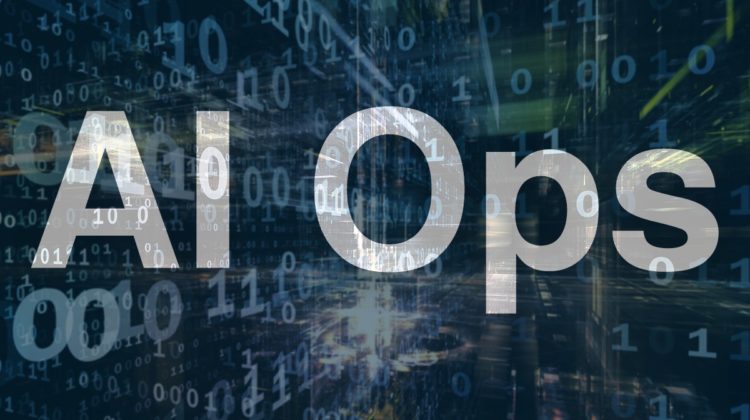

Digital Transformation Delivers Change at Scale
Just about every enterprise right now is going through some sort of digital transformation. For most, it’s about surviving, for many, it’s about disrupting and leading. Software and user experience (UX) are the new competitive edge. Managing availability and performance is now a matter of life or death for IT Operations/Devops. Digital transformation guarantees two things for IT Operations: more change and scale. If enterprises want to move faster they have to break things up into smaller pieces, and let teams work independently in an autonomous way. Agile, DevOps, Cloud and Microservices are real-life examples of this shift happening. Agile development means applications now change 10- 50X more frequently per year, and the adoption of AWS/Azure/Docker/Meso technologies now mean environments are 10–50X larger. To ensure availability and performance, enterprises today typically own 10–25 different tools, like Splunk, AppDynamics, Dynatrace, Nagios and Solarwinds to monitor their production stack of apps, network and infrastructure. It’s therefore common for these tools to generate millions of events and alerts everyday for IT Operations to analyze, correlate, prioritize and action. If it’s millions of events today, it’s billions tomorrow. Are you ready?
The Human Brain Has Limits
Research suggests that the human brain has a short-term memory capacity of between 7 and 9 items. Humans are really good at deriving meaning from a handful of data points. This cognitive limitation has survived the past decade in IT Operations where humans could deal with hundreds/thousands of events. We’ve now reached a point in time where even the smartest humans can no longer cope with the volume of events in their environments.
Introducing Algorithmic IT Operations (AIOps)
Compute power today is fast, available and cheap. Software algorithms are capable of processing millions of events in just a few milliseconds. Better still, algorithms today are actually capable of deriving meaning from large data sets on their own with/without human input. This is called supervised and unsupervised machine-learning. AIOps is about algorithms augmenting and assisting humans within IT Operations, it’s not about replacing humans.
AIOps can be applied to automate many use cases within IT Operations. A good example is incident management where AIOps can deliver massive benefits on top of your existing monitoring and service desk tools.
The Human Way
Most enterprises today have teams of NOC, helpdesk or level 1 operators who manually analyze, detect, correlate, prioritize and ticket event/alert telemetry from their ecosystem of monitoring tools. In many cases, email or a legacy manager of manger (MOM) like IBM Netcool, Microsoft SCOM or CA Spectrum is used to aggregate alerts into a central console. The result? Alert fatigue and operational noise. This is why most IT Operations teams still struggle to detect incidents and business impact before customers call the helpdesk. There is simply not enough time in the day for teams of operators to proactively analyze all the events in a manual fashion. Some enterprises actually disable monitoring alerts altogether just to reduce the operational noise. It’s therefore no surprise that nearly two thirds of incidents are still reported by customers. Missing incidents is just the tip of the iceberg. Lack of event/alert correlation means that operators will typically analyze events/alerts independently of other operators resulting in duplicate tickets, escalations and productivity burn.
The AIOps Way
Algorithms today can automate the process of analyzing and correlating event data. In fact, what takes humans hours to achieve can be done in milliseconds as alerts unfold in your environment. Millions of events can be reduced down to tens of incidents automatically, using software algorithms that can de-duplicate, blacklist and correlate event feeds in real-time. This real-time insight now allows IT Operations to be proactive 24/7. Algorithms enable humans to focus on the tens of incidents vs. millions of events/alerts that overload them every day. This level of automation means incidents can be detected instantly without requiring humans to manually connect the dots across various tools and silos. AIOps can also automate incident ticketing, notifications, knowledge re-use and decision support. For example, algorithms can blueprint every incident observed and capture all the tribal knowledge which was used to resolve that incident. Should a similar incident be observed in the future, those same algorithms can be used to automate knowledge re-use and decision support. Humans are still central to incident management, AIOps is merely increasing their productivity, responsiveness and value by automating the manual tedious tasks which they perform everyday. Algorithms on their own cannot resolve incidents or business impact.
Algorithmic IT operations platforms enable I&O leaders to meet the proactive, personal and dynamic demands of digital business by transforming the very nature of IT operations work via unprecedented, automated insight.
Key Findings
- Human capabilities, deductive reasoning and limited data analysis capacity are constraining IT operations from gaining the level of agility and insight required to support digital business initiatives.
- Current and future demands of infrastructure and operations (I&O) require a specific, strategic investment in a platform that is designed to collect and analyze data from any source with the assistance of increasingly intelligent machines.
- To date, the majority of I&O’s investments in algorithmic IT operations (AIOps) platform technologies (IT operations analytics, big data, machine learning, etc.) have been tactical and/ or isolated in nature, limiting their potential.
- Most I&O teams do not yet have the skills or experience needed to work effectively with AIOps platforms.
Recommendations
- Make a strategic investment in an AIOps platform that will support major IT operations functions (monitoring, automation, service desk and others).
- Balance ease of use with interchangeability of platform capabilities (data collection, storage, analytical engines, presentation, etc.) to avoid lock-in.
- Invest in building the skills and making the organizational changes needed to get value from an AIOps platform.
Strategic Planning Assumption
By 2019, 25% of global enterprises will have strategically implemented an AIOps platform that supports two or more major IT operations functions, up from fewer than 5% today.
Analysis
For far too long, IT operations management (ITOM) has been a series of “big data” challenges in terms of scale and complexity being managed with multiple, often isolated, and largely manual, “small data” tactics and tools. Current and future demands of ITOM cannot be met without taking full advantage of the same advanced analytical technologies used to support the most demanding of business applications (fraud detection) and deliver differentiating digital experiences to consumers (content delivery, social media). However, doing so requires discarding technological, behavioral and procedural constraints that have accumulated over decades, in favor of a data-driven, algorithmic, collaborative, even experimental approach to ITOM. This rethinking of ITOM functions based on a platform that enables the real-time and historical analysis of data from any source, assisted by machines, represents both radical change in approach and opportunity.
Definition
AIOps platforms utilize big data, modern machine learning and other advanced analytics technologies to directly and indirectly enhance IT operations (monitoring, automation and service desk) functions with proactive, personal and dynamic insight. AIOps platforms enable the concurrent use of multiple data sources, data collection methods, analytical (real-time and deep) technologies, and presentation technologies (see Figure 1).
Description
AIOps platforms are composed of multiple, loosely coupled layers that address data collection and storage, analytical engines (real time and deep), visualization/UI, and integration with other applications via APIs, as depicted in Figure 2.
The presentation layer of the AIOps platform supports multiple presentation and interaction methods inclusive of, but not limited to, both visualization and natural-language processing (NLP) as useful interfaces. The analytical learning layer of the AIOps platform supports both deep analytical capabilities (deep neural networks, deep Q-networks, deep coding, etc.), which analyze large datasets in search of probable answers to incredibly complex problems (e.g., image recognition and description), and real-time analytical capabilities, which can process high volumes of streaming data (e.g., time series metric data) in real time. Multiple machine learning and other analytical techniques are applied in both instances to facilitate analysis.
Data storage will most often be supported by a combination of nonrelational data stores (such as MongoDB and other NoSQL databases) and highly distributed data processing and file management systems (such as Hadoop). Data collection is primarily performed via machine data forwarding and/or import (logs, documentation), data streaming (events, metrics, etc.) or API integrations from other tools that are collecting and/or generating data through their normal operations.
Examples of data sources analyzed by AIOps platforms include:
- Data natively generated by IT infrastructure and applications (e.g., streams, logs, packets, flows, etc.)
- • Data generated by tools used in the course of application development and DevOps initiatives (e.g., build/continuous integration [CI] tools, source code management, issue/bug tracking, testing, etc.)
- Data collected or generated by ITOM tools (e.g., agents or other instrumentation, discovery mechanisms, automation artifacts, configuration states, documentation or other knowledge items, service desk interactions and requests, etc.)
- Data collected or generated by identity and access management tools, line-of-business applications, social media and collaboration platforms, sentiment analysis mechanisms, and the Internet of Things
- Syndicated content from public and private external (third party) knowledge providers (e.g., government and nonprofit associations, consumer applications, commercial data providers)
AIOps platforms’ extensibility and ideally loose coupling of the data source, collection, storage, analysis, and presentation layers help avoid vendor lock-in and retain the ability to add new capabilities as they emerge. AIOps platforms’ datasource-agnostic approach also lends itself to being used in a uniquely flexible fashion, supplementing and enhancing other ITOM tool investments while minimizing their lock-in potential. While AIOps platforms can be substantially composed of open-source software components, it is expected that the majority of enterprises will either assemble or acquire solutions that incorporate both open-source and commercial software. Many of the most significant big data technologies in use today either have their roots in open source (Elasticsearch, Hadoop, Cassandra, Spark and others) or have since been contributed to the open-source community. This trend is expected to continue, such that enterprises should expect open-source technologies to play a critical part in AIOps platforms for the foreseeable future (five years or longer), enabling the platforms to take advantage of innovative technologies as they emerge. The location and delivery method (on-premises, SaaS or hybrid) of each layer and/or its component technologies can be considered independently; however, they should be considered in the context of a holistic AIOps platform strategy, as the complexity, performance and cost implications will vary significantly.
Benefits and Uses
AIOps platforms provide advanced analytical capabilities to multiple IT operations disciplines in both a direct and supplemental fashion. By doing so in a coordinated, centralized, yet flexible platform manner, they represent an opportunity to continuously deliver proactive insights informed by an automated, algorithmic learning capability analyzing an unprecedented breadth of data. Proactive insight delivered to IT operations specialists by AIOps platforms will generally take the forms of assisting human execution (making directed analysis easier, faster and or better) and augmenting human capabilities (using automated analysis to discover previously unseen insights). Providing insights in both forms allows AIOps platforms to support multiple skills levels and encourage adoption across a wide variety of use cases. It is common, for example, for subject matter experts to take advantage of assistance capabilities that help them get answers to diagnostic questions they know to ask based on experience. In contrast, it is common for operations generalists, architects and business professionals to gravitate toward the guidance that augmentation capabilities provide (see Table 1).
Deriving maximum value from AIOps platform capabilities will be achieved through the pervasive use of augmentation and assistance capabilities both directly, through applications built on the platform that can provide a holistic view across ITOM functions, and indirectly, through integration with tooling used within each ITOM function.
An example of an application built on an AIOps platform that spans multiple ITOM functions is an actionable, comprehensive feedback loop for a DevOps-delivered application to drive its continuous improvement. Some enterprise DevOps teams have done exactly this, building applications of this scope for a given application that include data from monitoring, automation, service desk and application development tools using AIOps platform tooling from Splunk, Sumo Logic, Elastic and others. Key to the decision to use an AIOps platform is that AIOps platforms uniquely provide more than just a method for gaining visibility into all the activities associated with an application’s creation, performance and evolution (using a variety of data sources, as noted in the Description section). Importantly, they also add the capability for both machines and people to learn from the behavior of the people and systems involved. These learning capabilities, informed by a broad perspective, are indeed useful when taken as a whole, but they also can provide significant value when leveraged within specific ITOM functions. The following are just a sample of use cases within major IT operations functions that illustrate both augmentation and assistance capabilities enabled by AIOps platforms.
Automation
Intelligently Adaptive (Heuristic) Automation — Augmentation: Automated workflows could be made “smarter” by having them take advantage of deterministic explicit knowledge, human tacit knowledge and AIOps-driven behavioral analysis, to deliver better outcomes in dynamic conditions.
Machine-Generated and Managed Automations — Augmentation: AIOps platforms could be used to identify patterns of positive behavior that could be automated, to codify that behavior in the form of automated tasks and workflows, to initiate those tasks and workflows given certain conditions, and to evolve those automated tasks and workflows based on outcomes.
Monitoring
Automated Behavior Prediction — Augmentation: The behavior of applications, infrastructure and users can be observed and analyzed on an ongoing basis to predict probable future events that may impact availability and performance.
Causal Analysis — Assistance and Augmentation: A combination of analytical approaches (Bayesian, Granger/temporal, etc.) can be applied to a broad set of data to suggest and compare multiple probable root causes of availability and performance issues.
Service Support
Intelligent Notification — Assistance and Augmentation: End users and IT operations personnel can be proactively notified across current or potential service impairments that will specifically impact them or need their specific attention. Intelligent Collaboration — Augmentation: Collaborative workspaces or communications streams can be enhanced with contextually relevant knowledge artifact (knowledge base/ FAQ articles, product documentation, support site links, etc.) recommendations or suggestions that dynamically adjust as the interaction progresses.
Business Value Dashboards
Business Opportunity Discovery — Augmentation: By analyzing both IT operational and business data, patterns of behavior yielding positive business outcomes could be detected.
Dynamic Decision Support — Assistance and Augmentation: Decision scenario design can be informed by AIOps platform recommendations based on real-time and historical analysis of both IT operational and business behavioral data.
AIOps platforms can also play important roles in IT security operations and business intelligence strategies, by providing ready access to the rich data and context generated in the course of IT operations.
To date, AIOps platform technologies have been most frequently adopted in support of availability and performance monitoring efforts. This is due to a number of factors, most notably the need of monitoring teams to rapidly perform often highly complex diagnostic tasks that AIOps technologies are ideally suited for. However, as IT operations tasks become increasingly automated, and roles and responsibilities continue to converge — with DevOps as a leading example — the work of analysis becomes a growing portion of all IT operations functions. This convergence in turn results in a growing need for AIOps platform capabilities that both AIOps-platform-focused and domain-centric (technology and discipline) vendors will continue to work to fulfill. Domain-centric vendors will continue to add AIOps platform technologies in various forms in a bid to become the dominant platform vendor, and current AIOpsplatform-focused vendors will continue to add capabilities that make them an increasingly viable alternative to domain-centric tooling.
Risks
The primary risk associated with investment in AIOps platforms mirrors that of most transformational efforts — an overemphasis on the technological component with insufficient focus on the changes in skills, roles, metrics and processes required to get value from the technology. Secondarily, platform investments are uniquely susceptible to both the effects of scope creep and “big bang” implementations that, at best, fail to meet unrealistic expectations and, at worst, negatively impact current operations. It remains critical that while the AIOps platform strategy should be comprehensive in its breadth, its implementation should be incremental.
There is a significant risk of confusing the value of AIOps platform augmentation and assistance with that of skills/people replacement, and that confusion in turn is being used to guide investment decisions. For the foreseeable future, the majority of value achieved leveraging AIOps platform capabilities will be realized by enhancing the capabilities of IT operations team personnel through augmentation and assistance, not by replacing them.
Alternatively, I&O leaders (and the enterprises they support) that do not invest in AIOps platforms run the risk of becoming irrelevant as their skills and tooling fail to keep up with exponentially growing operational complexity and the demand for proactive, personal and dynamic services. This growing irrelevance not only affects I&O leaders’ ability to compete for internal and external (outside the IT budget) funding, but can also put in jeopardy the enterprise’s ability to compete as a business.
Recommendations
Make a strategic investment in an AIOps platform initiative that will support major IT operations functions (monitoring, automation, service desk and more). The majority of enterprise investments in the technologies that can be used as part of an AIOps platform have been made in a tactical, fragmented fashion that significantly limits their potential value. To realize maximum value, enterprises should make a strategic and comprehensive investment in an AIOps platform initiative to be implemented in an incremental manner. I&O leaders should keep in mind, however, that while an AIOps platform includes all the capabilities described in the logical architecture diagram in Figure 2, the initial use cases, the technologies and vendors utilized, and the order in which those capabilities are implemented will vary from organization to organization.
Balance ease of use with interchangeability of platform capabilities (data collection, storage, analytical engines, presentation, etc.) to avoid lock-in. Many AIOps platform technologies and their interactions can be quite complex to implement and use. For example, some big data systems can require significant effort to size, scope and administer properly to achieve expected performance. Some machine learning techniques can require significant model building and training to achieve the expected results. Several vendors have responded to this challenge by coupling and/or consolidating various functional layers of AIOps platforms in the name of simplicity (such as XpoLog, Moogsoft, BigPanda, Rocana, Splunk, Sumo Logic and others). The drawback to this coupling is that it provides opportunities for vendors to create technical dependencies on that vendors’ products. It is important to be aware that lock-in can be designed at all functional layers of the AIOps platform, and it is the buyer’s responsibility to ensure that this risk is planned for.
Invest in building the skills and making the organizational changes needed to get value from an AIOps platform. AIOps platforms are often composed of bleeding-edge, leading-edge and established technologies that each bring respective skills requirements, particularly that of data science, which is often in short supply on IT operations teams. Most enterprise IT operations teams will have to significantly invest in building and acquiring the skills needed to take advantage of AIOps platforms. Skills sourcing plans should look to assemble and/or build data science, statistical, machine learning, operations modeling and mathematical skills, in addition to experience using advanced analytics tools. As part of a strategic, comprehensive AIOps investment plan, these skills investments need to be enabled by organizational changes that result in a team of AIOps specialists. Without this level of change, AIOps platform initiatives will likely fail to deliver expected results.
Representative Providers
Providers offering both machine learning and big data capabilities in one AIOps platform product: Hewlett Packard Enterprise (HPE), Rocana, Sumo Logic, XpoLog Providers offering one or more AIOps platform capabilities: BigPanda, BMC, Elastic, Evolven, ExtraHop, Graylog, IBM, Moogsoft, Prelert, Splunk, VMware Additional research contribution and review: Will Cappelli, Vivek Bhalla, Ian Head
Evidence
Additional data for this research was drawn from approximately 200 client inquiries over the past six months.