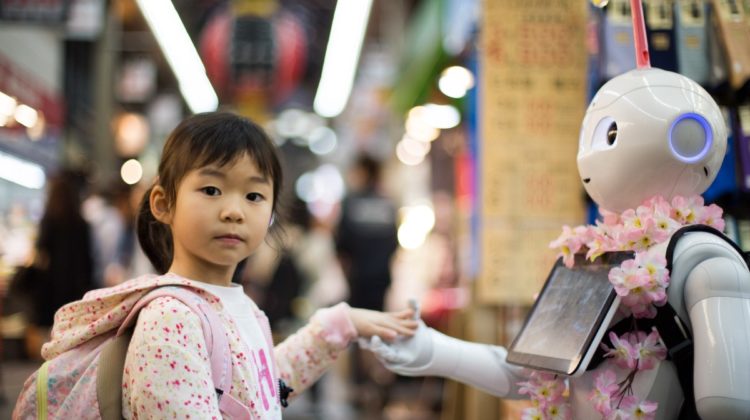
Judgment has been the bedrock of decision-making ever since human beings started trading with each other. Today judgment is one of the primary attributes that differentiate a good manager from the not so good one. Even in team sports, it’s not the best player but, usually, the player with the best judgment who is in charge of the reins of the team as a captain.
Few other advances have impacted business decision-making the way the application of Data Science has. Data Science has added objectivity to the judgment, which used to be primarily subjective and dependent on who the judge was. Its ability to crunch huge data and make sense of it is something beyond the capability of humans. Nevertheless, Data Science, in its avatar of Machine Learning and Artificial Intelligence, is still not a perfect answer to real-life business quests.
This post does not seek to critique or write off Data Science. Its objective is to highlight some of the challenges a business manager should be aware of before pinning all her hopes on DS & ML for making a forecast or a decision. I have listed down the challenges based on my own recent and limited experience and made use of real-life scenarios to make it easier for a layman to understand. In the next sections, let’s take a case for a manager trying to predict revenues of brick and mortar stores based on external and internal factors.
Data is the new oil, and it too doesn’t come easy: Any model is only as good as the data it feeds on. A typical real-life scenario needs reams of data to attribute sufficient explanation or meaningful interpretation. The data needs to have the right mix of quantity and quality to ensure that the model outcomes are balanced. With new technologies such as image processing, NLP, Internet-of-Things (IoT), and Big Data, etc, the collection of data has been revolutionized, however, there is still a lot of data that needs to be surveyed manually and also requires judgment by the surveyor. Moreover, not all data can be captured in a survey too. For instance, a store’s performance depends on the competitors around it. Therefore, to build a model to predict its performance, one needs to collect exhaustive data from competitors as well. The only plausible way is to conduct surveys of the competitors, but the process is usually cumbersome, expensive, and static.
The curse of the intangible: The model tries to explain everything with the data that has gone into it as input. In real life, it is seldom possible to collect all the data that needs to be fed in. Despite having everything in place, a store may be highly underperforming due to a previous scandal that might have brought a bad reputation to it. Even attributes such as staff morale and operational efficiency in the backend are difficult to judge and rate objectively as an input parameter. There can be a stark difference in a model’s prediction and ground reality in cases where intangible factors have a significant impact.
The Algorithm Black Box: Managers often feel uncomfortable with the idea of not being able to explain the reasoning behind a certain outcome or observation. With Data Science, they eventually have to become comfortable with it. While there are ways to validate the model’s outcome, it is difficult to even the most seasoned data scientists to explain why a certain independent variable is not making the kind of impact that a manager had expected based on a real-life example.
Changes in dynamics: Machine Learning models work the best in steady-state scenarios with mature brands and businesses. When it comes to the entry of a new brand, disruption in business due to the advent of new technology, and changes in government regulations, etc., managers have to go back to their humane skills to make judgments based on hunch, wisdom, guesswork, or a mix of all. Let’s say a government relaxes norms to retail recreational cannabis. Owing to the lack of previous data on cannabis sales, the ML modeling may just offer you a straight face, while your resourceful manager may still find a way out with her wits.
In a nutshell, Data Science has been one of the biggest blessings to the management in the last few decades, but it still plays more of a supporting role, aiding managers but not really replacing them. Yet!
Have any more examples? Would love to hear from you in the comments.