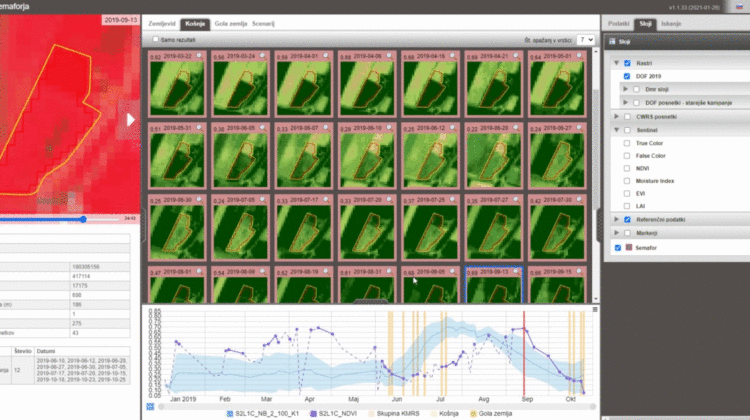
This is a multi-part series about machine learning and EO data supporting Common Agriculture Policy. Find information about related blog posts at the bottom.
In Area Monitoring (AM) having “better than 90% accuracy” ML results could still mean that 10% of the farmers might be wrongly penalized or that the Member State has to return the funds distributed inappropriately. Both outcomes result in millions of EURs of unnecessary cost and unhappy claimants.
The Expert Judgement Application (also known as the Expert App) is an integral part of AM, used by authorized operators to decide about parcels, where compliance (or non-compliance) cannot be determined automatically with an appropriate level of confidence— yellow parcels based on traffic lights (TL) nomenclature.
Besides categorisation of the yellow TL, the Expert-App is also used in the effective marker development procedure, for validation of automatically generated markers and, feeding the results back to the ML model for further improvements, hopefully resulting in more reliable red/green categorisations, in a positive reinforcing feedback loop.
As an example, expert validation is made by confirming or rejecting automatically derived crop group and/or land cover, or in case of event markers, by selecting image chips on which event can be recognized (as illustrated in the figure below).
The process also facilitates the identification of other clearly visible features, thereby further improving the reliability of the ML model used for the automatic assessment, as shown in the figure below.
However advanced the ML model is, it will struggle to provide a satisfactory degree of confidence for small or narrow parcels, or in areas with multiple cropping, partial abandonments and other mixed-signal responses. Thousands of parcels will require operators engagement to make a decision on whether the agricultural parcel is compliant with the CAP policy or not.
The workflow part of the Expert-App serves to distribute and organize the work, starting with the bulk creation of package bundles, each consisting of relevant (and, often, related) tasks to be performed, then assigned to the authorised users.
Package bundles are generated through the wizard – and after selecting the module type (e.g. TL assessment, marker validation, or clear-event identification), and desired inference (an application of the model on the data to produce a marker), authorised users may filter FOI’s based on available parameters (such as crop type, land use, area, etc.) or markers, in order to select priority packages of interest.
The expert app consists of several components, namely;
- Admin module, used for the creation of package bundles and package distribution among users and
- Main UI, where all available data is provided for expert’s interpretation (image chips, time-lapse, FOI’s attribute data, map with various layers such as aerial imagery and VHR satellite imagery, signal graph/profile, task list, and the settings panel for adapting the view).
It should be noted that visual components (time-lapse and signal graph/profiles) are standalone components provided as generic web services, which can be integrated into any other application.
Commissioning higher-resolution imagery
The main source of imagery is from Sentinel, whose excellent availability and revisit capability is suitable for most agricultural parcels, but where parcel size or shape (e.g. narrow elongated parcels only a few meters wide) become an issue, the Expert-App permits the integration of specially-commissioned data from various high-resolution satellite imagery (e.g. PlanetScope, SPOT, Pleiades) to assist in the decision making.
the Expert-App interface allows the user to order VHR imagery of higher spatial resolution from the relevant supplier services, defining desired source, date, and filtered on parameters such as cloud coverage. In a matter of minutes, users could make a validation by showing the results in the Expert App map.
ML-assisted rapid processing of large amounts of Earth Observation data will provide timely and effective area monitoring for many applications, and the Expert-App will provide additional support where there is still some ambiguity. As the system goes into production dealing with real-life cases, we are continuing to evolve and refine it.
Soon you may expect other posts related to the expert app in which we will dive deeper into the subject and present components in more detail, as well as show few real-life examples from Slovenia followed by explanations of how decision making is improved. Stay tuned!
This post is one of the series of blogs related to our work in Area Monitoring. We have decided to openly share our knowledge on this subject as we believe that discussion and comparison of approaches are required among all the groups involved in it. We would welcome any kind of feedback, ideas and lessons learned. For those willing to do it publicly, we are happy to host them at this place.
The content: