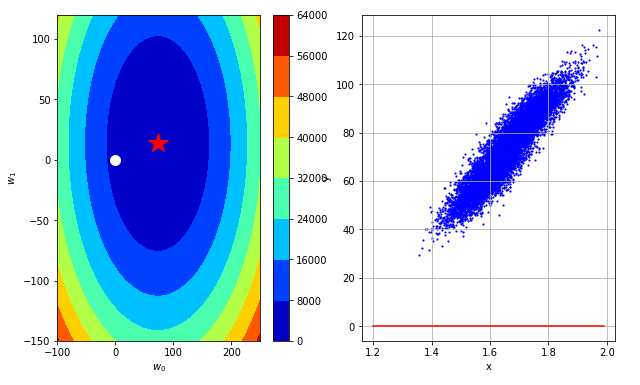

梯度下降被廣泛運用在深度學習,用於尋找目標函數的局部最小值,詳細的過程可參考這篇,寫的很詳細又易懂!這裡就把重心放在應用梯度下降於簡單的資料。
首先介紹資料,共10000筆,變數為身高(m.)體重(kg.)及性別,並用身高去預測體重:
要執行梯度下降就得先算梯度,這裡的預測是用簡單線性方程式:
矩陣裡面的1欄位是為了線性方程式中的常數項(constant term)而加的。有了預測的方程式下一步就可以計算誤差,使用MSE(Mean Square Error)當做損失函數(loss/cost function),並將其對參數微分得到梯度(gradient):
有了梯度就能結合梯度及學習率(learning rate)更新參數以尋求最佳解:
更新公式中學習率(tau)會影響參數更新的速度,太小的話更新效率容易過慢不易收斂,太大容易越過最小值但相對跌代次數少。梯度下降的過程中需先設定參數w的起始值以及跌代(Iteration)次數,參數通常尤0開始。