cultural processes frequently differ even within close groups. Culture is not static or permanent and it is always changing. Some aspects change quickly for example music tastes, hairstyles, fashion trends and diet fads. Other aspects change slowly. How do AI principles translate linguistically and culturally across borders? While AI systems hold incredible promise for social goods like improved agriculture, better medicine and more accessible education, so far this promise comes with a dark side. AI systems have a pattern of entrenching and amplifying social inequality [13].
- Educational: Advantages of AI in Education can include education at any time, self paced customized or individualized education per need and interest and virtual mentors. AI can enable globalized learning platforms and students can learn from remote locations. There are a lot of AI-based solutions that improve the education field. However, this industry is quite promising due to incredible opportunities for development [14].
- Scaling: AI is supposed to mimic brain functionality. Code designed to provide functionality can be tested with predefined algorithms. Many AI algorithms are expected to get unpredicted data inputs in field. Also these algorithms can be used in wide environments like R & D, Manufacturing, Retail, Agriculture, Supply chain, Commercial so on. Deployment of these frameworks from labs to production environment is harder than conventional deployments [15].
- Platform: AI Platforms facilitates building, migrating, modeling, training, deploying, automating, forecasting along with many others aspects for managing AI applications. AI platforms are crucial for stepping into the modern era. The benefits they provide are compelling yet insufficient to resolve major open concerns. Most of existing platforms are good for point solutions and provide initial values but not capable for scaling and long term value propositions. AI platforms need strategic alignment for gathering right data, ensure data quality, protect data, shared, disaster proofing data and much more.
- Fabric: Such radical technology deployment that requires new silicon HW will also need end-to-end capabilities such as AI algorithms compilers, network protocols, file systems, drivers, schedulers to enable rapid automation. AI Fabric requirements differ compared to good old von Neumann Architecture.
- Data management: Align data strategy with business outcomes, bridge multidisciplinary teams comprising technical, business, regulatory and domain specialists are key to build a scalable data-based AI solution. Lot more needs to be done to define storage and retrieval strategy, data types, hyper parameters, optimizations, training data sets, real time inputs, logs, results tracking so on.
- Information gap: Human brain can store approximately 2.5 peta bytes of data and every person out of 7 billion global population have unique experiences, expectations, aspersions. Digital data stored in the world exceeds 125 zeta bytes and most of the data remains unusable.
AI relies on training data and larger well funded players have an incredible advantage. Often, they lead by tapping the information flowing across their platforms. Digitization is the new currency for businesses however extracting value from it does take lots of effort. Learning how to connect dots based on data from various sources, process and analyze their outputs to achieve diffusion. And when harnessed correctly, data can provide invaluable insights into a business performance and bottom line. - Traditional economics vs digital economics: The digital economy is driven by the information and communication technology revolution. Declines in the costs of computation, information storage and communication have enabled new ways of serving old needs. Social media giants can utilize the user data to manipulate advertising however what is the price tag or user behavior patterns? AI needs lots of data to learn and if this data provides value to the end user as well as service provides then we need some systems to optimize these values rather than breaking the links under privacy constraints.
- Digital channels: As AI becomes ubiquitous, machine generated data and machine to machine communication will be growing at faster pace than human generated data and communication. Power and speed constrains between machine driven system will be of much variable ranges. Principals on which next generation internet will be build also needs to account the future of AI. Defining features to the new generation digital channels need to include content-addressable storage, peer-to-peer routing, personal cloud computers, network OS and decentralized identity [16].
- Infrastructure: As AI evolves infrastructure needs for AI will evolve as well. Convergence to low power neuromorphic computing and nanophotonic communication devices will prompt new infrastructure architectures. While most of the research focused on AI algorithms, there is much bigger scope to many challenges in infrastructure space.
- Practical applications: AI have potential to impact every aspect of life and have been successfully deployed in many applications yet its usability potential is significantly higher compared to currently deployed applications. Most of the existing AI application deployments are related to image processing, yet there are many high value use cases that requires further research. Research community seems to be focused on low hanging fruits rather than fundamentally advancing AI to broaden its reach to more use cases. [17]
- AI Literacy: Defined as a set of competencies that helps to utilize, communicate and collaborate effectively with AI. AI literacy need not include understanding of algorithms but encourage learners and especially young learners to be critical consumers of AI technologies by questioning its intelligence and trustworthiness. What do AI experts think non-technical learners should know about AI? and What existing perceptions and misconceptions do non-technical learners have when interacting with AI? [18]
- Debugging: How to fix AI when it misunderstood’s the world? Inability of computational systems to understand the meaning makes them a dangerous tool for decisions that involve qualitative and sensitive judgments. For example, who should be hired for a job, get parole or admitted to the college. Importance of human-centric design and human-machine collaboration as a counterbalance to technological chauvinism is still remains essential for dealing with the edge-cases that computers are fundamentally incapable of resolving [19].
- Knowledge transfer: Humans have inherent ways to transfer relevant knowledge from previous learning experiences when encountered with new tasks. The more related a new task is to the previous experience, the more easily we can master it. In contrast, most of the machine learning algorithms address isolated tasks. Transfer learning attempts to address this by developing algorithms to leverage knowledge learned in one or more source tasks and use it to improve learning in a related target task [20].
- Hidden layers representations: Deep Neural Networks(DNN) have been evolved as a powerful function approximators to solve a wide range of problems. However, a clear understanding of their inner workings has not been satisfactorily understood yet. Factors such as the architecture, number of hidden layers and neurons, and activation function are largely determined in a guess-and-test manner that is reminiscent of alchemy more than of chemistry [21].
- Self supervised learning: As the rate of data generation is far more than current analysis capabilities, self supervised learning is a compelling possibility. With self supervised leaning, AI not only learns the decisions but also learns decision making process. Even though self-supervised learning have achieved some success recently, only few publications investigate the inner workings behind it [22]
- AI productization process: Successful engineering systems and products follow well-defined and streamlined processes. Testing standards ensure high quality and reliable results for all customer uses and corner cases. On the other hand, development and deployment of AI systems can be challenging due to unpredictable misuse and data unavailability to test all corner cases resulting expensive consequences.
- AI infuse and defuse: Can we hold a machine liable for any risky or wrong choice? With AI, the liability model shifts to producer rather than consumer. Deepfakes can have devastating consequences if we can’t develop forensic capability to defuse this technology. For example, auto-bots influencing election by spreading false and misleading information across social media networks.
- Displaced job revitalization: AI have huge potential to create value across many sectors. AI deployment can lift the global economy and increase global prosperity. AI technologies can live up to their full potential for the good of humanity. Studies find that about 30% of tasks in 60% occupations could be automated and around 15% of the global workforce or about 400 million workers could be displaced due to AI automation by 2030. We have work for everyone today and there will be work for everyone tomorrow, yet the work will be different requiring new a far greater adaptability of the workforce than we have seen [23].
- Regulation: One of the key concern with AI is, it may present new and unknown risks which current laws may not be able to cope. Hence, we may need new rules and regulations to mitigate the risks posed by AI. Regulators need to focus on things matter most rather than creating a drag for advancement of AI.
Future holds new challenges and new promises. Even though many hard problems remains to be solved and will require major scientific breakthroughs, AI’s impact on humanity will likely to be profound given productivity gains drives economic growth and AI augmenting human capabilities, freeing up resources to engage in higher value tasks assuming businesses and governments can proactively manage this transition.
[1] The Ethics of AI Ethics: An Evaluation of Guidelines
[2] Gender Shades: Intersectional Accuracy Disparities in Commercial Gender Classification
[3] Ethical Considerations in AI-Based Recruitment
[4] Artificial intelligence and cybersecurity: opportunities and challenges
technical workshop summary report
[5] Rapid Trust Calibration through Interpretable and Uncertainty-Aware AI
[6] The relationship between trust in AI and trustworthy machine learning technologies
[7] Transparency in artificial intelligence
[8] Democratization of AI: A double-edged sword
[9] What Can AI Learn from Human Intelligence?
[10] Top Five Data Privacy Issues that Artificial Intelligence and Machine Learning Startups Need to Know
[11] Five Maturity Levels of Managing AI: From Isolated Ignorance to Integrated Intelligence
[12] Artificial Intelligence as a Services (AI-aaS) on Software-Defined Infrastructure
[13] Global AI Ethics: A Review of the Social Impacts and Ethical Implications of Artificial Intelligence
[14] Artificial Intelligence in Education: Benefits, Challenges, and Use Cases
[15] Scaling AI: 5 Reasons Why It’s Difficult
[16] Five Defining Features to Build the New Generation Internet
[17] 100+ AI Use Cases & Applications in 2021: In-Depth Guide
[18] What is AI Literacy? Competencies and Design Considerations
[19] Artificial Unintelligence: How Computers Misunderstand the World
[20] Transfer Learning
[21] Hidden representations in deep neural networks
[22] Self-supervised Learning: Generative or Contrastive
[23] AI, automation, and the future of work: Ten things to solve for