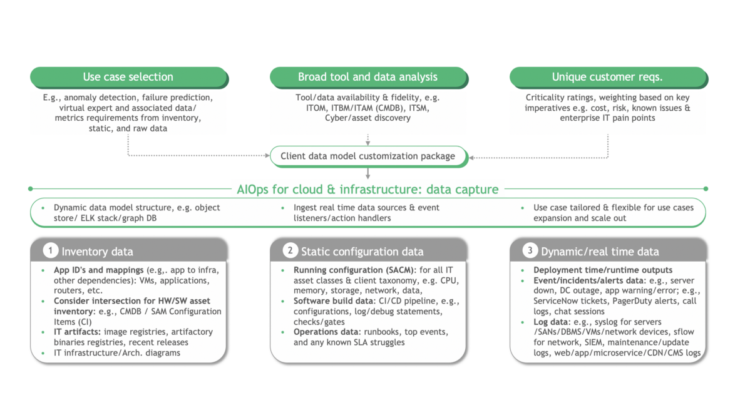

AIOps for Cloud and IT Infrastructure can enable intelligent operations for enterprise & mission critical functions
In the broader cloud market, hyperscale data centers, cloud service providers (CSPs), and overall workload adoption on public cloud in the commercial and public sectors continue to grow. Cloud adoption has steadily increased over the past several years, and it is estimated that while 75% of workloads remain on-premises in classic IT and private cloud environments, 25% of all enterprise workloads now reside in public cloud between legacy modernization & migration as well as cloud-native, digital product development and engineering (1). The growth in public cloud adoption continues, but we still have significant runway ahead of us for continued adoption, development and evolution of cloud operations.
However, with legacy application modernization and data migration, legacy ways of working and operational constructs can and have already replicated themselves in the cloud as well. Many legacy IT operations process, governance constructs & financial management approaches have been replicated from the data center into the private and public cloud. As heterogenous IT environments grow in complexity among both classic IT infrastructure remains and cloud-native, the burden on IT operations is also growing. The flood of enterprise, operational and mission-centric data that IT must manage, the requirement of CIO’s to constantly do more with less, and the shift from legacy to next-generation security models all contribute to these operational inefficiencies (2).
This is severely limiting the potential of your cloud & IT infrastructure environment. A foundation of true cloud-native product development and operations can and should be enhanced with intelligent operations, also known as AIOps. Incorporating AIOps at every layer of the technology stack, and every phase of an IT operational lifecycle, can enhance your cloud and IT infrastructure environment to be significantly more predictive and intelligent nature, even to the point by which aspects of your environment can be “zero touch” (e.g. automated remediation and self-healing).
A fast-growing set of tools and approaches to combat these problems lie within AIOps (also referred to as MLOps depending on vendor and use case), which is a method of utilizing big data, advanced analytics and machine learning to enhance and automate IT operations & monitoring, enabling intelligent operations of your IT environment. While it is early days, IT leaders are starting to employ machine learning (ML) technologies within their own functions to effect operational efficiencies and cost reduction for enterprise technology. In the past few years, a number of vendors have begun designing and developing powerful analytics tools to address the particular challenges that IT personnel face in managing, updating, and running IT hardware and software across the enterprise (3).
Core AIOps generally covers Artificial Intelligence and Machine Learning (AI/ML) use cases in key areas of the Software Development Lifecycle (SDLC), including: Application Performance Monitoring, IT infrastructure monitoring, IT Event Correlation & Analysis, and Network Performance Monitoring and Diagnostics.
There is a mix of both tool and vendor driven enablers in this space, coupled with highly custom methods for aggregating operational data and driving differentiated insights for each AIOps use case. Some highlights include:
Cloud Service Providers (CSPs).
More generally, AWS, GCP, and Azure are highly supportive of and integrate well with the key ISVs that bring to bear AIOps capabilities into the public cloud ecosystem.
While much native tooling is generally integrated and not native, native configuration of public cloud services (e.g. CI/CD pipelines, data services), can get you quite far in this domain. Check out GCP MLOps, Azure MLOps, and AWS MLOps (as well as AWS Sagemaker), to see what is possible with native CSP services as well as their embedded Machine Learning tech before introducing integrated tooling and services.
ISVs and Commercial Off The Shelf (COTS) vendors.
Splunk, the “Data-to-Everything Platform”, and which is well known for log management, has expanded its platform as it is a logical event and data source for AIOps use cases. The Splunk Machine Learning Toolkit and Artificial Intelligence for IT Operations each provide a launch kit and tooling to operate natively in Splunk.
Moogsoft is more of a native platform engineered specifically for AIOps enablement, integrates with a number of well-known tools and data sources, and is supportive of a number of the use cases detailed below. Moogsoft delivers an enterprise-class, cloud-native platform that empowers customers to drive adoption at their own pace at a much lower cost according to their website.
Open standards & Open Source heritage.
Elastic, the creators of the ELK Stack, are one of many examples of “Open Source heritage” solutions that have expanded into AIOps functionality. Elastic supports a number of machine learning use cases to include anomaly detection from event sourced data.
IT Service Management (ITSM) platforms.
ITSM platforms and Service Operations in general are absolutely central to the process and data that unlock AIOps use cases and capabilities. The two key leading ITSM vendors are offering out of the box capabilities in ServiceNow AIOps and BMC AIOps.
Get the operational foundation right.
IT Service Management (ITSM) and operations. Perhaps a dedicated article for another day, but modern ITSM practices and operations are critical and foundational to AIOps, and the lack of integrated, automated ITIL functions for end-to-end IT processes are often a root cause for bolting on and integrating AIOps into your ecosystem for improvement of the environment. AIOps should enable continuous service improvement and a constant feedback loop for increasing the operational efficiency of your ITSM platforms and ITIL processes.
Increase your environment visibility and transparency.
Determine your initial AIOps strategy. First, determine whether you are targeting discrete use cases and opportunity areas (e.g. anomaly detection, noise reduction, incident prediction, automated issue remediation), or if this will be a broader, experimentation and discovery to identify areas of opportunity for more focused deep dive and use case development. If the former, this effort can get very targeted very quickly on the nature of data you need to source and what you are trying to solve for. If the latter, the initiative should be stood up more in a pilot fashion to experiment and identify where the value proofs are in your environment by casting a much wider net.
Conduct a targeted initial data capture. Now that you have a sense for what you are solving for (or want to identify what it might be), you can key in on the tools, data, and layers of the infrastructure stack that you need to target for data capture. The figure below provides a good framework for your unique inputs and how they will affect the inventory, static configuration, and dynamic data elements ideal for capture in any given use case:
Iteratively build and train the data model. This data capture establishes a starting point for identifying value proofs. You have created the “haystack”…. now you have to find the “needle”!
Feature engineering is now enabled by taking these ingested, raw data assets and transforming them into input variables for applied machine learning techniques, including error and incident counts, thresholds and trends in IT operations data, etc.
These input variables will present opportunities for certain use cases. For instance, normalized error and incident data may suggest a value proof around an Anomaly Detection use case for detecting early signs of deviation from normal operational infrastructure and application behavior. You may then choose certain classification and machine learning methods (e.g. Isolation Forest, clustering approaches) to further refine the data, generate an anomaly score or other measurable unit for insights, and generate a deliberate feedback loop to further refine and train the model to identify true failure events & reduce both false positives & actions. Multiple iterative, agile improvement cycles will likely be required to sharpen the data, model and insights into something truly informative to drive decision making and eventually automated action handling of identified anomalies in your cloud and IT infrastructure environment.
Here’s a much more detailed view and step by step mapping of what a use case, fully enabled in your environment, could look like for you:
Reduce the noise.
Convert visibility into findings that create a deep understanding of the issue. This iterative process to sharpening the data and findings will have a “noise reduction” effect in identifying what actually matters by removing false positives and / or negatives and exposing what is truly actionable, or informs some action, in your environment. These insights generated span a wide range of core use cases, including:
· Noise reduction: the act of noise reduction itself is foundational to analyze data and patterns of alerts and subsequently filtering out false positives in order to enable more progressive use cases below
· Triaging and alert correlation: clustering and correlating alters from segments of your cloud & IT infrastructure environment into a single, discrete incident in order to accelerate and provide transparency into the triage process
· Service impact analysis: drawing dependency mappings across clusters of identified events to analyze downstream or cascading effects, both technical and business side, of IT failures, outages, and other disruption events
· Root cause analysis: identifying and inferring the root causes of realized disruption events in the application and cloud / IT infrastructure tiers
· Forecasting: predicting future application requirements for infrastructure capacity & demand allocation
· Incident prediction: translate detected anomalies across the IT environment to predict and prevent future failures
… “extended” use cases can involve integration of these core examples out to DevOps / DevSecOps pipelines, business outcomes, IT Service Management (ITIL modules and processes), and core Network and Security Operations (NOC/SOC).
Generate informed decision making and automated actions.
Leverage deep understanding of the issue into recommendations to inform decisions or even take immediate, automated remediation actions. Okay, I have significantly more visibility into my enterprise environment all of these great insights… what can I actually do with it all to translate the data into something executable to be carried out. Can I take it a step further and decompose the data into tasks that are automated for a hands off, autonomous environment. The potential is definitely here! Some key actioning use cases include:
· Remediation recommendation (manual acceleration): using machine learning techniques to develop an “expert” recommendation system to enable IT ops to more easily troubleshoot incident response
· Automated issue remediation (automation & orchestration): automatically trigger the resolution process in case of an incident, also known as “self-healing”
Below is an indicative reference architecture for what your environment could look like to include event and data sources, a custom aggregator and data lake for your AIOps use case identification and refinement, downstream visibility and action handling methods, and a feedback loop to constantly improve your cloud and IT infrastructure environment and service operations functions:
… this can be built in any on-premise environment, public CSP environment, or even distributed across a hybrid and multi-cloud ecosystem depending on your environment posture and targeted use cases.
This should provide a high-level overview for what the art of the possible holds with AIOps and how you can take a heterogeneous, complicated environment and decompose the signals from your environment sensors into a real, autonomous, continuous feedback loop of improvement for enterprise cloud and IT infrastructure operations. While nascent, the tools, capabilities, data and methods are all present for a package that will bring your cloud from initial operating capability to the “Smart Cloud”.
If you find this article informative, please also check out the broader BCG perspective on the AIOps market and uses cases, as well as my perspective on AIOps opportunities within the US Federal Government for an industry specific lens to enabling AIOps.
1. Gartner Predicts 2019. https://www.gartner.com/en/documents/3895580/predicts-2019-increasing-reliance-on-cloud-computing-tra
2. Proving efficiencies from AIOps in federal government. GCN. https://gcn.com/articles/2020/10/14/aiops-efficiencies.aspx
3. Ready or Not, AI Is Coming to IT Operations. BCG. https://www.bcg.com/en-us/publications/2019/artificial-intelligence-coming-information-technology-operations