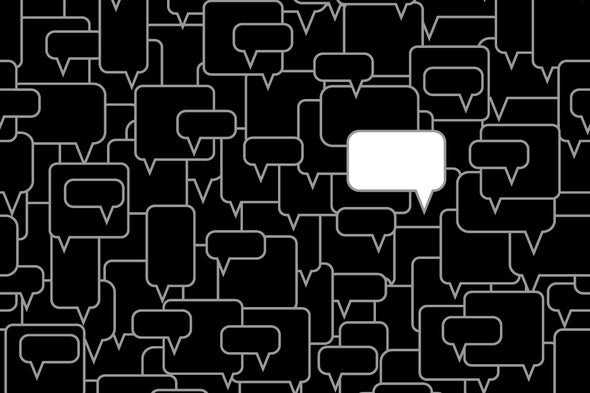

The benefits of mobile technology are not accessible to most of the world’s 700 million illiterate people
By Moussa Doumbouya, Lisa Einstein, and Chris Piech
Note: This article originally appeared in Scientific American
When we asked Aissatou, our new friend from a rural village in Guinea, West Africa, to add our phone numbers to her phone so we could stay in touch, she replied in Susu, “M’mou noma. M’mou kharankhi.” “I can’t, because I did not go to school.” Lacking a formal education, Aissatou does not read or write in French. But we believe Aissatou’s lack of schooling should not keep her from accessing basic services on her phone. The problem, as we see it, is that Aissatou’s phone does not understand her local language.
Computer systems should adapt to the ways people — all people — use language. West Africans have spoken their languages for thousands of years, creating rich oral history traditions that have served communities by bringing alive ancestral stories and historical perspectives and passing down knowledge and morals. Computers could easily support this oral tradition. While computers are typically designed for use with written languages, speech-based technology does exist. Speech technology, however, does not “speak” any of the 2,000 languages and dialects spoken by Africans. Apple’s Siri, Google Assistant, and Amazon’s Alexa collectively service zero African languages.
In fact, the benefits of mobile technology are not accessible to most of the 700 million illiterate people around the world who, beyond simple use cases such as answering a phone call, cannot access functionalities as simple as contact management or text messaging. Because illiteracy tends to correlate with lack of schooling and thus the inability to speak a common world language, speech technology is not available to those who need it the most. For them, speech recognition technology could help bridge the gap between illiteracy and access to valuable information and services from agricultural information to medical care.
Why aren’t speech technology products available in African and other local languages? Languages spoken by smaller populations are often casualties of commercial prioritization. Furthermore, groups with power over technological goods and services tend to speak the same few languages, making it easy to insufficiently consider those with different backgrounds. Speakers of languages such as those widely spoken in West Africa are grossly underrepresented in the research labs, companies and universities that have historically developed speech-recognition technologies. It is well known that digital technologies can have different consequences for people of different races. Technological systems can fail to provide the same quality of services for diverse users, treating some groups as if they do not exist.
Commercial prioritization, power and underrepresentation all exacerbate another critical challenge: lack of data. The development of speech recognition technology requires large annotated data sets. Languages spoken by illiterate people who would most benefit from voice recognition technology tend to fall in the “low-resource” category, which, in contrast to “high-resource” languages, have few available data sets. The current state-of-the-art method for addressing the lack of data is “transfer learning,” which transfers knowledge learned from high-resource languages to machine-learning tasks on low-resource languages. However, what is actually transferred is poorly understood, and there is a need for a more rigorous investigation of the trade-offs among the relevance, size and quality of data sets used for transfer learning. As technology stands today, hundreds of millions of users coming online in the next decade will not speak the languages serviced by their devices.
If those users manage to access online services, they will lack the benefits of automated content moderation and other safeguards enjoyed by the speakers of common world languages. Even in the United States, where users experience attention and contextualization, it is hard to keep people safe online. In Myanmar and beyond, we have seen how the rapid spread of unmoderated content can exacerbate social division and amplify extreme voices that stoke violence. Online abuse manifests differently in the Global South; and majority WEIRD (Western, educated, industrialized, rich and democratic) designers who do not understand local languages and cultures are ill-equipped to predict or prevent violence and discrimination outside of their own cultural contexts.
We are working to tackle this problem. We developed the first speech recognition models for Maninka, Pular and Susu, languages spoken by a combined 10 million people in seven countries with up to 68 percent illiteracy. Instead of exploiting data sets from unrelated, high-resource languages, we leveraged speech data that are abundantly available, even in low-resource languages: radio broadcasting archives. We collected two data sets for the research community. The first, West African Radio Corpus, contains 142 hours of audio in more than 10 languages with a labeled validation subset.
The second, West African Virtual Assistant Speech Recognition Corpus, consists of 10,000 labeled audio clips in four languages. We created West African wav2vec, a speech encoder trained on the noisy radio corpus, and compared it with the baseline Facebook speech encoder trained on six times more data of higher quality. We showed that, despite the small size and noisiness of the West African radio corpus, our speech encoder performs similarly to the baseline on a multilingual speech recognition task, and significantly outperforms the baseline on a West African language identification task. Finally, we prototyped a multilingual intelligent virtual assistant for illiterate speakers of Maninka, Pular and Susu (see video below). We are releasing all of our data sets, code and trained models to the research community in hopes it will catalyze further efforts in these areas.
Early computing luminaries knew that in order to make programming accessible to the masses, they would need to create programming languages that were easy for humans to learn. Even then, the first high-level programming languages were highly technical. Users today benefit from multiple levels of abstraction: you don’t need to understand JavaScript to read this article on your computer, and AI researchers do not need to interact with assembly code to advance the field of computer science.
Still, computers are not yet sufficiently evolved to be useful in some societies. Aissatou should not have to read and write a common language to contribute to scientific research, much less to merely interact with her smartphone.
Yes, it is challenging to create computers that understand the subtleties of oral communication in thousands of languages rich in oral features such as tone and other high-level semantics. But where researchers turn their attention, progress can be made. Innovation, access and safety demand that technology speak all of the world’s languages.
About the authors:
Lisa Einstein is a master’s candidate in computer science and international cyber policy at Stanford University, where she works at the Stanford Internet Observatory. Previously, she taught physics as a Peace Corps Volunteer in Guinea, West Africa. With Moussa Doumbouya, she co-founded GNCode.
Moussa Doumbouya is a Guinean-American artificial intelligence (AI) researcher and computer science educator who investigates how AI could best serve developing countries. With Lisa Einstein, he co-founded GNCode.
Chris Piech is an assistant professor of computer science at Stanford University. He was raised in Kenya and Malaysia. His research uses machine learning to understand human learning.