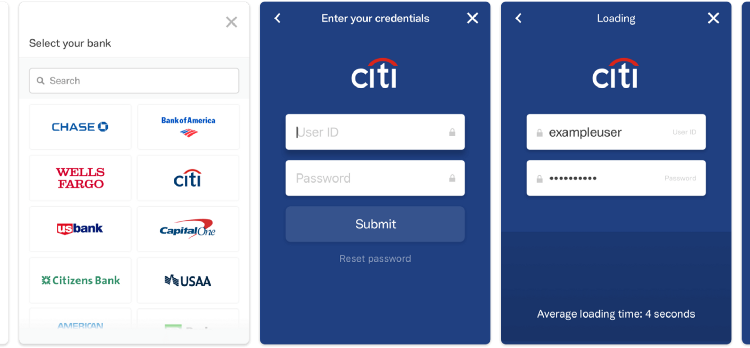

It is critical that both businesses and customers have confidence in the Reepei platform. One way to build confidence is to increase both parties’ understanding of how the platform works, specifically repayment. Let’s look into how the platform predicts this important number.
The context surrounding inability to pay
At Reepei, we believe that debts must be paid. But we also understand that life happens. There are many reasons people find themselves unable to make timely payments. One of the most common is lack of sufficient funds. Some customers have no available funds to pay what they owe while others have some funds, but choose to pay higher priority debts first. Overall, most customers want to pay, but simply cannot afford to do so. When they begin getting hounded by debt collection agencies, a friction occurs — customers do not have the funds necessary for repayment but repayment is the only way to satisfy collection agencies.
Reepei aims to eliminate this friction. We don’t want businesses or customers to worry about or be embarrassed by overdue debt. Repayment should be frictionless for all parties involved. And it can be.
How does it work?
Reepei uses Open Banking to access customers’ financial histories. The platform aggregates transactions for certain time periods, such as the previous three months. Based on this assessment, the platform predicts how much the customer can repay. But it also does much more.
In seconds, the platform reviews customer income and expenses and assesses how much cash will remain once expenses are paid. It uses machine learning (ML) to predict what customers with similar behaviour would do next. Here’s an example. Let’s look at a person who is refurbishing her bathroom; we will call her Julia.
Julia agreed to a refurbishment job that should total USD 3,000. The job took over a month to complete and Julia received an invoice. The plumbing company gave Julia 30 days to pay.
Part of the reason Julia commissioned the bathroom upgrade was that she had started to date someone new; we’ll call him John. The two planned to move in together and agreed to split the bathroom cost and pay USD 1,500 each. Julia had USD 2,000 in her savings account and felt that with John’s help, she could afford the upgrade.
As things became more serious with the couple, Julia began to buy John flowers every Friday. That was the day when the two met up for dinner, without fail, every week. Each week, the two took turns paying for the meal.
Then the unthinkable happened. John ghosted Julia. She never heard from him again. On top of that, Julia lost her job. She was left to foot the whole bathroom bill of USD 3,000. Because she had lost her job, she could not pay.
Luckily, the business that Julia owed used Reepei. After her invoice became overdue, the business sent Julia an invite to manage the debt using the platform. After Julia accepted the invitation, the platform aggregated data from her bank account. Multiple ML models produced predictions for Julia: the probability that she and John would break up as she bought him flowers; her dinner bills every other Friday; and the fact that she lost her job. Reepei uses this intelligence and its algorithm to predict how much Julia can repay now and in the future.
Let’s dig deeper into the details
The Reepei platform is built to be extremely efficient for both businesses and customers. Let’s look at Julia’s case to see how it works.
First, Julia approves providing Reepei access to her online banking accounts with a one-click confirmation. The platform aggregates all of Julia’s transactions over the last three months using Online Banking.
Next, the platform scores each transaction for a variety of factors. Typically, this includes the type of transaction (is it for broadband or flowers?) and how well the transaction matches what Julia will likely do next (will she have more or less funds coming in — like income, rent and gifts) and expenses.
Before the platform combines all the outcomes into a single score, it adds a few extra conditions. Many transactions are marked as irrelevant. This often includes transactions related to contracts like broadband internet and phone bills. What’s left are the transactions most relevant in predicting future repayment ability.
Any transaction that occurs rarely or over a limited period of time is prioritized in the prediction algorithm.
Finally, the platform combines the ML results with historical balances on Julia’s account and her current balance.
For Julia, her assessment looks like this:
– When the creditor invites Julia to use the Reepei platform, it adds the details of her USD 3,000 unpaid debt.
– Through Open Banking, Reepei obtains details about Julia’s USD 2,000 in her savings account. She spent a significant amount on flowers and eating out. She had a significant income. At a certain point, both her expenses and income stopped.
– The platform predicts that she will get a new job within six months.
– The platform predicts that she can spend USD 500 of her USD 2,000 in savings for her first instalment repayment.
– The platform predicts that she can spend USD 61 monthly to pay instalments for the next five months.
– The platform predicts that she can spend USD 363 each month after the first six months, when she would likely have a new job.
– Julia will have paid off the USD 3,000 debt after around 12 months.
All these steps happen seconds after Julia provides access to her bank account through Open Banking. At that point, Julia can relax. Repayment of her debt is now under control and she can rest assured that she can afford repayments.