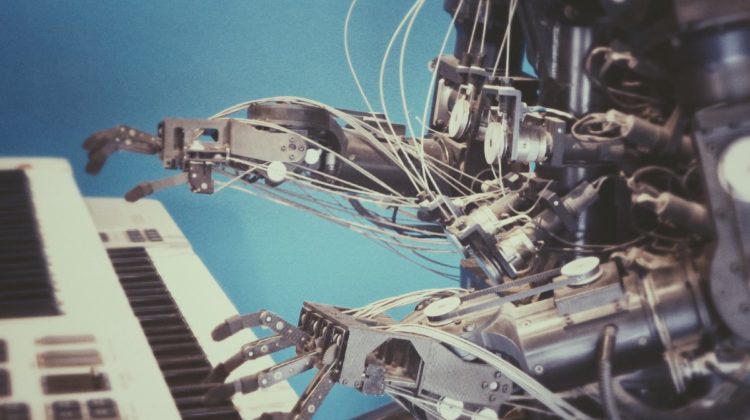
One of the other great successes of AI is speech recognition algorithms. With various deep learning values (phonetic analysis, word combination, and sentence structure) these have achieved up to 90% recognition accuracy. Yet, these technologies seem to increasingly to meet a dead end related to ambient noise’s sound disruption and word subtle similarities.
To overcome it, researchers have tackled other areas, such as translation or sentiment analysis. For translations, scientists started by identifying the words’ meaning by assigning them a number, but quickly realized the limitations of this technique. A number cannot account for the numerous connections between words from very different fields (for example the word “charm” is linked to “seduction”, but also to “humor” and “intelligence”).
To embody these semantic similarities, they conceived a multi-dimensional semantic space, where words can connect simultaneously to different associations. In other words, researched began to define words as vectors. Google Word2vec’s job was precisely to make an algorithm learn millions word vectors.
Armed with this semantic analysis, the researchers tackled then deep-learning-based translation. They designed a “recurrent neural network” recurrent that understands words’ meaning as the sentence progresses. Indeed, each previous word read by the algorithm becomes an output in the form of a vector, which will help in understanding the next word. However, it was easy for the program to forget information from the beginning of the string in their translation. Thus, researchers have invented “long-short-term memories” that select the inputs to be kept in memory.
With these recurrent neural network, machine translation has made remarkable progress, as you may have noticed with the new-found accuracy of Google Translation since 2016. And Google and other tech companies have quickly expressed their enthusiasm for a general intelligent machine in the translation field.
However, these algorithms still show some major flaws. They have a hard time translating ambient word matches, which depend on the context and the meaning of the sentence (such as a phrase like “take if with a grain salt”).
Also, they don’t have a basic elementary and visual knowledge of things (“a glass is filled with water, drunk by a human, held by hand”). Machines can easily confuse the role of the glass with that of the water or the hand, and thus translate the sentence in the wrong way (“it holds the water” being “the hand holds the water”) for example. Consequently, without the help of a human to adjust, they lack a fundamental understanding of words, which is related to human common sens.