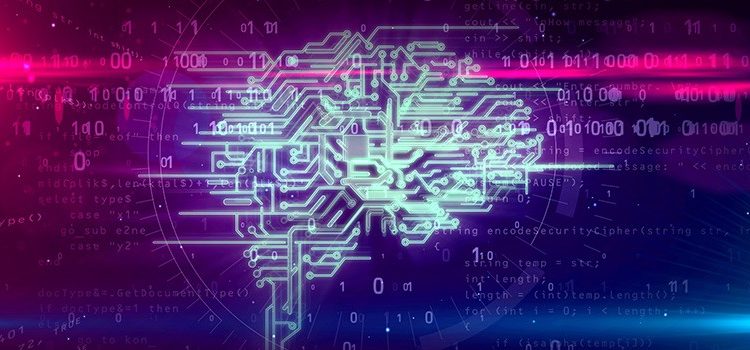

Location:
AI and ML initiatives are not solely conducted in the cloud nor are they handled on-premises. These initiatives should be executed in the location that makes the most sense given the output. For example, a facial recognition system at an airport should conduct the analysis locally, as the time taken to send the information to the cloud and back adds much latency to the process. It’s critical to ensure that infrastructure is deployed in the cloud, in the on-premises data center, and at the edge so the performance of AI initiatives is optimized.
To Know about: How is Robotic Technology Helping the Education Sector?
The breadth of high-performance infrastructure:
As mentioned earlier, AI performance is highly dependent on the underlying infrastructure. For example, graphical processing units (GPUs) can accelerate deep learning by 100 times compared to traditional central processing units (CPUs). Underpowering the server will cause delays in the process, while overpowering wastes money. Whether the strategy is end-to-end or best-of-breed, ensure the compute hardware has the right mix of processing capabilities and high-speed storage. This requires choosing a vendor that has a broad portfolio that can address any phase in the AI process.
Validated design:
Infrastructure is clearly important, but so is the software that runs on it. Once the software is installed, it can take several months to tune and optimize to fit the underlying hardware. Choose a vendor that has pre-installed the software and has a validated design in order to shorten the deployment time and ensure the performance is optimized.
Extension of the data center:
AI infrastructure does not live in isolation and should be considered an extension of the current data center. Ideally, businesses should look for a solution that can be managed with their existing tools.
End-to-end management:
There’s no single “AI in a box” that can be dropped in and turned on to begin the AI process. It’s composed of several moving parts, including servers, storage, networks, and software, with multiple choices at each position. The best solution would be a holistic one that includes all or at least most of the components that could be managed through a single interface.
Network infrastructure:
When deploying AI, an emphasis is put on GPU-enabled servers, flash storage, and other computer infrastructure. This makes sense, as AI is very processor and storage-intensive. However, the storage systems and servers must be fed data that traverses a network. Infrastructure for AI should be considered a “three-legged stool” where the legs are the network, servers, and storage. Each must be equally fast to keep up with each other. A lag in any one of these components can impair performance. The same level of due diligence given to servers and storage should be given to the network.
Security:
AI often involves extremely sensitive data such as patient records, financial information, and personal data. Having this data breached could be disastrous for the organization. Also, the infusion of bad data could cause the AI system to make incorrect inferences, leading to flawed decisions. The AI infrastructure must be secured from end to end with state-of-the-art technology.
Professional services:
Although services are not technically considered infrastructure, they should be part of the infrastructure decision. Most organizations, particularly inexperienced ones, won’t have the necessary skills in-house to make AI successful. A services partner can deliver the necessary training, advisory, implementation, and optimization services across the AI lifecycle and should be a core component of the deployment.
Broad ecosystem:
No single AI vendor can provide all technology everywhere. It’s crucial to use a vendor that has a broad ecosystem and can bring together all of the components of AI to deliver a full, turnkey, end-to-end solution. Having to cobble together the components will likely lead to delays and even failures. Choosing a vendor with a strong ecosystem provides a fast path to success.
Historically, AI and ML projects have been run by data science specialists, but that is quickly transitioning to IT professionals as these technologies move into the mainstream. As this transition happens and AI initiatives become more widespread, IT organizations should think more broadly about the infrastructure that enables AI. Instead of purchasing servers, network infrastructure, and other components for specific projects, the goal should be to think more broadly about the business’s needs both today and tomorrow, similar to the way data centers are run today.