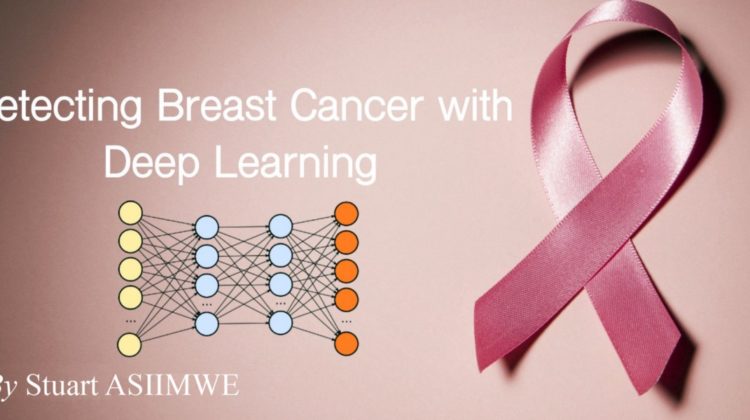

Each year, 182,000 women are diagnosed with breast cancer and 43,300 die. One woman in eight (1/8) either has or will develop breast cancer in her lifetime. If detected early, the five-year survival rate exceeds 95%. Mammography is the gold standard for detecting early signs of breast cancer, which can help cure the disease during its early stages. However, incorrect mammography diagnoses are common and may harm patients through unnecessary treatments and operations (or a lack of treatments). Therefore, systems that can learn to detect breast cancer on their own could help reduce the number of incorrect interpretations and missed cases which arise from failed responsibility of the physician to interpret breast cancer images to identify potential abnormalities, and their categorization with respect to growth. In this dissertation, design steps are presented for developing a new, reliable, and cost-effective diagnostic and prognostic tool for Breast Cancer using advanced Machine Learning (ML) techniques. The objective is to develop an online automated process that expertly catalogs suspicious regions, in the medical image hence supplementing and humanizing the physician’s ability to spot abnormalities. I present ‘DiagnoseCloud’, a Computer Aided Detection Web Application to suit the need of a CAD in breast cancer screening for cultivating image excellence and detecting abnormalities in a diversity of modalities associated with cancer screening. An 86.08% accuracy, ultimately was achieved on the mini-MIAS dataset, through transfer learning technique on pre-trained ImageNet weights of a VGG architecture applied on the dataset, to form the fully connected layers of the models Neural Network.