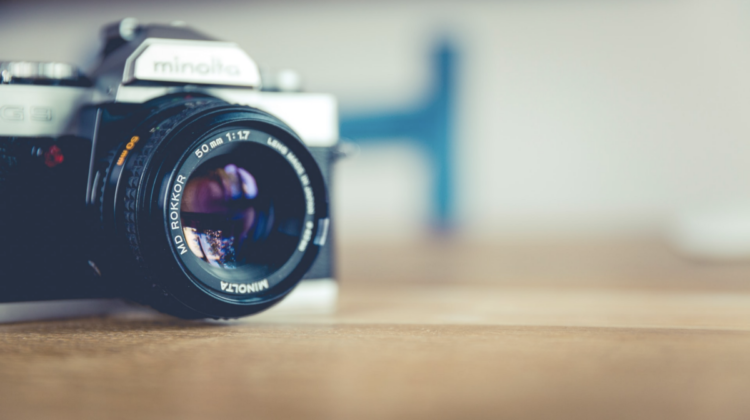

In previous article I walked through with you on how to build a real time object detection system using YOLOv5. In this article let’s build a web based text recognition system using Tesseract OCR, which is an open source optical character recognition engine for various operating systems.
I am going to use Tesseract version 4.1. The default installed language is English but you can install for other supported languages as well. The installation differs for each operating system and you can refer to the documentation.
- The web application uses camera to capture the photo and sends the image to the backend API using web socket.
- The API uses Tesseract to extract text from the image and sends the output back to the web application.
As you can see the quality of the image impacts the results. However Tesseract does a pretty decent job in extracting the information.
Front-End
The front-end is a simple React application which uses camera to capture photo. When the application starts, it connects to the backend using web socket.
The Backend
Backend is developed using FastAPI. There are 2 APIs provided
/ocr
allows you to upload an image for scanning’/ocr_ws
is the web socket endpoint.
You can test out the APIs by using the FastAPI provided endpoints through http://localhost:8088/docs
Both front-end and backend applications can be run using Docker. Just type make up
to start them using docker-compose.yml
For debugging Dockerized applications, you can check out the following articles in which I described the steps in details.
For production deployment, check out the article on YOLOv5 in which I explain why you must have valid certificates for the front-end and backend applications to serve secure traffic.
Using React and FastAPI, it is easy to host the text recognition APIs. There are definitely improvements which can be done to further improve the captured image quality and make it easier to extract text information from the image.
The source code for the application can be found at this repository.