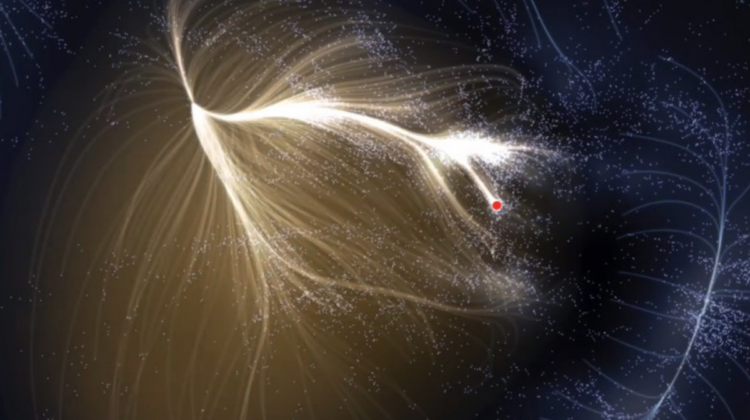
Something’s cooking in the world of physics. In 2006, the American theoretical physicist, Peter Woit, publishes a best-seller, Not Even Wrong. In his book, Woit argues, rather convincingly, that string theorists have gotten carried away. Without experimental evidence for their predictions, string theorists appear to live on a hunch: their math so articulated that their claims must be true. Woit’s book must have set things in motion. Others followed with similar findings. Then, in 2018, Sabine Hossenfelder publishes her best-seller with the hit-you-in-the-face title, Lost In Math, in which she takes on ‘the lot’ — the astute physicist, Eric Weinstein, later expresses appreciation for her courage. In a no-nonsense way, Hossenfelder shows the questionable rationale used by established physicists and reviewers when judging whether theories are right. The beauty of the math weighs too much, she argues. To paraphrase Hossenfelder, physicists are lured by mathematical construct, like we are lured by a building’s architecture, its originality and repetitive elements that make it unforgettable — the building collapsing, nonetheless.
Since that time, Hossenfelder, whom I follow on Twitter, has kind of served as keeper of sanity in the world of physics, giving an occasional nod but, more frequently, waking people up from non-sensical claims. Now and then, she retweets things of interest, such as, recently, an announcement of “mind-blowing interviews” with eight leading mathematicians and physicists on the question: “Is mathematics invented or discovered?” My instant gut response was: “INVENTED… [of course,] if you’d ask priests whether god exists, you know what to expect.” Curious about what these thinkers might actually believe, I took the time to listen through the interviews.
I kept track of the answers in a table with two columns, one for those who believe mathematics is invented and one for those who think it is an “independent” phenomenon, which defines reality and, thus, needs to be discovered. Soon, I had to add a third column for those who believed it could be either — as I gathered it, they didn’t really know and reasoned their way through it. Of the eight people interviewed, a majority of four ended up in the third column. I was stunned by their common reference to the Greek philosopher, Plato, when they reasoned that math or, at least, part of it must be discovered. Plato taught that physical reality was some kind of a reflection of fundamental forms or solids, such as the cube and tetrahedron. Accordingly, math shapes our reality as independent phenomenon just like Plato’s solids did. Frankly, I honestly believed that we had outgrown some of the insights of ancient Greek philosophers, however valuable they were, historically.
Of the remaining interviewees, two believed, at least as I perceived it, that math had to be discovered, independent as it was. Unsurprisingly, one was a string theorist, the other had proposed something as basic as strings.
I was most impressed, however, by the exceptionally well-articulated views of Stephen Wolfram and George Lakoff and not because they argued that math is invented. I knew about Wolfram’s “New Kind of Science” — I have his book, which is centered on the repeated computer-driven application of simple rules, so-called cellular automata. Refreshingly, Wolfram referred to the Babylonian civilization, which developed math by setting axioms, ‘operational principles’. To paraphrase Wolfram, certain math problems cannot be resolved because of the axioms involved. His objective, now, is to develop new axioms and cellular-automata-like tools that predict physical realities from the ground up, a new fundamental theory of physics, if you will. So, a new axiom, may lead to new math. There are, in other words, many ways that lead to Rome. George Lakoff, an American linguist and philosopher, was most explicit. As he pointed out, math is about metaphors, which we make up to describe our world. In fact, “a flower may fit a [mathematical] series but the series is not in the flower.”
Not long afterwards, an article appeared about the accurate prediction of planetary orbits by researchers at Princeton who used “Machine Learning” (ML), a form of “Artificial Intelligence” (AI). The lead researcher, Hong Qin, explains how they developed “a type of black box that can produce accurate predictions without using the traditional [math].” They first trained the ML model with the orbit data of various planets. Then, the model figured out by itself what the orbits of the other planets were. “There is no law of physics in the middle.”, Hong Qin points out. “I bypassed all the fundamental ingredients of physics [and went] directly from data to data.” AI, in other words, invented its own ‘math’ based on the data that Hong Qin and his colleagues fed it.
This is not a one-off success. I investigated a similar case, last year, involving a neural network that predicted the displacement of gravitational densities in the universe. It did so accurately and also much faster that traditional N-Body simulations. The latter predict the motion of individual ‘bodies’ — particles, gravitational densities, planets— by tediously calculating the effect of multiple ‘body-to-body’ interactions using the established laws of physics, an energy- and time-consuming process. Remarkably, the model was an adaptation of a deep-learning model developed to predict the spreading of cancer cells.
Crucially, the common denominator of both models is that their training data involved the displacement (or motion) of planets, gravitational densities, and cancer. In fact, gravitational-density predictions failed when the training data involved densities rather than their displacement.
To conclude, AI has found a way around the ‘spacetime empire’, a complicated ‘land of math and honing’, made up of spaces where certain rules apply. To traverse this empire, one usually travels through these spaces, labelled after founders, such as Euclid’s, Minkowski’s, Hilbert’s, Riemann’s, and Banach’s. That’s not counting the configuration and phase spaces that one is bound to run into. So, how did AI cut short its path to prediction — around it all?
To go by what Wolfram said, in order to explain what a ML black box does, one should search for the axiom that it spontaneously uses. In other words, what is the ‘operational principle’ that ML algorithms naturally converge on — their natural axiom or N-Axiom? Broadly speaking, ML algorithms are about data-pattern recognition. They sift through data to identify, at several levels (hence deep learning), any recurring patterns and the conditions, under which these appear. Once identified, the resulting, trained ML model can spit out recurring patterns for other conditions. Of course, as a Clubhouse-app room-session announcement on ‘AI attention mechanisms’, reminds me, increasingly clever ways are being developed to achieve this more efficiently. Then again, these ways don’t necessarily change the overall pattern-recognition objective.
So, what do ML algorithms actually ‘see’ when they identify recurrent data patterns (I prefer calling these patterns ‘data choreographies’ because they reflect repeated displacement or motion)? Not enlightened by what we presume when we observe our world from the ‘spacetime empire’ that gifted thinkers among us have crafted, ML algorithms simply meet the uncluttered outcome that the “process of nature” produced in the shape of raw data, that is, the examples they are trained with. In other words, ML basically reaches beyond and before the ‘land of math and honing’ to a realm of nature where the N-Axiom fosters the emergence of repeated displacement or motion.