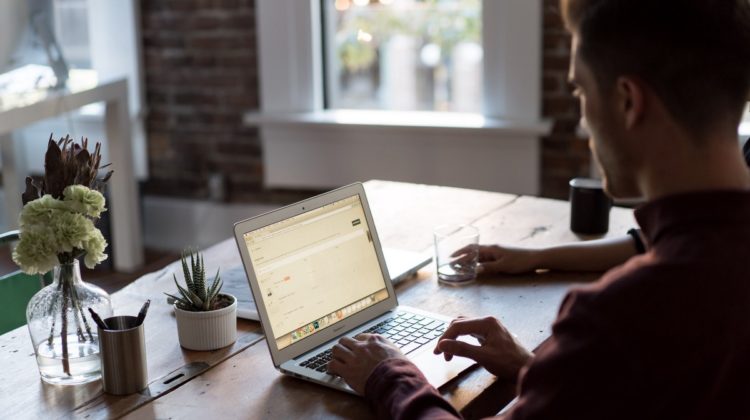
Opinion
- Introduction
- Object-Oriented Programming (OOP)
- Pandas
- scikit-learn
- Cross-Functional Collaboration
- Summary
- References
Data Science education, theory, and practice can oftentimes focus on statistics and Machine Learning algorithms. While this focus is of course necessary as these are the foundations and main facets of Data Science, there is another skill that is neglected, especially in school. This skill I am referring to is knowing how to code in Python. Of course as a Data Scientist now, you most likely already know how to program in Python, but when you are first starting out you may just be focusing on the latter instead. It is important to master Python first before learning Data Science because you will struggle to implement popular libraries and work with code that is scalable that other engineers can also work on as well. That being said, I am going to highlight a few reasons why you should learn Python first before learning Data Science.
Object-oriented programming is crucial when you are any type of engineer in the tech industry, or at least that is what my experience has been, as well as others who have worked in the industry. Sometimes when you learn Data Science, you can jump straight into the concepts and theory of Machine Learning algorithms, while of course useful, you will need to know how to apply those concepts and theories in practice — which is usually by means of a programming language. Some Data Scientists use R and some use Python, so you could apply some of these reasons to not only Python, but R as well.
Object-oriented programming consists of properties like classes, objects, inheritance, functions, methods, and instances. You can still perform Data Science processes without object-oriented programming, and most do in their research, but once you want to scale up and it is seen by more people; the codebase ultimately benefits from being more efficient.
Benefits of object-oriented programming practice through learning Python:
- modularized
- clean code structure
- scalability
- reuse of code
- security
- troubleshooting
Programming with OOP in mind leads to several benefits like the ones I included above, as well as many more. These benefits can also be shared amongst other coworkers in which I will discuss below for my last point. Now that we have discussed object-oriented programming, we can delve into some of the ways that we can incorporate OOP with a popular Python library.
Learning Python means you can learn Pandas. What is Pandas you say? Pandas [4] is a tool that you can use for data analysis that is fast and easy to use. While Pandas is often associated only with Data Science (for the most part), it is still something that you can learn beforehand for data analysis, and other calculations in different roles as well. There are countless benefits to learning Pandas, and it can be especially useful for the beginning and end parts of the Data Science process. A lot of the preprocessing steps that occur in Data Science can be performed by Pandas techniques, like exploratory data analysis at the beginning, and the interpretation of the results from your final model can be analyzed using Pandas as well.
Here are of the benefits of learning Pandas in Python:
- reshaping data
- subsetting observations
- subsetting variables
- summarizing data
- handling missing data
- making new columns
- combining datasets
- grouping data
- windows
- plotting
If you follow the above link, there is a cheat sheet that summarizes all of these further. A Pandas dataframe is a powerful part of Pandas that allows you to perform all of those data manipulations from above, and is one of the easiest ways to translate your data into a format that a Data Science model or Machine Learning algorithm can read. Similar to Pandas, there is another popular library that is easy to use and powerful that I will discuss below.
Another popular library or tool that Data Scientists often use is scikit-learn, meaning if you focus on learning this first with Python before jumping into the specifics of algorithms, you will have a better foundation of the algorithms in general. Since you would be learning this library before jumping into Machine Learning theory, you would just want to know about the possible algorithms and the different types at a high-level so that once you do start studying Data Science more specifically, you will have an idea of the range of algorithms that there are, for what I assume, with one of the most popular Python libraries (for Data Scientists). This technique might be a little unorthodox, but I believe it can be beneficial to have a simple overview of what you will eventually dive into — like learning about the main types of algorithms first and how to program with them in Python, and then getting into the specifics after so that Python is not the limiting factor moving forward.
Scikit learn [6] is a tool that allows predictive analysis, which is built on NumPy, SciPy, and matplotlib. Many Data Scientists use this library for working with a variety of algorithms.
Some of the popular ways you can use scikit-learn is by performing the following (a few examples, not limited to):
- classification — SVM, Random Forest
- regression — nearest neighbors
- clustering — k-Means
- model selection — grid search, cross-validation
- preprocessing — transformations
When you use these common libraries in Python you will want to be able to use them and discuss how they should work with other engineers as well, which leads me to my next point.
Being a Data Scientist means you will have to work with several different types of engineers, like Software Engineers and Machine Learning Engineers. Being able to communicate with them is incredibly important. One way to do that is through Python. Oftentimes, a Data Scientist can focus more on algorithms and some code; however, when they present the code for collaboration to the other engineers, it can be more messy or unclear — more research-focused and one-off oriented. Being able to write your Python code in a way that is scalable and easy-to-read will make you much better at implementing your code in a bigger repository of code.
Here are some of the benefits of Python that lead to increased cross-functional collaboration:
- being able to translate Data Science methodologies via Python
- collaborating on Python code together
- GitHub/Git pull requests
- code repositories
Being able to collaborate with others is of course a great skill to have, and it is even more important when you can apply that same collaboration to not only ideas and concepts but also to the code you are using to build your model.
As you can see, mastering Python is a crucial step in also learning Data Science. To become a great Data Scientist, there are several key concepts and skills you should acquire beforehand, like statistics and data analytics as well. For this article, I have discussed some of the key reasons why you would want to master Python before learning Data Science.
Here are those reasons summarized:
Object-Oriented Programming (OOP)Pandasscikit-learnCross-Functional Collaboration
I hope you found my article both interesting and useful. Please feel free to comment down below if you have learned Python first in some way before becoming a Data Scientist. Has it helped you in your Data Science career now? Do you agree or disagree, and why?
Please feel free to check out my profile and other articles, as well as reach out to me on LinkedIn.
Here is a similar article I wrote on Data Analytics as a prerequestie for Data Science [8]:
[1] Photo by Bench Accounting on Unsplash, (2015)
[2] Photo by Markus Spiske on Unsplash, (2017)
[3] Photo by Pascal Müller on Unsplash, (2018)
[4] pandas — NumFOCUS, Pandas Homepage, (2021)
[5] Photo by Tran Mau Tri Tam on Unsplash, (2021)
[6] scikit-learn, sci-kit learn Homepage, (2021)
[7] Photo by Marvin Meyer on Unsplash, (2018)
[8] M.Przybyla, You Should Master Data Analytics First Before Becoming a Data Scientist, (2021)