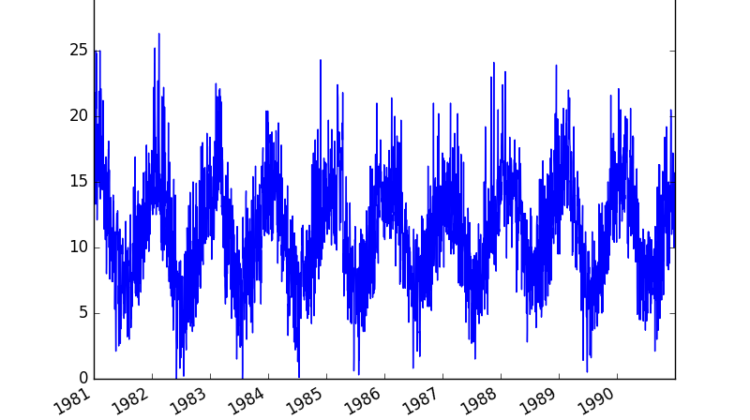

Autocorrelation and partial autocorrelation plots are heavily used in time series analysis and forecasting. These are plots that graphically summarize the strength of a relationship with an observation in a time series with observations at prior time steps. The difference between autocorrelation and partial autocorrelation can be difficult and confusing for beginners to time series forecasting.
This dataset describes the minimum daily temperatures over 10 years (1981–1990) in the city Melbourne, Australia.
The units are in degrees Celsius and there are 3,650 observations. The source of the data is credited as the Australian Bureau of Meteorology.
Download the dataset and place it in your current working directory with the filename “daily-minimum-temperatures.csv‘”.
Load the Minimum Daily Temperatures and graph the time series as follows:
from pandas import read_csvfrom matplotlib import pyplotseries = read_csv('daily-minimum-temperatures.csv', header=0, index_col=0)series.plot()pyplot.show()
Statistical correlation summarizes the strength of the relationship between two variables. We can assume the distribution of each variable fits a Gaussian (bell curve) distribution. If this is the case, we can use the Pearson’s correlation coefficient to summarize the correlation between the variables.
The Pearson’s correlation coefficient is a number between -1 and 1 that describes a negative or positive correlation respectively. A value of zero indicates no correlation.
We can calculate the correlation for time series observations with observations with previous time steps, called lags. Because the correlation of the time series observations is calculated with values of the same series at previous times, this is called a serial correlation, or an autocorrelation.
A plot of the autocorrelation of a time series by lag is called the AutoCorrelation Function, or the acronym ACF. This plot is sometimes called a correlogram or an autocorrelation plot.
Lets plot the autocorrelation plot for the Minimum Daily Temperatures using the plot_acf() function from the statsmodels library.
from pandas import read_csvfrom matplotlib import pyplotfrom statsmodels.graphics.tsaplots import plot_acfseries = read_csv('daily-minimum-temperatures.csv', header=0, index_col=0)plot_acf(series)pyplot.show()
This creates a 2D plot showing the lag value along the x-axis and the correlation on the y-axis between -1 and 1. Confidence intervals are drawn as a cone. By default, this is set to a 95% confidence interval.
By default, all lag values are printed, which makes the plot noisy. We can limit the number of lags on the x-axis to 50 to make the plot easier to read.
A partial autocorrelation is a summary of the relationship between an observation in a time series with observations at prior time steps with the relationships of intervening observations removed.
The partial autocorrelation at lag k is the correlation that results after removing the effect of any correlations due to the terms at shorter lags.
— Page 81, Section 4.5.6 Partial Autocorrelations, Introductory Time Series with R.
The autocorrelation for an observation and an observation at a prior time step is comprised of both the direct correlation and indirect correlations. These indirect correlations are a linear function of the correlation of the observation, with observations at intervening time steps. It is these indirect correlations that the partial autocorrelation function seeks to remove and forms the intuition for the partial autocorrelation.
Lets compute and plot the partial autocorrelation function for the first 50 lags in the Minimum Daily Temperatures dataset using the plot_pacf() from the statsmodels library.
from pandas import read_csvfrom matplotlib import pyplotfrom statsmodels.graphics.tsaplots import plot_pacfseries = read_csv('daily-minimum-temperatures.csv', header=0, index_col=0)plot_pacf(series, lags=50)pyplot.show()
This creates a 2D plot of the partial autocorrelation for the first 50 lags.