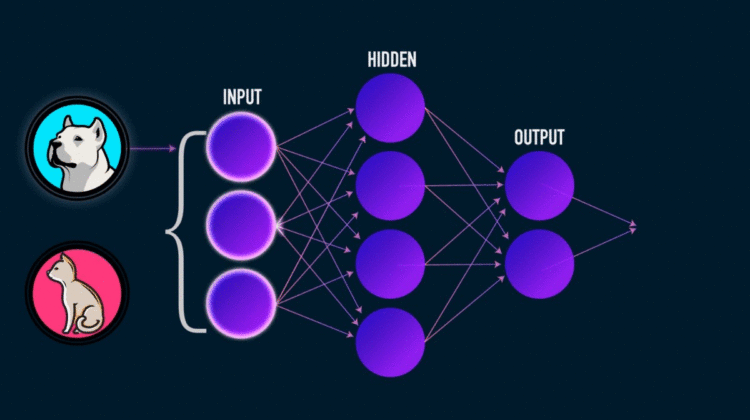
The above equation represents a sigmoid function. When we apply the weighted sum in the place of X, the values are scaled in between 0 and 1. The beauty of an exponent is that the value never reaches zero nor exceed 1 in the above equation. The large negative numbers are scaled towards 0 and large positive numbers are scaled towards 1.
In the above example, as x goes to minus infinity, y goes to 0 (tends not to fire).
As x goes to infinity, y goes to 1 (tends to fire):
At x=0, y=1/2.
The threshold is set to 0.5. If the value is above 0.5 it is scaled towards 1 and if it is below 0.5 it is scaled towards 0.
We can also change the sign to implement the opposite of the threshold by the above example. With a large positive input we get a large negative output which tends to not fire and with a large negative input we get a large positive output which tends to fire.
The beauty of sigmoid function is that the derivative of the function.
Once this is computed, it is easy to apply gradient descent during back propagation. It makes it smooth to gradually descent towards to minima once this is scaled while we apply the gradient descent. Here is a visual representation,
The Tanh function is an activation function which re scales the values between -1 and 1 by applying a threshold just like a sigmoid function. The advantage i.e the values of a tanh is zero centered which helps the next neuron during propagating.
Below is a tanh function
When we apply the weighted sum of the inputs in the tanh(x), it re scales the values between -1 and 1. . The large negative numbers are scaled towards -1 and large positive numbers are scaled towards 1.
In the above example, as x goes to minus infinity, tanh(x) goes to -1 (tends not to fire).
As x goes to infinity, tanh(x) goes to 1 (tends to fire):
At x=0, tanh(x)=0.
The thresold is set to 0. If the value is above 0 it is scaled towards 1 and if it is below 0 it is scaled towards -1.
This is implemented in the computation, just like the sigmoid it will smooth the curve where gradient descent will converge towards the minima based on the learning rate. Here is a visual of how it works,
This is one of the most widely used activation function. The benefits of ReLU is the sparsity, it allows only values which are positive and negative values are not passed which will speed up the process and it will negate or bring down possibility of occurrence of a dead neuron.
f(x) = (0,max)
This function will allow only the maximum values to pass during the front propagation as shown in the graph below. The draw backs of ReLU is when the gradient hits zero for the negative values, it does not converge towards the minima which will result in a dead neuron while back propagation.
This can be overcome by Leaky ReLU , which allows a small negative value during the back propagation if we have a dead ReLU problem. This will eventually activate the neuron and bring it down.
f(x)=1(x<0)(αx)+1(x>=0)(x) where α is a small constant
Some people have got results with this activation function but they are not always consistent. This activation function also has drawbacks, during the front propagation if the learning rate is set very high it will overshoot killing the neuron. This will happen when the learning rate is not set at an optimum level like in the below graph,
High learning rate leading to overshoot during gradient descent.
Low and optimal learning rate leading to a gradual descent towards the minima.
Softmax function is often described as a combination of multiple sigmoids. We know that sigmoid returns values between 0 and 1, which can be treated as probabilities of a data point belonging to a particular class. Thus sigmoid is widely used for binary classification problems.
The softmax function can be used for multiclass classification problems. This function returns the probability for a datapoint belonging to each individual class. Here is the mathematical expression of the same-
While building a network for a multiclass problem, the output layer would have as many neurons as the number of classes in the target. For instance if you have three classes, there would be three neurons in the output layer. Suppose you got the output from the neurons as [1.2 , 0.9 , 0.75].
Applying the softmax function over these values, you will get the following result — [0.42 , 0.31, 0.27]. These represent the probability for the data point belonging to each class. Note that the sum of all the values is 1. Let us code this in python
def softmax_function(x):
z = np.exp(x)
z_ = z/z.sum()
return z_softmax_function([0.8, 1.2, 3.1])
Output:
array([0.08021815, 0.11967141, 0.80011044])
Now that we have seen so many activation functions, we need some logic / heuristics to know which activation function should be used in which situation. Good or bad — there is no rule of thumb.
However depending upon the properties of the problem we might be able to make a better choice for easy and quicker convergence of the network.
- Sigmoid functions and their combinations generally work better in the case of classifiers
- Sigmoids and tanh functions are sometimes avoided due to the vanishing gradient problem
- ReLU function is a general activation function and is used in most cases these days
- If we encounter a case of dead neurons in our networks the leaky ReLU function is the best choice
- Always keep in mind that ReLU function should only be used in the hidden layers
- As a rule of thumb, you can begin with using ReLU function and then move over to other activation functions in case ReLU doesn’t provide with optimum results