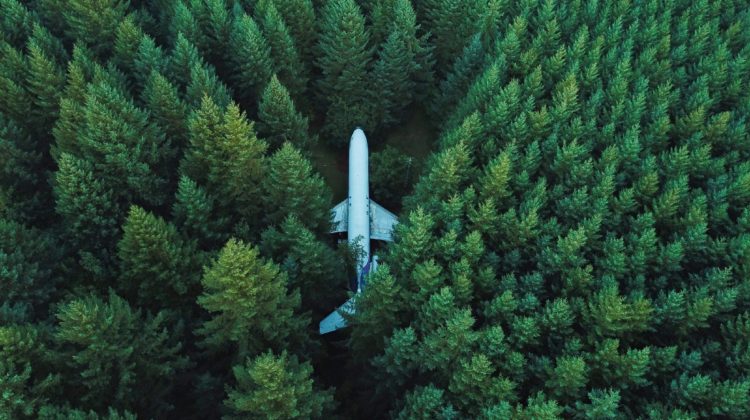
Alright, we know a random forest is made up of decision trees, and we have a rough idea what a decision tree is. How many trees are in the forest? The number of trees is determined by the user. Selecting the number of decision trees is important and often comes down to a simple cost-benefit equation: the cost of calculating more trees vs the possible benefit of increased performance.
To create multiple decision trees from the same training data, we apply bagging. Bagging is a common process in machine learning and is not limited to tree based methods. Essentially bagging is building multiple models, each based on a random sample of the training data. Pretty simple right?
To generate these samples, bootstrapping is used. To understand bootstrapping, imagine you have a toy data set:
import pandas as pd
import numpy as npnp.random.seed(0)
toy_data = pd.DataFrame({
'a' : np.random.choice(57, 10),
'c' : np.random.choice(11, 10),
'y' : np.random.choice(78, 10)
})display(toy_data)
To bootstrap this toy data, you first need to randomly select one row. The row selected is our first observation sampled from toy_data
. Next you sample another row, noting that the row you sampled first remains in the pool of possible rows to be selected. Repeat this process until you have the number of observations you would like. Now you have a bootstrapped sample!
When growing a random forest, the number of rows selected through bootstrapping will generally be equal to the number of rows in the training data. The number of bootstrapped samples you need is equal to the number of decision trees you need to grow.
As you can see, bootstrapping is simply sampling with replacement from the training data. The number of rows in each sample is the number of rows in the training data and the number of samples is the number of trees required for your forest. Easy.
Let’s see it in practice.
def bootstrap(df, random_state):
return df.sample(len(df), replace = True,
random_state = random_state)bootstrap(toy_data, 1)
You can see I’ve used pandas.DataFrame.sample with replacement = True
That’s all there is to it.
So we have a number of decision trees, grown based on bootstrapped samples of our training data. Do we have a random forest yet? Not quite. We need to address the random part of the random forest!
In each stage of growing a normal decision tree, all predictors are considered to determine the best next step in the tree.
In a random forest tree, a random sample of possible predictors is taken, before the each decision step is assessed.
This limits which predictors can be chosen for each step. Why is this important? Imagine three trees grown with this modified process, compared to three trees grown with the standard process. The three random forest trees will most likely be less similar to each other, because they have each been forced to consider a randomly selected set of predictors. Each random forest tree is more likely to consider predictors other trees have ignored.
Compare this with the standard process — these trees will probably be quite similar to each other. They have all considered the same set of predictors at each stage, the only difference is the bootstrapped sample they received as training input.
The result of the random forest tree process is reduced correlation among the trees.
A prediction in a random forest is simply a summary of the predictions from the the decision trees in the forest. The goal of summarising over many trees is to reduce variance right? Summarising over a set of less correlated trees will reduce variance even more. Take home?
A random forest will generally perform better on unseen test data than a single decision tree or a bagged set of decision trees.
Now that we have an understanding of the basic building blocks, We’re ready to tackle chapter two — growing a decision tree! Coming soon…:-)
If you found this insightful, helpful, or at all enjoyable, give some clappy hands to prop up my fragile ego…and to help others find it too!