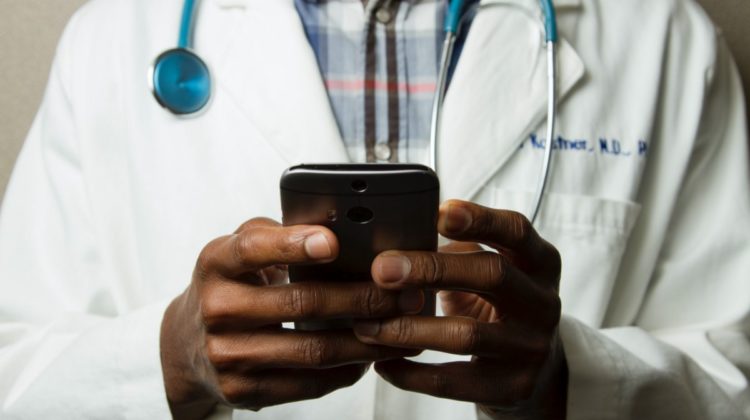
Early warning system for emerging diseases
Detecting the next major disease outbreak early is a priority for every nation. Machine learning models that can find patterns in traditional and alternative data sources might give us this early warning.
Covid-19 did not catch everyone flatfooted. BlueDot, an AI-powered health monitoring platform, notified its clients of an infectious disease outbreak in Wuhan on 31 December 2019, a full 9 days before the World Health Organization notified the public.
BlueDot applies machine learning and natural language processing to news reports in 65 languages, animal & plant disease data, and official releases to flag potential outbreaks. BlueDot even used airline ticketing data to correctly predict the initial spread from Wuhan to Bankgok, Seoul, Taipei and Tokyo.
Expect to see more of these AI-powered risk detection platforms in future. Corporations, investors, and governments are willing to pay. It will not be a surprise to see the likes of Google or Bloomberg provide similar services.
Remote diagnosis
The pandemic has strained hospital resources to near breaking point. Hospitals and clinics have every reason to embrace AI-enabled remote diagnosis tools to reduce pressure on doctors. Essentially, hospitals will rely on computers to diagnose medical conditions from MRIs, X-rays, CT scans, and even cellphone photos.
A deep learning model developed at MIT predicts breast cancer risk by analyzing a mammogram. What’s more, the model has a higher success rate than traditional screening methods and was trained and tested on 90,000 mammogram images.
Remote diagnosis applies to most conditions that can be diagnosed from medical images. More importantly, remote diagnosis tools can increase access to quality diagnosis in rural and low-income areas. Small rural testing centers can provide faster, cheaper diagnosis and collect data on emerging illnesses before it overwhelms small towns and villages.
Insights from clinical data
The U.S. healthcare system produces around 1.2 billion clinical documents per year. The majority of these are unstructured documents (e.g. handwritten doctors’ notes, images), with the rest being semi-structured (e.g. lab results, procedures, patient vitals).
There is too much data for physicians and nurses to sort through, even when they are in electronic format. Primary care physicians can spend up to 6 hours per day interacting with electronic health records. Research from the IBM Watson team found that physicians might miss around one important problem per patient record.
AI can help doctors and nurses extract key patient data and future medical risks to patients. Deep learning models paired with optical character recognition can digitize and label handwritten doctors’ notes and medical images for easy search and retrieval. Natural language processing and machine learning tools can generate patient summaries, highlight patient problems, and predict future medical risks such as diabetes or kidney disease.
It won’t be easy. Clinics and hospitals need ready and reliable data for AI tools to provide clinical decision support. Unfortunately, clinical data can be unorganized, siloed, or incomplete. Data consolidation, cleaning, and preprocessing will be necessary before AI tools influence medical decisions.
Accelerated drug discovery
Machine learning is reducing drug discovery costs and time to market by helping design chemical compounds with target properties.
Designing a new drug isn’t easy or cheap. A single drug may require an investment of $1 billion or more with only a 15% chance of approval. Drug discovery typically starts by analyzing up to 10,000 compounds. The entire process can exceed 10 years.
AI, specifically neural networks, can shorten the drug development timeline and reduce cost. A specific type of neural network, Generative Adversarial Networks (GAN), can be used to design chemical compounds with desirable characteristics for new drugs.
Google’s DeepMind developed a deep learning program called AlphaFold to determine the 3D shape of proteins. Predicting the structure of proteins gives insights into specific diseases. For example, researchers can understand how proteins interact. This could speed up drug development by showing researchers what properties a drug’s compound should have.
Using AI to accelerate drug discovery has massive implications for saving lives as well as increasing corporate profits. Still, many of these techniques are new and must be developed further. We won’t see labs shut down and scientists out of work anytime soon.
Improved hospital operations
A sobering lesson of the pandemic is that our hospitals can become overwhelmed. The early months of the pandemic saw hospitals in major cities run out of personal protective equipment (PPE; surgical masks, gowns), ventilators, and even hospital beds.
What if this could be avoided? AI-enabled trend analysis and prediction tools can flag emerging disease outbreaks, alerting hospitals to a need for beds. The BlueDot example above explores this. Faster illness detection using remote diagnosis can alert hospitals to stock up on PPE and staff. Inventory prediction models can use data on medical supplies usage to order supplies and prevent shortage.
Even after the pandemic, using AI to improve hospital operations will save money, reduce administrative burden, and improve patient care. One use case is optimizing hospital bed allocation using machine learning. Hospitals can streamline bed allocation by predicting when current patients will be discharged. This helps ensure bed capacity, allows hospitals to schedule surgeries with greater certainty, and reduces uncertainty and wait times for patients.
Improving hospital operations with AI is a clear win for everyone. Hospital resources are better utilized, enough staff are on hand, and fewer surgeries are cancelled for lack of beds. This improves both the patient experience and hospital revenues.
AI has the potential to drastically improve patient care, drug discovery, hospital operations, and disease tracking. Pharmaceutical firms, hospitals, and healthcare technology providers who adopt AI tools will boost both profits and market share.
Still, many of these technologies are new. There is still work required to ensure viability and safety. Adoption will also take time. Hospitals, clinics, and pharma companies should ensure adequate data quality and availability to use AI tools.
Healthcare providers and scientists will still be in demand when AI tools become widespread. AI tools might make diagnoses based on medical images, but doctors must still treat and reassure patients. Deep learning programs might speed up drug discovery, but scientists still have to double check the results. The cost of being wrong is high.
Ultimately, AI will speed up processing and generate insights, but this must be coupled with human judgement to improve patient outcomes. The future of healthcare is human plus AI, not just AI.