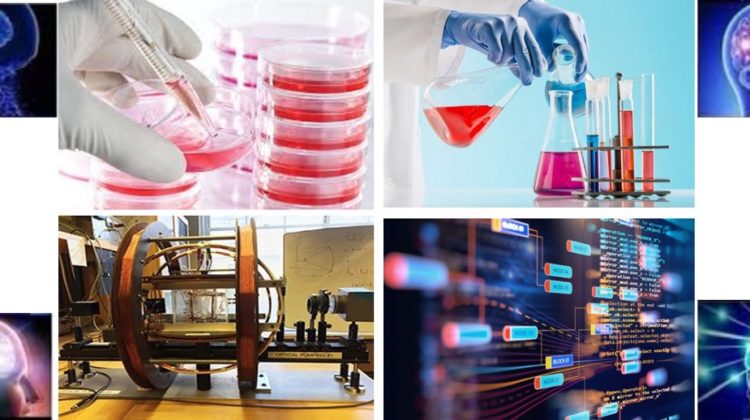

One of my old observation on people in academia in general is that they want to climb the ladder of theoretical complexity at the expense of real complexity. A person working in biology wants to show himself as a chemist, a chemist tries to project himself as physicist, a physicist assumes that he is a mathematician and mathematician don’t care about world. Surely I am not above this. Similar phenomena is driving academia towards the new buzz word of this decade, Artificial Intelligence, where they want to climb the ladder of computational complexity at the expense of theoretical complexity.
Whichever way you look at it, majority of scientific research articles by theoreticians fail to solve any real world problem. If this is an acceptable fact, it is getting worse because of the surge of articles where people are trying to exploit machine learning models to solve problems that are either too obvious or have no value. While climbing the ladder of theoretical complexity is fun and can be attributed to natural curiosity of peeping into unknown, climbing the ladder towards artificial intelligence can be dangerous because there is nothing to be known. Instead the real subject which requires attention is statistics. Every theorem and axiom using to build a machine learning method is already worked out in statistics. A good knowledge of basic statistical theorems will allow us to understand the structural patterns in the data beforehand. Machine learning is not a subject and should always be viewed as a mere tool. My advice to newbie’s who want to learn more about this new area is to take up course in statistics before getting tangled in the cobweb of artificial intelligence.